Simultaneous prediction of binding free energy and specificity for PDZ domain-peptide interactions
- PMID: 24305904
- PMCID: PMC3909503
- DOI: 10.1007/s10822-013-9696-9
Simultaneous prediction of binding free energy and specificity for PDZ domain-peptide interactions
Abstract
Interactions between protein domains and linear peptides underlie many biological processes. Among these interactions, the recognition of C-terminal peptides by PDZ domains is one of the most ubiquitous. In this work, we present a mathematical model for PDZ domain-peptide interactions capable of predicting both affinity and specificity of binding based on X-ray crystal structures and comparative modeling with ROSETTA. We developed our mathematical model using a large phage display dataset describing binding specificity for a wild type PDZ domain and 91 single mutants, as well as binding affinity data for a wild type PDZ domain binding to 28 different peptides. Structural refinement was carried out through several ROSETTA protocols, the most accurate of which included flexible peptide docking and several iterations of side chain repacking and backbone minimization. Our findings emphasize the importance of backbone flexibility and the energetic contributions of side chain-side chain hydrogen bonds in accurately predicting interactions. We also determined that predicting PDZ domain-peptide interactions became increasingly challenging as the length of the peptide increased in the N-terminal direction. In the training dataset, predicted binding energies correlated with those derived through calorimetry and specificity switches introduced through single mutations at interface positions were recapitulated. In independent tests, our best performing protocol was capable of predicting dissociation constants well within one order of magnitude of the experimental values and specificity profiles at the level of accuracy of previous studies. To our knowledge, this approach represents the first integrated protocol for predicting both affinity and specificity for PDZ domain-peptide interactions.
Conflict of interest statement
Conflicts of interest: none
Figures
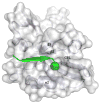
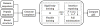
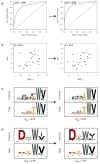
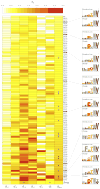
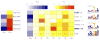
Similar articles
-
A physical model for PDZ-domain/peptide interactions.J Mol Model. 2011 Feb;17(2):315-24. doi: 10.1007/s00894-010-0725-5. Epub 2010 May 12. J Mol Model. 2011. PMID: 20461427 Free PMC article.
-
An in silico analysis of the binding modes and binding affinities of small molecule modulators of PDZ-peptide interactions.PLoS One. 2013 Aug 8;8(8):e71340. doi: 10.1371/journal.pone.0071340. eCollection 2013. PLoS One. 2013. PMID: 23951139 Free PMC article.
-
Hidden dynamic allostery in a PDZ domain.Proc Natl Acad Sci U S A. 2009 Oct 27;106(43):18249-54. doi: 10.1073/pnas.0904492106. Epub 2009 Oct 14. Proc Natl Acad Sci U S A. 2009. PMID: 19828436 Free PMC article.
-
The emerging contribution of sequence context to the specificity of protein interactions mediated by PDZ domains.FEBS Lett. 2012 Aug 14;586(17):2648-61. doi: 10.1016/j.febslet.2012.03.056. Epub 2012 Apr 4. FEBS Lett. 2012. PMID: 22709956 Review.
-
Seeking allosteric networks in PDZ domains.Protein Eng Des Sel. 2018 Oct 1;31(10):367-373. doi: 10.1093/protein/gzy033. Protein Eng Des Sel. 2018. PMID: 30690500 Free PMC article. Review.
Cited by
-
Computational design of selective peptides to discriminate between similar PDZ domains in an oncogenic pathway.J Mol Biol. 2015 Jan 30;427(2):491-510. doi: 10.1016/j.jmb.2014.10.014. Epub 2014 Oct 30. J Mol Biol. 2015. PMID: 25451599 Free PMC article.
References
-
- Pawson T, Nash P. Assembly of cell regulatory systems through protein interaction domains. Science. 2003;300:445. - PubMed
-
- Socolich M, Lockless SW, Russ WP, Lee H, Gardner KH, Ranganathan R. Evolutionary information for specifying a protein fold. Nature. 2005;437:512–518. - PubMed
-
- Russ WP, Lowery DM, Mishra P, Yaffe MB, Ranganathan R. Natural-like function in artificial WW domains. Nature. 2005;437:579–583. - PubMed
-
- Tonikian R, Xin X, Toret CP, Gfeller D, Landgraf C, Panni S, Paoluzi S, Castagnoli L, Currell B, Seshagiri S, Yu H, Winsor B, Vidal M, Gerstein MB, Bader GD, Volkmer R, Cesareni G, Drubin DG, Kim PM, Sidhu SS, Boone C. Bayesian modeling of the yeast SH3 domain interactome predicts spatiotemporal dynamics of endocytosis proteins. PLoS Biol. 2009;7:e1000218. - PMC - PubMed
Publication types
MeSH terms
Substances
Grants and funding
LinkOut - more resources
Full Text Sources
Other Literature Sources