Integrative pathway-based approach for genome-wide association studies: identification of new pathways for rheumatoid arthritis and type 1 diabetes
- PMID: 24205270
- PMCID: PMC3808349
- DOI: 10.1371/journal.pone.0078577
Integrative pathway-based approach for genome-wide association studies: identification of new pathways for rheumatoid arthritis and type 1 diabetes
Abstract
Genome-wide association studies (GWAS) led to the identification of numerous novel loci for a number of complex diseases. Pathway-based approaches using genotypic data provide tangible leads which cannot be identified by single marker approaches as implemented in GWAS. The available pathway analysis approaches mainly differ in the employed databases and in the applied statistics for determining the significance of the associated disease markers. So far, pathway-based approaches using GWAS data failed to consider the overlapping of genes among different pathways or the influence of protein-interactions. We performed a multistage integrative pathway (MIP) analysis on three common diseases--Crohn's disease (CD), rheumatoid arthritis (RA) and type 1 diabetes (T1D)--incorporating genotypic, pathway, protein- and domain-interaction data to identify novel associations between these diseases and pathways. Additionally, we assessed the sensitivity of our method by studying the influence of the most significant SNPs on the pathway analysis by removing those and comparing the corresponding pathway analysis results. Apart from confirming many previously published associations between pathways and RA, CD and T1D, our MIP approach was able to identify three new associations between disease phenotypes and pathways. This includes a relation between the influenza-A pathway and RA, as well as a relation between T1D and the phagosome and toxoplasmosis pathways. These results provide new leads to understand the molecular underpinnings of these diseases. The developed software herein used is available at http://www.cogsys.cs.uni-tuebingen.de/software/GWASPathwayIdentifier/index.htm.
Conflict of interest statement
Figures
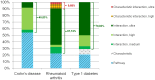
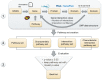
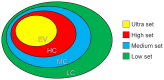
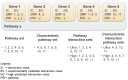
Similar articles
-
Integrated enrichment analysis of variants and pathways in genome-wide association studies indicates central role for IL-2 signaling genes in type 1 diabetes, and cytokine signaling genes in Crohn's disease.PLoS Genet. 2013;9(10):e1003770. doi: 10.1371/journal.pgen.1003770. Epub 2013 Oct 3. PLoS Genet. 2013. PMID: 24098138 Free PMC article.
-
Integrative analysis of genome-wide association studies and gene expression analysis identifies pathways associated with rheumatoid arthritis.Oncotarget. 2016 Feb 23;7(8):8580-9. doi: 10.18632/oncotarget.7390. Oncotarget. 2016. PMID: 26885899 Free PMC article.
-
Integrative analysis of genome-wide association study and expression quantitative trait loci datasets identified various immune cell-related pathways for rheumatoid arthritis.Ann Hum Genet. 2020 Jan;84(1):72-79. doi: 10.1111/ahg.12351. Epub 2019 Sep 4. Ann Hum Genet. 2020. PMID: 31486066
-
BridGE: a pathway-based analysis tool for detecting genetic interactions from GWAS.Nat Protoc. 2024 May;19(5):1400-1435. doi: 10.1038/s41596-024-00954-8. Epub 2024 Mar 21. Nat Protoc. 2024. PMID: 38514837 Free PMC article. Review.
-
A network analysis framework of genetic and nongenetic risks for type 2 diabetes.Rev Endocr Metab Disord. 2021 Jun;22(2):461-469. doi: 10.1007/s11154-020-09585-2. Epub 2020 Sep 14. Rev Endocr Metab Disord. 2021. PMID: 32926312 Review.
Cited by
-
Functional and genomic context in pathway analysis of GWAS data.Trends Genet. 2014 Sep;30(9):390-400. doi: 10.1016/j.tig.2014.07.004. Epub 2014 Aug 22. Trends Genet. 2014. PMID: 25154796 Free PMC article. Review.
-
Promising targets and drugs in rheumatoid arthritis: a module-based and cumulatively scoring approach.Bone Joint Res. 2020 Aug 2;9(8):501-514. doi: 10.1302/2046-3758.98.BJR-2019-0301.R1. eCollection 2020 Aug. Bone Joint Res. 2020. PMID: 32922758 Free PMC article.
-
Update on the genetic architecture of rheumatoid arthritis.Nat Rev Rheumatol. 2017 Jan;13(1):13-24. doi: 10.1038/nrrheum.2016.176. Epub 2016 Nov 4. Nat Rev Rheumatol. 2017. PMID: 27811914 Review.
-
Pathway-based association analysis of two genome-wide screening data identifies rheumatoid arthritis-related pathways.Genes Immun. 2014 Oct;15(7):487-94. doi: 10.1038/gene.2014.48. Epub 2014 Aug 7. Genes Immun. 2014. PMID: 25101796
-
Integrated genome-wide association, coexpression network, and expression single nucleotide polymorphism analysis identifies novel pathway in allergic rhinitis.BMC Med Genomics. 2014 Aug 2;7:48. doi: 10.1186/1755-8794-7-48. BMC Med Genomics. 2014. PMID: 25085501 Free PMC article.
References
Publication types
MeSH terms
Grants and funding
LinkOut - more resources
Full Text Sources
Other Literature Sources
Medical