Widespread purifying selection on RNA structure in mammals
- PMID: 23847102
- PMCID: PMC3783177
- DOI: 10.1093/nar/gkt596
Widespread purifying selection on RNA structure in mammals
Abstract
Evolutionarily conserved RNA secondary structures are a robust indicator of purifying selection and, consequently, molecular function. Evaluating their genome-wide occurrence through comparative genomics has consistently been plagued by high false-positive rates and divergent predictions. We present a novel benchmarking pipeline aimed at calibrating the precision of genome-wide scans for consensus RNA structure prediction. The benchmarking data obtained from two refined structure prediction algorithms, RNAz and SISSIz, were then analyzed to fine-tune the parameters of an optimized workflow for genomic sliding window screens. When applied to consistency-based multiple genome alignments of 35 mammals, our approach confidently identifies >4 million evolutionarily constrained RNA structures using a conservative sensitivity threshold that entails historically low false discovery rates for such analyses (5-22%). These predictions comprise 13.6% of the human genome, 88% of which fall outside any known sequence-constrained element, suggesting that a large proportion of the mammalian genome is functional. As an example, our findings identify both known and novel conserved RNA structure motifs in the long noncoding RNA MALAT1. This study provides an extensive set of functional transcriptomic annotations that will assist researchers in uncovering the precise mechanisms underlying the developmental ontologies of higher eukaryotes.
Figures
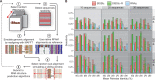
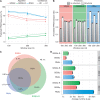
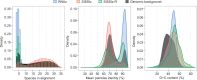
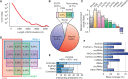
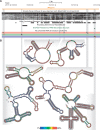
Similar articles
-
The RNAz web server: prediction of thermodynamically stable and evolutionarily conserved RNA structures.Nucleic Acids Res. 2007 Jul;35(Web Server issue):W335-8. doi: 10.1093/nar/gkm222. Epub 2007 Apr 22. Nucleic Acids Res. 2007. PMID: 17452347 Free PMC article.
-
Considerations in the identification of functional RNA structural elements in genomic alignments.BMC Bioinformatics. 2007 Jan 30;8:33. doi: 10.1186/1471-2105-8-33. BMC Bioinformatics. 2007. PMID: 17263882 Free PMC article.
-
Comparative RNA Genomics.Methods Mol Biol. 2018;1704:363-400. doi: 10.1007/978-1-4939-7463-4_14. Methods Mol Biol. 2018. PMID: 29277874
-
Evolutionary conservation of RNA sequence and structure.Wiley Interdiscip Rev RNA. 2021 Sep;12(5):e1649. doi: 10.1002/wrna.1649. Epub 2021 Mar 22. Wiley Interdiscip Rev RNA. 2021. PMID: 33754485 Free PMC article. Review.
-
Evolution to the rescue: using comparative genomics to understand long non-coding RNAs.Nat Rev Genet. 2016 Oct;17(10):601-14. doi: 10.1038/nrg.2016.85. Epub 2016 Aug 30. Nat Rev Genet. 2016. PMID: 27573374 Review.
Cited by
-
RNA sequencing describes both population structure and plasticity-selection dynamics in a non-model fish.BMC Genomics. 2021 Apr 15;22(1):273. doi: 10.1186/s12864-021-07592-4. BMC Genomics. 2021. PMID: 33858341 Free PMC article.
-
The interplay of long non-coding RNAs and MYC in cancer.AIMS Biophys. 2015;2(4):794-809. doi: 10.3934/biophy.2015.4.794. Epub 2015 Dec 1. AIMS Biophys. 2015. PMID: 27077133 Free PMC article.
-
Ancient exapted transposable elements promote nuclear enrichment of human long noncoding RNAs.Genome Res. 2019 Feb;29(2):208-222. doi: 10.1101/gr.229922.117. Epub 2018 Dec 26. Genome Res. 2019. PMID: 30587508 Free PMC article.
-
Probing the phenomics of noncoding RNA.Elife. 2013 Dec 31;2:e01968. doi: 10.7554/eLife.01968. Elife. 2013. PMID: 24381251 Free PMC article.
-
The function of miR-143, miR-145 and the MiR-143 host gene in cardiovascular development and disease.Vascul Pharmacol. 2019 Jan;112:24-30. doi: 10.1016/j.vph.2018.11.006. Epub 2018 Nov 29. Vascul Pharmacol. 2019. PMID: 30502421 Free PMC article. Review.
References
-
- Mattick JS. Introns: evolution and function. Curr. Opin. Genet. Dev. 1994;4:823–831. - PubMed
Publication types
MeSH terms
Substances
LinkOut - more resources
Full Text Sources
Other Literature Sources
Research Materials