Toward a "structural BLAST": using structural relationships to infer function
- PMID: 23349097
- PMCID: PMC3610042
- DOI: 10.1002/pro.2225
Toward a "structural BLAST": using structural relationships to infer function
Abstract
We outline a set of strategies to infer protein function from structure. The overall approach depends on extensive use of homology modeling, the exploitation of a wide range of global and local geometric relationships between protein structures and the use of machine learning techniques. The combination of modeling with broad searches of protein structure space defines a "structural BLAST" approach to infer function with high genomic coverage. Applications are described to the prediction of protein-protein and protein-ligand interactions. In the context of protein-protein interactions, our structure-based prediction algorithm, PrePPI, has comparable accuracy to high-throughput experiments. An essential feature of PrePPI involves the use of Bayesian methods to combine structure-derived information with non-structural evidence (e.g. co-expression) to assign a likelihood for each predicted interaction. This, combined with a structural BLAST approach significantly expands the range of applications of protein structure in the annotation of protein function, including systems level biological applications where it has previously played little role.
Copyright © 2013 The Protein Society.
Figures
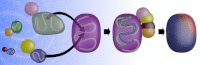

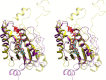
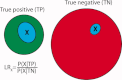
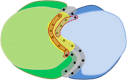
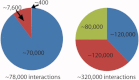
Similar articles
-
Template-based prediction of protein function.Curr Opin Struct Biol. 2015 Jun;32:33-8. doi: 10.1016/j.sbi.2015.01.007. Epub 2015 Feb 10. Curr Opin Struct Biol. 2015. PMID: 25678152 Free PMC article. Review.
-
Structure-based prediction of protein-protein interactions on a genome-wide scale.Nature. 2012 Oct 25;490(7421):556-60. doi: 10.1038/nature11503. Epub 2012 Sep 30. Nature. 2012. PMID: 23023127 Free PMC article.
-
Predicting peptide-mediated interactions on a genome-wide scale.PLoS Comput Biol. 2015 May 4;11(5):e1004248. doi: 10.1371/journal.pcbi.1004248. eCollection 2015 May. PLoS Comput Biol. 2015. PMID: 25938916 Free PMC article.
-
PrePPI: A Structure Informed Proteome-wide Database of Protein-Protein Interactions.J Mol Biol. 2023 Jul 15;435(14):168052. doi: 10.1016/j.jmb.2023.168052. Epub 2023 Mar 17. J Mol Biol. 2023. PMID: 36933822 Free PMC article.
-
Automatic annotation of protein function.Curr Opin Struct Biol. 2005 Jun;15(3):267-74. doi: 10.1016/j.sbi.2005.05.010. Curr Opin Struct Biol. 2005. PMID: 15922590 Review.
Cited by
-
Protein models docking benchmark 2.Proteins. 2015 May;83(5):891-7. doi: 10.1002/prot.24784. Epub 2015 Mar 25. Proteins. 2015. PMID: 25712716 Free PMC article.
-
Template-based prediction of protein function.Curr Opin Struct Biol. 2015 Jun;32:33-8. doi: 10.1016/j.sbi.2015.01.007. Epub 2015 Feb 10. Curr Opin Struct Biol. 2015. PMID: 25678152 Free PMC article. Review.
-
In Silico Methods for Identification of Potential Active Sites of Therapeutic Targets.Molecules. 2022 Oct 20;27(20):7103. doi: 10.3390/molecules27207103. Molecules. 2022. PMID: 36296697 Free PMC article. Review.
-
Artificial intelligence in the prediction of protein-ligand interactions: recent advances and future directions.Brief Bioinform. 2022 Jan 17;23(1):bbab476. doi: 10.1093/bib/bbab476. Brief Bioinform. 2022. PMID: 34849575 Free PMC article. Review.
-
Integrating 3D structural information into systems biology.J Biol Chem. 2021 Jan-Jun;296:100562. doi: 10.1016/j.jbc.2021.100562. Epub 2021 Mar 18. J Biol Chem. 2021. PMID: 33744294 Free PMC article. Review.
References
-
- Pearl F, Todd A, Sillitoe I, Dibley M, Redfern O, Lewis T, Bennett C, Marsden R, Grant A, Lee D, Akpor A, Maibaum M, Harrison A, Dallman T, Reeves G, Diboun I, Addou S, Lise S, Johnston C, Sillero A, Thornton J, Orengo C. The CATH Domain Structure Database and related resources Gene3D and DHS provide comprehensive domain family information for genome analysis. Nucleic Acids Res. 2005;33:D247–D251. - PMC - PubMed
-
- Kolodny R, Petrey D, Honig B. Protein structure comparison: implications for the nature of ‘fold space’, and structure and function prediction. Curr Opin Struct Biol. 2006;16:393–398. - PubMed
Publication types
MeSH terms
Substances
Grants and funding
LinkOut - more resources
Full Text Sources
Other Literature Sources
Research Materials