integIRTy: a method to identify genes altered in cancer by accounting for multiple mechanisms of regulation using item response theory
- PMID: 23014630
- PMCID: PMC3496341
- DOI: 10.1093/bioinformatics/bts561
integIRTy: a method to identify genes altered in cancer by accounting for multiple mechanisms of regulation using item response theory
Abstract
Motivation: Identifying genes altered in cancer plays a crucial role in both understanding the mechanism of carcinogenesis and developing novel therapeutics. It is known that there are various mechanisms of regulation that can lead to gene dysfunction, including copy number change, methylation, abnormal expression, mutation and so on. Nowadays, all these types of alterations can be simultaneously interrogated by different types of assays. Although many methods have been proposed to identify altered genes from a single assay, there is no method that can deal with multiple assays accounting for different alteration types systematically.
Results: In this article, we propose a novel method, integration using item response theory (integIRTy), to identify altered genes by using item response theory that allows integrated analysis of multiple high-throughput assays. When applied to a single assay, the proposed method is more robust and reliable than conventional methods such as Student's t-test or the Wilcoxon rank-sum test. When used to integrate multiple assays, integIRTy can identify novel-altered genes that cannot be found by looking at individual assay separately. We applied integIRTy to three public cancer datasets (ovarian carcinoma, breast cancer, glioblastoma) for cross-assay type integration which all show encouraging results.
Availability and implementation: The R package integIRTy is available at the web site http://bioinformatics.mdanderson.org/main/OOMPA:Overview.
Contact: kcoombes@mdanderson.org.
Supplementary information: Supplementary data are available at Bioinformatics online.
Figures
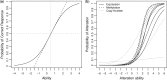
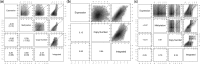
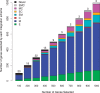
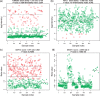
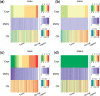
Similar articles
-
Joint analysis of expression profiles from multiple cancers improves the identification of microRNA-gene interactions.Bioinformatics. 2013 Sep 1;29(17):2137-45. doi: 10.1093/bioinformatics/btt341. Epub 2013 Jun 14. Bioinformatics. 2013. PMID: 23772050 Free PMC article.
-
SIBER: systematic identification of bimodally expressed genes using RNAseq data.Bioinformatics. 2013 Mar 1;29(5):605-13. doi: 10.1093/bioinformatics/bts713. Epub 2013 Jan 9. Bioinformatics. 2013. PMID: 23303507 Free PMC article.
-
DINGO: differential network analysis in genomics.Bioinformatics. 2015 Nov 1;31(21):3413-20. doi: 10.1093/bioinformatics/btv406. Epub 2015 Jul 6. Bioinformatics. 2015. PMID: 26148744 Free PMC article.
-
Inference of patient-specific pathway activities from multi-dimensional cancer genomics data using PARADIGM.Bioinformatics. 2010 Jun 15;26(12):i237-45. doi: 10.1093/bioinformatics/btq182. Bioinformatics. 2010. PMID: 20529912 Free PMC article.
-
CNAmet: an R package for integrating copy number, methylation and expression data.Bioinformatics. 2011 Mar 15;27(6):887-8. doi: 10.1093/bioinformatics/btr019. Epub 2011 Jan 12. Bioinformatics. 2011. PMID: 21228048
Cited by
-
OncoScape: Exploring the cancer aberration landscape by genomic data fusion.Sci Rep. 2016 Jun 20;6:28103. doi: 10.1038/srep28103. Sci Rep. 2016. PMID: 27321817 Free PMC article.
-
A Bayesian approach to joint analysis of multivariate longitudinal data and parametric accelerated failure time.Stat Med. 2014 Feb 20;33(4):580-94. doi: 10.1002/sim.5956. Epub 2013 Sep 6. Stat Med. 2014. PMID: 24009073 Free PMC article.
References
-
- Allison DB, et al. Microarray data analysis: from disarray to consolidation and consensus. Nat. Rev. Genet. 2006;7:55–65. - PubMed
-
- Andersen E. Discrete Statistical Models with Social Science Applications. 1980. North-Holland, Amsterdam.
-
- Andrich D. A rating formulation for ordered response categories. Psychometrika. 1978;43:561–573.
-
- Aoki M, et al. Expression of developmentally regulated endothelial cell locus 1 was induced by tumor-derived factors including VEGF. Biochem. Biophys. Res. Commun. 2005;333:990–995. - PubMed
Publication types
MeSH terms
Grants and funding
LinkOut - more resources
Full Text Sources
Medical