Inferring transcriptional and microRNA-mediated regulatory programs in glioblastoma
- PMID: 22929615
- PMCID: PMC3435504
- DOI: 10.1038/msb.2012.37
Inferring transcriptional and microRNA-mediated regulatory programs in glioblastoma
Abstract
Large-scale cancer genomics projects are profiling hundreds of tumors at multiple molecular layers, including copy number, mRNA and miRNA expression, but the mechanistic relationships between these layers are often excluded from computational models. We developed a supervised learning framework for integrating molecular profiles with regulatory sequence information to reveal regulatory programs in cancer, including miRNA-mediated regulation. We applied our approach to 320 glioblastoma profiles and identified key miRNAs and transcription factors as common or subtype-specific drivers of expression changes. We confirmed that predicted gene expression signatures for proneural subtype regulators were consistent with in vivo expression changes in a PDGF-driven mouse model. We tested two predicted proneural drivers, miR-124 and miR-132, both underexpressed in proneural tumors, by overexpression in neurospheres and observed a partial reversal of corresponding tumor expression changes. Computationally dissecting the role of miRNAs in cancer may ultimately lead to small RNA therapeutics tailored to subtype or individual.
Conflict of interest statement
The authors declare that they have no conflict of interest.
Figures
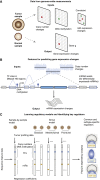
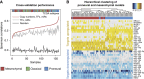
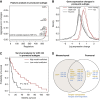
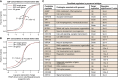
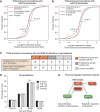
Similar articles
-
Genome-wide profiling identified a set of miRNAs that are differentially expressed in glioblastoma stem cells and normal neural stem cells.PLoS One. 2012;7(4):e36248. doi: 10.1371/journal.pone.0036248. Epub 2012 Apr 30. PLoS One. 2012. PMID: 22558405 Free PMC article.
-
MicroRNA expression and gene regulation drive breast cancer progression and metastasis in PyMT mice.Breast Cancer Res. 2016 Jul 22;18(1):75. doi: 10.1186/s13058-016-0735-z. Breast Cancer Res. 2016. PMID: 27449149 Free PMC article.
-
Integrated miRNA and mRNA expression profiling of mouse mammary tumor models identifies miRNA signatures associated with mammary tumor lineage.Genome Biol. 2011 Aug 16;12(8):R77. doi: 10.1186/gb-2011-12-8-r77. Genome Biol. 2011. PMID: 21846369 Free PMC article.
-
Targeting strategies on miRNA-21 and PDCD4 for glioblastoma.Arch Biochem Biophys. 2015 Aug 15;580:64-74. doi: 10.1016/j.abb.2015.07.001. Epub 2015 Jul 2. Arch Biochem Biophys. 2015. PMID: 26142886 Review.
-
Changes in miRNA expression in solid tumors: an miRNA profiling in melanomas.Semin Cancer Biol. 2008 Apr;18(2):111-22. doi: 10.1016/j.semcancer.2008.01.001. Epub 2008 Jan 15. Semin Cancer Biol. 2008. PMID: 18296065 Review.
Cited by
-
Reconstructing dynamic microRNA-regulated interaction networks.Proc Natl Acad Sci U S A. 2013 Sep 24;110(39):15686-91. doi: 10.1073/pnas.1303236110. Epub 2013 Aug 28. Proc Natl Acad Sci U S A. 2013. PMID: 23986498 Free PMC article.
-
Integrating multi-omics for uncovering the architecture of cross-talking pathways in breast cancer.PLoS One. 2014 Aug 19;9(8):e104282. doi: 10.1371/journal.pone.0104282. eCollection 2014. PLoS One. 2014. PMID: 25137136 Free PMC article.
-
Inferred miRNA activity identifies miRNA-mediated regulatory networks underlying multiple cancers.Bioinformatics. 2016 Jan 1;32(1):96-105. doi: 10.1093/bioinformatics/btv531. Epub 2015 Sep 10. Bioinformatics. 2016. PMID: 26358730 Free PMC article.
-
Inferring RBP-Mediated Regulation in Lung Squamous Cell Carcinoma.PLoS One. 2016 May 17;11(5):e0155354. doi: 10.1371/journal.pone.0155354. eCollection 2016. PLoS One. 2016. PMID: 27186987 Free PMC article.
-
Chromatin-informed inference of transcriptional programs in gynecologic and basal breast cancers.Nat Commun. 2019 Sep 25;10(1):4369. doi: 10.1038/s41467-019-12291-6. Nat Commun. 2019. PMID: 31554806 Free PMC article.
References
-
- Basso K, Margolin AA, Stolovitzky G, Klein U, Dalla-Favera R, Califano A (2005) Reverse engineering of regulatory networks in human B cells. Nat Genet 37: 382–390 - PubMed
Publication types
MeSH terms
Substances
Grants and funding
LinkOut - more resources
Full Text Sources
Other Literature Sources
Molecular Biology Databases