Genome-wide localization of protein-DNA binding and histone modification by a Bayesian change-point method with ChIP-seq data
- PMID: 22844240
- PMCID: PMC3406014
- DOI: 10.1371/journal.pcbi.1002613
Genome-wide localization of protein-DNA binding and histone modification by a Bayesian change-point method with ChIP-seq data
Abstract
Next-generation sequencing (NGS) technologies have matured considerably since their introduction and a focus has been placed on developing sophisticated analytical tools to deal with the amassing volumes of data. Chromatin immunoprecipitation sequencing (ChIP-seq), a major application of NGS, is a widely adopted technique for examining protein-DNA interactions and is commonly used to investigate epigenetic signatures of diffuse histone marks. These datasets have notoriously high variance and subtle levels of enrichment across large expanses, making them exceedingly difficult to define. Windows-based, heuristic models and finite-state hidden Markov models (HMMs) have been used with some success in analyzing ChIP-seq data but with lingering limitations. To improve the ability to detect broad regions of enrichment, we developed a stochastic Bayesian Change-Point (BCP) method, which addresses some of these unresolved issues. BCP makes use of recent advances in infinite-state HMMs by obtaining explicit formulas for posterior means of read densities. These posterior means can be used to categorize the genome into enriched and unenriched segments, as is customarily done, or examined for more detailed relationships since the underlying subpeaks are preserved rather than simplified into a binary classification. BCP performs a near exhaustive search of all possible change points between different posterior means at high-resolution to minimize the subjectivity of window sizes and is computationally efficient, due to a speed-up algorithm and the explicit formulas it employs. In the absence of a well-established "gold standard" for diffuse histone mark enrichment, we corroborated BCP's island detection accuracy and reproducibility using various forms of empirical evidence. We show that BCP is especially suited for analysis of diffuse histone ChIP-seq data but also effective in analyzing punctate transcription factor ChIP datasets, making it widely applicable for numerous experiment types.
Conflict of interest statement
The authors have declared that no competing interests exist.
Figures
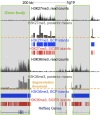
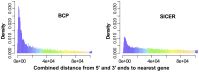
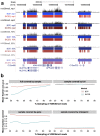
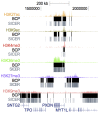


Similar articles
-
A novel Bayesian change-point algorithm for genome-wide analysis of diverse ChIPseq data types.J Vis Exp. 2012 Dec 10;(70):e4273. doi: 10.3791/4273. J Vis Exp. 2012. PMID: 23271069 Free PMC article.
-
Integrative analyses for omics data: a Bayesian mixture model to assess the concordance of ChIP-chip and ChIP-seq measurements.J Toxicol Environ Health A. 2012;75(8-10):461-70. doi: 10.1080/15287394.2012.674914. J Toxicol Environ Health A. 2012. PMID: 22686305
-
Genome-Wide Identification of Transcription Factor-Binding Sites in Quiescent Adult Neural Stem Cells.Methods Mol Biol. 2018;1686:265-286. doi: 10.1007/978-1-4939-7371-2_19. Methods Mol Biol. 2018. PMID: 29030827 Free PMC article.
-
Role of ChIP-seq in the discovery of transcription factor binding sites, differential gene regulation mechanism, epigenetic marks and beyond.Cell Cycle. 2014;13(18):2847-52. doi: 10.4161/15384101.2014.949201. Cell Cycle. 2014. PMID: 25486472 Free PMC article. Review.
-
ChIP-seq and beyond: new and improved methodologies to detect and characterize protein-DNA interactions.Nat Rev Genet. 2012 Dec;13(12):840-52. doi: 10.1038/nrg3306. Epub 2012 Oct 23. Nat Rev Genet. 2012. PMID: 23090257 Free PMC article. Review.
Cited by
-
Features that define the best ChIP-seq peak calling algorithms.Brief Bioinform. 2017 May 1;18(3):441-450. doi: 10.1093/bib/bbw035. Brief Bioinform. 2017. PMID: 27169896 Free PMC article.
-
Saturation analysis of ChIP-seq data for reproducible identification of binding peaks.Genome Res. 2015 Sep;25(9):1391-400. doi: 10.1101/gr.189894.115. Epub 2015 Jul 10. Genome Res. 2015. PMID: 26163319 Free PMC article.
-
Clustering-local-unique-enriched-signals (CLUES) promotes identification of novel regulators of ES cell self-renewal and pluripotency.PLoS One. 2018 Nov 6;13(11):e0206844. doi: 10.1371/journal.pone.0206844. eCollection 2018. PLoS One. 2018. PMID: 30399165 Free PMC article.
-
RNA polymerase II primes Polycomb-repressed developmental genes throughout terminal neuronal differentiation.Mol Syst Biol. 2017 Oct 16;13(10):946. doi: 10.15252/msb.20177754. Mol Syst Biol. 2017. PMID: 29038337 Free PMC article.
-
Brahma safeguards canalization of cardiac mesoderm differentiation.Nature. 2022 Feb;602(7895):129-134. doi: 10.1038/s41586-021-04336-y. Epub 2022 Jan 26. Nature. 2022. PMID: 35082446 Free PMC article.
References
-
- Ren B, Robert F, Wyrick JJ, Aparicio O, Jennings EG, et al. Genome-wide location and function of dna binding proteins. Science. 2000;290:2306–2309. - PubMed
-
- Johnson DS, Mortazavi A, Myers RM, Wold B. Genome-wide mapping of in vivo protein-dna interactions. Science. 2007;316:1497–1502. - PubMed
-
- Robertson, Hirst M, Bainbridge M, Bilenky M, Zhao Y, et al. Genome-wide profiles of stat1 dna association using chromatin immunoprecipitation and massively parallel sequencing. Nat Methods. 2007;4:651–657. - PubMed
-
- Barski, Cuddapah S, Cui K, Roh TY, Schones DE, et al. High-resolution profiling of histone methylations in the human genome. Cell. 2007;129:823–837. - PubMed
Publication types
MeSH terms
Substances
LinkOut - more resources
Full Text Sources