Risk estimation and risk prediction using machine-learning methods
- PMID: 22752090
- PMCID: PMC3432206
- DOI: 10.1007/s00439-012-1194-y
Risk estimation and risk prediction using machine-learning methods
Abstract
After an association between genetic variants and a phenotype has been established, further study goals comprise the classification of patients according to disease risk or the estimation of disease probability. To accomplish this, different statistical methods are required, and specifically machine-learning approaches may offer advantages over classical techniques. In this paper, we describe methods for the construction and evaluation of classification and probability estimation rules. We review the use of machine-learning approaches in this context and explain some of the machine-learning algorithms in detail. Finally, we illustrate the methodology through application to a genome-wide association analysis on rheumatoid arthritis.
Figures
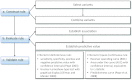
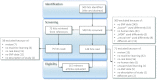
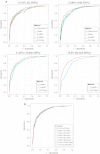
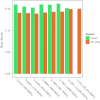
Similar articles
-
Probability machines: consistent probability estimation using nonparametric learning machines.Methods Inf Med. 2012;51(1):74-81. doi: 10.3414/ME00-01-0052. Epub 2011 Sep 14. Methods Inf Med. 2012. PMID: 21915433 Free PMC article.
-
Supervised machine learning and logistic regression identifies novel epistatic risk factors with PTPN22 for rheumatoid arthritis.Genes Immun. 2010 Apr;11(3):199-208. doi: 10.1038/gene.2009.110. Epub 2010 Jan 21. Genes Immun. 2010. PMID: 20090771 Free PMC article.
-
MegaSNPHunter: a learning approach to detect disease predisposition SNPs and high level interactions in genome wide association study.BMC Bioinformatics. 2009 Jan 9;10:13. doi: 10.1186/1471-2105-10-13. BMC Bioinformatics. 2009. PMID: 19134182 Free PMC article.
-
Bayesian Networks for Risk Prediction Using Real-World Data: A Tool for Precision Medicine.Value Health. 2019 Apr;22(4):439-445. doi: 10.1016/j.jval.2019.01.006. Epub 2019 Mar 15. Value Health. 2019. PMID: 30975395 Review.
-
Probability estimation with machine learning methods for dichotomous and multicategory outcome: theory.Biom J. 2014 Jul;56(4):534-63. doi: 10.1002/bimj.201300068. Epub 2014 Jan 29. Biom J. 2014. PMID: 24478134 Review.
Cited by
-
The usefulness of artificial intelligence in breast reconstruction: a systematic review.Breast Cancer. 2024 Jul;31(4):562-571. doi: 10.1007/s12282-024-01582-6. Epub 2024 Apr 15. Breast Cancer. 2024. PMID: 38619786 Review.
-
Genetic variants and their interactions in disease risk prediction - machine learning and network perspectives.BioData Min. 2013 Mar 1;6(1):5. doi: 10.1186/1756-0381-6-5. BioData Min. 2013. PMID: 23448398 Free PMC article.
-
Calibrating random forests for probability estimation.Stat Med. 2016 Sep 30;35(22):3949-60. doi: 10.1002/sim.6959. Epub 2016 Apr 13. Stat Med. 2016. PMID: 27074747 Free PMC article.
-
Early Prediction of Tacrolimus-Induced Tubular Toxicity in Pediatric Refractory Nephrotic Syndrome Using Machine Learning.Front Pharmacol. 2021 Aug 27;12:638724. doi: 10.3389/fphar.2021.638724. eCollection 2021. Front Pharmacol. 2021. PMID: 34512318 Free PMC article.
-
Pipeline design to identify key features and classify the chemotherapy response on lung cancer patients using large-scale genetic data.BMC Syst Biol. 2018 Nov 20;12(Suppl 5):97. doi: 10.1186/s12918-018-0615-5. BMC Syst Biol. 2018. PMID: 30458782 Free PMC article.
References
-
- Anderson J. Separate sample logistic discrimination. Biometrika. 1972;59:19–35. doi: 10.1093/biomet/59.1.19. - DOI
-
- Arminger G, Enache D. Statistical models and artificial neural networks. In: Bock H, Polasek W, editors. Data analysis and information systems. Heidelberg: Springer; 1996. pp. 243–260.
-
- Arshadi N, Chang B, Kustra R. Predictive modeling in case–control single-nucleotide polymorphism studies in the presence of population stratification: a case study using Genetic Analysis Workshop 16 Problem 1 dataset. BMC Proc. 2009;3(Suppl 7):S60. doi: 10.1186/1753-6561-3-s7-s60. - DOI - PMC - PubMed
-
- Banerjee M, Ding Y, Noone A (2012) Identifying representative trees from ensembles. Stat Med 31:1601–1616. doi:10.1002/sim.4492 4 - PubMed
Publication types
MeSH terms
Grants and funding
LinkOut - more resources
Full Text Sources
Other Literature Sources