The brain as a complex system: using network science as a tool for understanding the brain
- PMID: 22432419
- PMCID: PMC3621511
- DOI: 10.1089/brain.2011.0055
The brain as a complex system: using network science as a tool for understanding the brain
Abstract
Although graph theory has been around since the 18th century, the field of network science is more recent and continues to gain popularity, particularly in the field of neuroimaging. The field was propelled forward when Watts and Strogatz introduced their small-world network model, which described a network that provided regional specialization with efficient global information transfer. This model is appealing to the study of brain connectivity, as the brain can be viewed as a system with various interacting regions that produce complex behaviors. In practice, graph metrics such as clustering coefficient, path length, and efficiency measures are often used to characterize system properties. Centrality metrics such as degree, betweenness, closeness, and eigenvector centrality determine critical areas within the network. Community structure is also essential for understanding network organization and topology. Network science has led to a paradigm shift in the neuroscientific community, but it should be viewed as more than a simple "tool du jour." To fully appreciate the utility of network science, a greater understanding of how network models apply to the brain is needed. An integrated appraisal of multiple network analyses should be performed to better understand network structure rather than focusing on univariate comparisons to find significant group differences; indeed, such comparisons, popular with traditional functional magnetic resonance imaging analyses, are arguably no longer relevant with graph-theory based approaches. These methods necessitate a philosophical shift toward complexity science. In this context, when correctly applied and interpreted, network scientific methods have a chance to revolutionize the understanding of brain function.
Figures
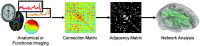
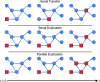
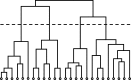
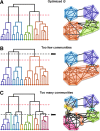
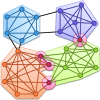
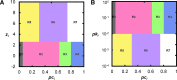
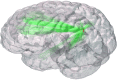
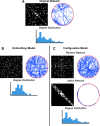
Similar articles
-
Evolving brain network dynamics in early childhood: Insights from modular graph metrics.Neuroimage. 2024 Aug 15;297:120740. doi: 10.1016/j.neuroimage.2024.120740. Epub 2024 Jul 23. Neuroimage. 2024. PMID: 39047590
-
Dyconnmap: Dynamic connectome mapping-A neuroimaging python module.Hum Brain Mapp. 2021 Oct 15;42(15):4909-4939. doi: 10.1002/hbm.25589. Epub 2021 Jul 11. Hum Brain Mapp. 2021. PMID: 34250674 Free PMC article.
-
Emerging Frontiers of Neuroengineering: A Network Science of Brain Connectivity.Annu Rev Biomed Eng. 2017 Jun 21;19:327-352. doi: 10.1146/annurev-bioeng-071516-044511. Epub 2017 Mar 27. Annu Rev Biomed Eng. 2017. PMID: 28375650 Free PMC article. Review.
-
Disconnection of network hubs and cognitive impairment after traumatic brain injury.Brain. 2015 Jun;138(Pt 6):1696-709. doi: 10.1093/brain/awv075. Epub 2015 Mar 25. Brain. 2015. PMID: 25808370 Free PMC article.
-
Small-World Brain Networks Revisited.Neuroscientist. 2017 Oct;23(5):499-516. doi: 10.1177/1073858416667720. Epub 2016 Sep 21. Neuroscientist. 2017. PMID: 27655008 Free PMC article. Review.
Cited by
-
Consistency of network modules in resting-state FMRI connectome data.PLoS One. 2012;7(8):e44428. doi: 10.1371/journal.pone.0044428. Epub 2012 Aug 31. PLoS One. 2012. PMID: 22952978 Free PMC article.
-
Method for persistent topological features extraction of schizophrenia patients' electroencephalography signal based on persistent homology.Front Comput Neurosci. 2022 Oct 5;16:1024205. doi: 10.3389/fncom.2022.1024205. eCollection 2022. Front Comput Neurosci. 2022. PMID: 36277610 Free PMC article.
-
Application of Graph Theory to Assess Static and Dynamic Brain Connectivity: Approaches for Building Brain Graphs.Proc IEEE Inst Electr Electron Eng. 2018 May;106(5):886-906. doi: 10.1109/JPROC.2018.2825200. Epub 2018 Apr 25. Proc IEEE Inst Electr Electron Eng. 2018. PMID: 30364630 Free PMC article.
-
Metanetwork Transmission Model for Predicting a Malaria-Control Strategy.Front Genet. 2018 Oct 17;9:446. doi: 10.3389/fgene.2018.00446. eCollection 2018. Front Genet. 2018. PMID: 30386373 Free PMC article.
-
Altered cortical structure network in children with obstructive sleep apnea.Sleep. 2022 May 12;45(5):zsac030. doi: 10.1093/sleep/zsac030. Epub 2022 Feb 5. Sleep. 2022. PMID: 35554588 Free PMC article.
References
Publication types
MeSH terms
LinkOut - more resources
Full Text Sources