Potential responders to FOLFOX therapy for colorectal cancer by Random Forests analysis
- PMID: 22095227
- PMCID: PMC3251854
- DOI: 10.1038/bjc.2011.505
Potential responders to FOLFOX therapy for colorectal cancer by Random Forests analysis
Abstract
Background: Molecular characterisation using gene-expression profiling will undoubtedly improve the prediction of treatment responses, and ultimately, the clinical outcome of cancer patients.
Methods: To establish the procedures to identify responders to FOLFOX therapy, 83 colorectal cancer (CRC) patients including 42 responders and 41 non-responders were divided into training (54 patients) and test (29 patients) sets. Using Random Forests (RF) algorithm in the training set, predictor genes for FOLFOX therapy were identified, which were applied to test samples and sensitivity, specificity, and out-of-bag classification accuracy were calculated.
Results: In the training set, 22 of 27 responders (81.4% sensitivity) and 23 of 27 non-responders (85.1% specificity) were correctly classified. To improve the prediction model, we removed the outliers determined by RF, and the model could correctly classify 21 of 23 responders (91.3%) and 22 of 23 non-responders (95.6%) in the training set, and 80.0% sensitivity and 92.8% specificity, with an accuracy of 69.2% in 29 independent test samples.
Conclusion: Random Forests on gene-expression data for CRC patients was effectively able to stratify responders to FOLFOX therapy with high accuracy, and use of pharmacogenomics in anticancer therapy is the first step in planning personalised therapy.
Figures
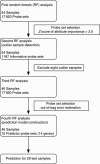
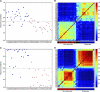
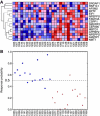
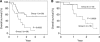
Similar articles
-
Gene expression signature and response to the use of leucovorin, fluorouracil and oxaliplatin in colorectal cancer patients.Clin Transl Oncol. 2011 Jun;13(6):419-25. doi: 10.1007/s12094-011-0676-z. Clin Transl Oncol. 2011. PMID: 21680303
-
FOLFOX treatment response prediction in metastatic or recurrent colorectal cancer patients via machine learning algorithms.Cancer Med. 2020 Feb;9(4):1419-1429. doi: 10.1002/cam4.2786. Epub 2020 Jan 1. Cancer Med. 2020. PMID: 31893575 Free PMC article.
-
Qualitative transcriptional signature for predicting pathological response of colorectal cancer to FOLFOX therapy.Cancer Sci. 2020 Jan;111(1):253-265. doi: 10.1111/cas.14263. Epub 2019 Dec 18. Cancer Sci. 2020. PMID: 31785020 Free PMC article.
-
Capecitabine plus oxaliplatin vs infusional 5-fluorouracil plus oxaliplatin in the treatment of colorectal cancer. Pro: The CapeOx regimen is preferred over FOLFOX.Clin Adv Hematol Oncol. 2005 May;3(5):400-4. Clin Adv Hematol Oncol. 2005. PMID: 16167013 Review. No abstract available.
-
FOLFOX versus FOLFIRI: a comparison of regimens in the treatment of colorectal cancer metastases.Anticancer Res. 2005 Jan-Feb;25(1B):563-76. Anticancer Res. 2005. PMID: 15816629 Review.
Cited by
-
Towards the Interpretability of Machine Learning Predictions for Medical Applications Targeting Personalised Therapies: A Cancer Case Survey.Int J Mol Sci. 2021 Apr 22;22(9):4394. doi: 10.3390/ijms22094394. Int J Mol Sci. 2021. PMID: 33922356 Free PMC article. Review.
-
Novel insights into the role of translesion synthesis polymerase in DNA incorporation and bypass of 5-fluorouracil in colorectal cancer.Nucleic Acids Res. 2024 May 8;52(8):4295-4312. doi: 10.1093/nar/gkae102. Nucleic Acids Res. 2024. PMID: 38416579 Free PMC article.
-
Machine learning for tissue diagnostics in oncology: brave new world.Br J Cancer. 2019 Sep;121(6):431-433. doi: 10.1038/s41416-019-0535-1. Epub 2019 Aug 9. Br J Cancer. 2019. PMID: 31395951 Free PMC article.
-
Big Data to Knowledge: Application of Machine Learning to Predictive Modeling of Therapeutic Response in Cancer.Curr Genomics. 2021 Dec 16;22(4):244-266. doi: 10.2174/1389202921999201224110101. Curr Genomics. 2021. PMID: 35273457 Free PMC article. Review.
-
Low expression of chloride channel accessory 1 predicts a poor prognosis in colorectal cancer.Cancer. 2015 May 15;121(10):1570-80. doi: 10.1002/cncr.29235. Epub 2015 Jan 20. Cancer. 2015. PMID: 25603912 Free PMC article.
References
-
- Affymetrix. Technical notes. Affymetrix I (2005) Guide to probe logarithmic intensity error (plier) estimation. http://www.affymetrix.com/support/technical/technotesmain.affx
-
- Berchuck A, Iversen ES, Lancaster JM, Pittman J, Luo J, Lee P, Murphy S, Dressman HK, Febbo PG, West M, Nevins JR, Marks JR (2005) Patterns of gene expression that characterize long-term survival in advanced stage serous ovarian cancers. Clin Cancer Res 11: 3686–3696 - PubMed
-
- Braun MS, Adab F, Bradley C, McAdam K, Thomas G, Wadd NJ, Rea D, Philips R, Twelves C, Bozzino J, MacMillan C, Saunders MP, Counsell R, Anderson H, McDonald A, Stewart J, Robinson A, Davies S, Richards FJ, Seymour MT (2003) Modified de Gramont with oxaliplatin in the first-line treatment of advanced colorectal cancer. Br J Cancer 89: 1155–1158 - PMC - PubMed
-
- Breiman L (2001) Random Forests. Mach Learn 45: 5–32
Publication types
MeSH terms
Substances
Supplementary concepts
LinkOut - more resources
Full Text Sources
Other Literature Sources
Medical
Molecular Biology Databases