DENSE: efficient and prior knowledge-driven discovery of phenotype-associated protein functional modules
- PMID: 22024446
- PMCID: PMC3231954
- DOI: 10.1186/1752-0509-5-172
DENSE: efficient and prior knowledge-driven discovery of phenotype-associated protein functional modules
Abstract
Background: Identifying cellular subsystems that are involved in the expression of a target phenotype has been a very active research area for the past several years. In this paper, cellular subsystem refers to a group of genes (or proteins) that interact and carry out a common function in the cell. Most studies identify genes associated with a phenotype on the basis of some statistical bias, others have extended these statistical methods to analyze functional modules and biological pathways for phenotype-relatedness. However, a biologist might often have a specific question in mind while performing such analysis and most of the resulting subsystems obtained by the existing methods might be largely irrelevant to the question in hand. Arguably, it would be valuable to incorporate biologist's knowledge about the phenotype into the algorithm. This way, it is anticipated that the resulting subsytems would not only be related to the target phenotype but also contain information that the biologist is likely to be interested in.
Results: In this paper we introduce a fast and theoretically guranteed method called DENSE (Dense and ENriched Subgraph Enumeration) that can take in as input a biologist's prior knowledge as a set of query proteins and identify all the dense functional modules in a biological network that contain some part of the query vertices. The density (in terms of the number of network egdes) and the enrichment (the number of query proteins in the resulting functional module) can be manipulated via two parameters γ and μ, respectively.
Conclusion: This algorithm has been applied to the protein functional association network of Clostridium acetobutylicum ATCC 824, a hydrogen producing, acid-tolerant organism. The algorithm was able to verify relationships known to exist in literature and also some previously unknown relationships including those with regulatory and signaling functions. Additionally, we were also able to hypothesize that some uncharacterized proteins are likely associated with the target phenotype. The DENSE code can be downloaded from http://www.freescience.org/cs/DENSE/
Figures
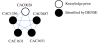
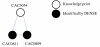
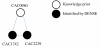
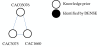
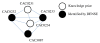
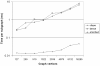
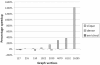
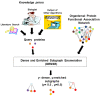
Similar articles
-
Complex receptor-ligand dynamics control the response of the VEGF system to protease injury.BMC Syst Biol. 2011 Oct 21;5:170. doi: 10.1186/1752-0509-5-170. BMC Syst Biol. 2011. PMID: 22014244 Free PMC article.
-
NIBBS-search for fast and accurate prediction of phenotype-biased metabolic systems.PLoS Comput Biol. 2012;8(5):e1002490. doi: 10.1371/journal.pcbi.1002490. Epub 2012 May 10. PLoS Comput Biol. 2012. PMID: 22589706 Free PMC article.
-
Modeling of growth factor-receptor systems from molecular-level protein interaction networks to whole-body compartment models.Methods Enzymol. 2009;467:461-497. doi: 10.1016/S0076-6879(09)67018-X. Methods Enzymol. 2009. PMID: 19897104 Free PMC article.
-
Neuropilin-1 enforces extracellular matrix signalling via ABL1 to promote angiogenesis.Biochem Soc Trans. 2014 Oct;42(5):1429-34. doi: 10.1042/BST20140141. Biochem Soc Trans. 2014. PMID: 25233427 Review.
-
Engineering Aspects of Olfaction.In: Persaud KC, Marco S, Gutiérrez-Gálvez A, editors. Neuromorphic Olfaction. Boca Raton (FL): CRC Press/Taylor & Francis; 2013. Chapter 1. In: Persaud KC, Marco S, Gutiérrez-Gálvez A, editors. Neuromorphic Olfaction. Boca Raton (FL): CRC Press/Taylor & Francis; 2013. Chapter 1. PMID: 26042329 Free Books & Documents. Review.
Cited by
-
Complex biomarker discovery in neuroimaging data: Finding a needle in a haystack.Neuroimage Clin. 2013 Aug 7;3:123-31. doi: 10.1016/j.nicl.2013.07.004. Neuroimage Clin. 2013. PMID: 24179856 Free PMC article. Review.
-
In-silico identification of phenotype-biased functional modules.Proteome Sci. 2012 Jun 21;10 Suppl 1(Suppl 1):S2. doi: 10.1186/1477-5956-10-S1-S2. Proteome Sci. 2012. PMID: 22759578 Free PMC article.
-
Quantitative assessment of gene expression network module-validation methods.Sci Rep. 2015 Oct 16;5:15258. doi: 10.1038/srep15258. Sci Rep. 2015. PMID: 26470848 Free PMC article.
-
SPICE: discovery of phenotype-determining component interplays.BMC Syst Biol. 2012 May 14;6:40. doi: 10.1186/1752-0509-6-40. BMC Syst Biol. 2012. PMID: 22583800 Free PMC article.
References
-
- Lopez-Gomollon S, Hernandez JA, Pellicer S, Angarica VE, Peleato ML, Fillat MF. Cross-talk between iron and nitrogen regulatory networks in Anabaena (Nostoc) sp. PCC 7120: Identification of overlapping genes in FurA and NtcA regulons. J Mol Biol. 2007;374:267–281. doi: 10.1016/j.jmb.2007.09.010. - DOI - PubMed
-
- Yebra MJ, Perez-Martinez G. Cross-talk between the L-sorbose and D-sorbitol (D-glucitol) metabolic pathways in Lactobacillus casei. Microbiology. 2002;148(8):2351–2359. - PubMed
Publication types
MeSH terms
Substances
LinkOut - more resources
Full Text Sources
Research Materials
Miscellaneous