Transcriptome sequencing across a prostate cancer cohort identifies PCAT-1, an unannotated lincRNA implicated in disease progression
- PMID: 21804560
- PMCID: PMC3152676
- DOI: 10.1038/nbt.1914
Transcriptome sequencing across a prostate cancer cohort identifies PCAT-1, an unannotated lincRNA implicated in disease progression
Abstract
Noncoding RNAs (ncRNAs) are emerging as key molecules in human cancer, with the potential to serve as novel markers of disease and to reveal uncharacterized aspects of tumor biology. Here we discover 121 unannotated prostate cancer-associated ncRNA transcripts (PCATs) by ab initio assembly of high-throughput sequencing of polyA(+) RNA (RNA-Seq) from a cohort of 102 prostate tissues and cells lines. We characterized one ncRNA, PCAT-1, as a prostate-specific regulator of cell proliferation and show that it is a target of the Polycomb Repressive Complex 2 (PRC2). We further found that patterns of PCAT-1 and PRC2 expression stratified patient tissues into molecular subtypes distinguished by expression signatures of PCAT-1-repressed target genes. Taken together, our findings suggest that PCAT-1 is a transcriptional repressor implicated in a subset of prostate cancer patients. These findings establish the utility of RNA-Seq to identify disease-associated ncRNAs that may improve the stratification of cancer subtypes.
Figures
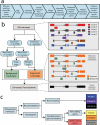
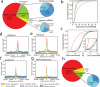
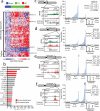
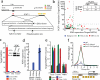
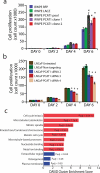
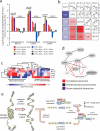
Similar articles
-
Tetraarsenic oxide affects non-coding RNA transcriptome through deregulating polycomb complexes in MCF7 cells.Adv Biol Regul. 2021 May;80:100809. doi: 10.1016/j.jbior.2021.100809. Epub 2021 Apr 14. Adv Biol Regul. 2021. PMID: 33932728
-
Expression changes in EZH2, but not in BMI-1, SIRT1, DNMT1 or DNMT3B are associated with DNA methylation changes in prostate cancer.Cancer Biol Ther. 2007 Sep;6(9):1403-12. doi: 10.4161/cbt.6.9.4542. Cancer Biol Ther. 2007. PMID: 18637271
-
Repression of E-cadherin by the polycomb group protein EZH2 in cancer.Oncogene. 2008 Dec 11;27(58):7274-84. doi: 10.1038/onc.2008.333. Epub 2008 Sep 22. Oncogene. 2008. PMID: 18806826 Free PMC article.
-
The EZH2 polycomb transcriptional repressor--a marker or mover of metastatic prostate cancer?Cancer Cell. 2002 Nov;2(5):349-50. doi: 10.1016/s1535-6108(02)00187-3. Cancer Cell. 2002. PMID: 12450788 Review.
-
Mutations and deletions of PRC2 in prostate cancer.Bioessays. 2016 May;38(5):446-54. doi: 10.1002/bies.201500162. Epub 2016 Mar 22. Bioessays. 2016. PMID: 27000413 Review.
Cited by
-
Integrative analysis reveals clinical phenotypes and oncogenic potentials of long non-coding RNAs across 15 cancer types.Oncotarget. 2016 Jun 7;7(23):35044-55. doi: 10.18632/oncotarget.9037. Oncotarget. 2016. PMID: 27147563 Free PMC article.
-
High expression of long non-coding RNA SBF2-AS1 promotes proliferation in non-small cell lung cancer.J Exp Clin Cancer Res. 2016 May 6;35:75. doi: 10.1186/s13046-016-0352-9. J Exp Clin Cancer Res. 2016. PMID: 27154193 Free PMC article.
-
The non-coding oncogene: a case of missing DNA evidence?Front Genet. 2012 Sep 12;3:170. doi: 10.3389/fgene.2012.00170. eCollection 2012. Front Genet. 2012. PMID: 22988449 Free PMC article. Review.
-
Regulating Methylation at H3K27: A Trick or Treat for Cancer Cell Plasticity.Cancers (Basel). 2020 Sep 29;12(10):2792. doi: 10.3390/cancers12102792. Cancers (Basel). 2020. PMID: 33003334 Free PMC article. Review.
-
The lncRNA landscape of breast cancer reveals a role for DSCAM-AS1 in breast cancer progression.Nat Commun. 2016 Sep 26;7:12791. doi: 10.1038/ncomms12791. Nat Commun. 2016. PMID: 27666543 Free PMC article.
References
Publication types
MeSH terms
Substances
Associated data
- Actions
- Actions
- Actions
Grants and funding
LinkOut - more resources
Full Text Sources
Other Literature Sources
Medical
Molecular Biology Databases