ModEnzA: Accurate Identification of Metabolic Enzymes Using Function Specific Profile HMMs with Optimised Discrimination Threshold and Modified Emission Probabilities
- PMID: 21541071
- PMCID: PMC3085309
- DOI: 10.1155/2011/743782
ModEnzA: Accurate Identification of Metabolic Enzymes Using Function Specific Profile HMMs with Optimised Discrimination Threshold and Modified Emission Probabilities
Abstract
Various enzyme identification protocols involving homology transfer by sequence-sequence or profile-sequence comparisons have been devised which utilise Swiss-Prot sequences associated with EC numbers as the training set. A profile HMM constructed for a particular EC number might select sequences which perform a different enzymatic function due to the presence of certain fold-specific residues which are conserved in enzymes sharing a common fold. We describe a protocol, ModEnzA (HMM-ModE Enzyme Annotation), which generates profile HMMs highly specific at a functional level as defined by the EC numbers by incorporating information from negative training sequences. We enrich the training dataset by mining sequences from the NCBI Non-Redundant database for increased sensitivity. We compare our method with other enzyme identification methods, both for assigning EC numbers to a genome as well as identifying protein sequences associated with an enzymatic activity. We report a sensitivity of 88% and specificity of 95% in identifying EC numbers and annotating enzymatic sequences from the E. coli genome which is higher than any other method. With the next-generation sequencing methods producing a huge amount of sequence data, the development and use of fully automated yet accurate protocols such as ModEnzA is warranted for rapid annotation of newly sequenced genomes and metagenomic sequences.
Figures
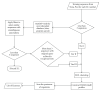
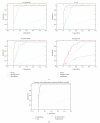
Similar articles
-
HMM-ModE: implementation, benchmarking and validation with HMMER3.BMC Res Notes. 2014 Jul 30;7:483. doi: 10.1186/1756-0500-7-483. BMC Res Notes. 2014. PMID: 25073805 Free PMC article.
-
HMM-ModE--improved classification using profile hidden Markov models by optimising the discrimination threshold and modifying emission probabilities with negative training sequences.BMC Bioinformatics. 2007 Mar 27;8:104. doi: 10.1186/1471-2105-8-104. BMC Bioinformatics. 2007. PMID: 17389042 Free PMC article.
-
Implementation of homology based and non-homology based computational methods for the identification and annotation of orphan enzymes: using Mycobacterium tuberculosis H37Rv as a case study.BMC Bioinformatics. 2020 Oct 19;21(1):466. doi: 10.1186/s12859-020-03794-x. BMC Bioinformatics. 2020. PMID: 33076816 Free PMC article.
-
[Analysis, identification and correction of some errors of model refseqs appeared in NCBI Human Gene Database by in silico cloning and experimental verification of novel human genes].Yi Chuan Xue Bao. 2004 May;31(5):431-43. Yi Chuan Xue Bao. 2004. PMID: 15478601 Chinese.
-
An Experimental Approach to Genome Annotation: This report is based on a colloquium sponsored by the American Academy of Microbiology held July 19-20, 2004, in Washington, DC.Washington (DC): American Society for Microbiology; 2004. Washington (DC): American Society for Microbiology; 2004. PMID: 33001599 Free Books & Documents. Review.
Cited by
-
Enzyme informatics.Curr Top Med Chem. 2012;12(17):1911-23. doi: 10.2174/156802612804547353. Curr Top Med Chem. 2012. PMID: 23116471 Free PMC article. Review.
-
HMM-ModE: implementation, benchmarking and validation with HMMER3.BMC Res Notes. 2014 Jul 30;7:483. doi: 10.1186/1756-0500-7-483. BMC Res Notes. 2014. PMID: 25073805 Free PMC article.
-
Accurately predicting enzyme functions through geometric graph learning on ESMFold-predicted structures.Nat Commun. 2024 Sep 18;15(1):8180. doi: 10.1038/s41467-024-52533-w. Nat Commun. 2024. PMID: 39294165 Free PMC article.
-
Giant hydrogen sulfide plume in the oxygen minimum zone off Peru supports chemolithoautotrophy.PLoS One. 2013 Aug 21;8(8):e68661. doi: 10.1371/journal.pone.0068661. eCollection 2013. PLoS One. 2013. PMID: 23990875 Free PMC article.
-
Evidential deep learning for trustworthy prediction of enzyme commission number.Brief Bioinform. 2023 Nov 22;25(1):bbad401. doi: 10.1093/bib/bbad401. Brief Bioinform. 2023. PMID: 37991247 Free PMC article.
References
-
- MacLean D, Jones JDG, Studholme DJ. Application of ’next-generation’ sequencing technologies to microbial genetics. Nature Reviews Microbiology. 2009;7(4):287–296. - PubMed
-
- Galperin MY, Koonin EV. Searching for drug targets in microbial genomes. Current Opinion in Biotechnology. 1999;10(6):571–578. - PubMed
-
- Hopkins AL, Groom CR. The druggable genome. Nature Reviews Drug Discovery. 2002;1(9):727–730. - PubMed
-
- Russ AP, Lampel S. The druggable genome: an update. Drug Discovery Today. 2005;10(23-24):1607–1610. - PubMed
LinkOut - more resources
Full Text Sources