Resolving the structure of interactomes with hierarchical agglomerative clustering
- PMID: 21342576
- PMCID: PMC3044301
- DOI: 10.1186/1471-2105-12-S1-S44
Resolving the structure of interactomes with hierarchical agglomerative clustering
Abstract
Background: Graphs provide a natural framework for visualizing and analyzing networks of many types, including biological networks. Network clustering is a valuable approach for summarizing the structure in large networks, for predicting unobserved interactions, and for predicting functional annotations. Many current clustering algorithms suffer from a common set of limitations: poor resolution of top-level clusters; over-splitting of bottom-level clusters; requirements to pre-define the number of clusters prior to analysis; and an inability to jointly cluster over multiple interaction types.
Results: A new algorithm, Hierarchical Agglomerative Clustering (HAC), is developed for fast clustering of heterogeneous interaction networks. This algorithm uses maximum likelihood to drive the inference of a hierarchical stochastic block model for network structure. Bayesian model selection provides a principled method for collapsing the fine-structure within the smallest groups, and for identifying the top-level groups within a network. Model scores are additive over independent interaction types, providing a direct route for simultaneous analysis of multiple interaction types. In addition to inferring network structure, this algorithm generates link predictions that with cross-validation provide a quantitative assessment of performance for real-world examples.
Conclusions: When applied to genome-scale data sets representing several organisms and interaction types, HAC provides the overall best performance in link prediction when compared with other clustering methods and with model-free graph diffusion kernels. Investigation of performance on genome-scale yeast protein interactions reveals roughly 100 top-level clusters, with a long-tailed distribution of cluster sizes. These are in turn partitioned into 1000 fine-level clusters containing 5 proteins on average, again with a long-tailed size distribution. Top-level clusters correspond to broad biological processes, whereas fine-level clusters correspond to discrete complexes. Surprisingly, link prediction based on joint clustering of physical and genetic interactions performs worse than predictions based on individual data sets, suggesting a lack of synergy in current high-throughput data.
Figures
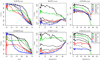
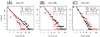
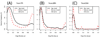
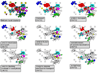
Similar articles
-
How networks change with time.Bioinformatics. 2012 Jun 15;28(12):i40-8. doi: 10.1093/bioinformatics/bts211. Bioinformatics. 2012. PMID: 22689777 Free PMC article.
-
The relative vertex clustering value--a new criterion for the fast discovery of functional modules in protein interaction networks.BMC Bioinformatics. 2015;16 Suppl 4(Suppl 4):S3. doi: 10.1186/1471-2105-16-S4-S3. Epub 2015 Feb 23. BMC Bioinformatics. 2015. PMID: 25734691 Free PMC article.
-
Markov clustering versus affinity propagation for the partitioning of protein interaction graphs.BMC Bioinformatics. 2009 Mar 30;10:99. doi: 10.1186/1471-2105-10-99. BMC Bioinformatics. 2009. PMID: 19331680 Free PMC article.
-
Review on statistical methods for gene network reconstruction using expression data.J Theor Biol. 2014 Dec 7;362:53-61. doi: 10.1016/j.jtbi.2014.03.040. Epub 2014 Apr 12. J Theor Biol. 2014. PMID: 24726980 Review.
-
Integrated inference and analysis of regulatory networks from multi-level measurements.Methods Cell Biol. 2012;110:19-56. doi: 10.1016/B978-0-12-388403-9.00002-3. Methods Cell Biol. 2012. PMID: 22482944 Free PMC article. Review.
Cited by
-
How networks change with time.Bioinformatics. 2012 Jun 15;28(12):i40-8. doi: 10.1093/bioinformatics/bts211. Bioinformatics. 2012. PMID: 22689777 Free PMC article.
-
Global airborne bacterial community-interactions with Earth's microbiomes and anthropogenic activities.Proc Natl Acad Sci U S A. 2022 Oct 18;119(42):e2204465119. doi: 10.1073/pnas.2204465119. Epub 2022 Oct 10. Proc Natl Acad Sci U S A. 2022. PMID: 36215495 Free PMC article.
-
A gene ontology inferred from molecular networks.Nat Biotechnol. 2013 Jan;31(1):38-45. doi: 10.1038/nbt.2463. Nat Biotechnol. 2013. PMID: 23242164 Free PMC article.
-
Functional network motifs defined through integration of protein-protein and genetic interactions.PeerJ. 2022 Feb 22;10:e13016. doi: 10.7717/peerj.13016. eCollection 2022. PeerJ. 2022. PMID: 35223214 Free PMC article.
-
Compact Integration of Multi-Network Topology for Functional Analysis of Genes.Cell Syst. 2016 Dec 21;3(6):540-548.e5. doi: 10.1016/j.cels.2016.10.017. Epub 2016 Nov 23. Cell Syst. 2016. PMID: 27889536 Free PMC article.
References
-
- Zachary WW. An Information Flow Model for Conflict and Fission in Small Groups. Journal of Anthropological Research. 1977;33(4):452–473.
-
- Yu H, Braun P, Yildirim MA, Lemmens I, Venkatesan K, Sahalie J, Hirozane-Kishikawa T, Gebreab F, Li N, Simonis N, Hao T, Rual JF, Dricot A, Vazquez A, Murray RR, Simon C, Tardivo L, Tam S, Svrzikapa N, Fan C, de Smet AS, Motyl A, Hudson ME, Park J, Xin X, Cusick ME, Moore T, Boone C, Snyder M, Roth FP, Barabasi AL, Tavernier J, Hill DE, Vidal M. High-Quality Binary Protein Interaction Map of the Yeast Interactome Network. Science. 2008;322(5898):104–110. doi: 10.1126/science.1158684. - DOI - PMC - PubMed
Publication types
MeSH terms
LinkOut - more resources
Full Text Sources
Molecular Biology Databases