A hybrid BPSO-CGA approach for gene selection and classification of microarray data
- PMID: 21210743
- PMCID: PMC3244808
- DOI: 10.1089/cmb.2010.0064
A hybrid BPSO-CGA approach for gene selection and classification of microarray data
Abstract
Microarray analysis promises to detect variations in gene expressions, and changes in the transcription rates of an entire genome in vivo. Microarray gene expression profiles indicate the relative abundance of mRNA corresponding to the genes. The selection of relevant genes from microarray data poses a formidable challenge to researchers due to the high-dimensionality of features, multiclass categories being involved, and the usually small sample size. A classification process is often employed which decreases the dimensionality of the microarray data. In order to correctly analyze microarray data, the goal is to find an optimal subset of features (genes) which adequately represents the original set of features. A hybrid method of binary particle swarm optimization (BPSO) and a combat genetic algorithm (CGA) is to perform the microarray data selection. The K-nearest neighbor (K-NN) method with leave-one-out cross-validation (LOOCV) served as a classifier. The proposed BPSO-CGA approach is compared to ten microarray data sets from the literature. The experimental results indicate that the proposed method not only effectively reduce the number of genes expression level, but also achieves a low classification error rate.
Figures
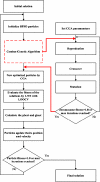
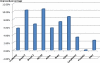
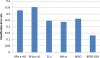
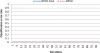
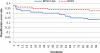
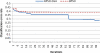
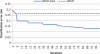
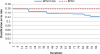
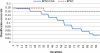
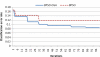
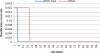
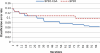
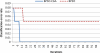
Similar articles
-
Chaotic genetic algorithm for gene selection and classification problems.OMICS. 2009 Oct;13(5):407-20. doi: 10.1089/omi.2009.0007. OMICS. 2009. PMID: 19594377
-
Tabu search and binary particle swarm optimization for feature selection using microarray data.J Comput Biol. 2009 Dec;16(12):1689-703. doi: 10.1089/cmb.2007.0211. J Comput Biol. 2009. PMID: 20047491
-
Correlation-based gene selection and classification using Taguchi-BPSO.Methods Inf Med. 2010;49(3):254-68. doi: 10.3414/ME09-01-0010. Epub 2010 Feb 5. Methods Inf Med. 2010. PMID: 20135079
-
Filter versus wrapper gene selection approaches in DNA microarray domains.Artif Intell Med. 2004 Jun;31(2):91-103. doi: 10.1016/j.artmed.2004.01.007. Artif Intell Med. 2004. PMID: 15219288 Review.
-
Gene set enrichment analysis: performance evaluation and usage guidelines.Brief Bioinform. 2012 May;13(3):281-91. doi: 10.1093/bib/bbr049. Epub 2011 Sep 7. Brief Bioinform. 2012. PMID: 21900207 Free PMC article. Review.
Cited by
-
Feature Subset Selection for Cancer Classification Using Weight Local Modularity.Sci Rep. 2016 Oct 5;6:34759. doi: 10.1038/srep34759. Sci Rep. 2016. PMID: 27703256 Free PMC article.
-
Gene selection for microarray data classification via subspace learning and manifold regularization.Med Biol Eng Comput. 2018 Jul;56(7):1271-1284. doi: 10.1007/s11517-017-1751-6. Epub 2017 Dec 19. Med Biol Eng Comput. 2018. PMID: 29256006
-
Sulfatase 2 Is Associated with Steroid Resistance in Childhood Nephrotic Syndrome.J Clin Med. 2021 Feb 2;10(3):523. doi: 10.3390/jcm10030523. J Clin Med. 2021. PMID: 33540508 Free PMC article.
-
Identification of SNP barcode biomarkers for genes associated with facial emotion perception using particle swarm optimization algorithm.Ann Gen Psychiatry. 2014 May 21;13:15. doi: 10.1186/1744-859X-13-15. eCollection 2014. Ann Gen Psychiatry. 2014. PMID: 24955105 Free PMC article.
-
Multi-class BCGA-ELM based classifier that identifies biomarkers associated with hallmarks of cancer.BMC Bioinformatics. 2015 May 20;16:166. doi: 10.1186/s12859-015-0565-5. BMC Bioinformatics. 2015. PMID: 25986937 Free PMC article.
References
-
- AltIncay H. Ensembling evidential k-nearest neighbor classifiers through multi-modal perturbation. Applied Soft Computing. 2007;7:1072–1083.
-
- Breiman L. Classification and Regression Trees. Chapman & Hall/CRC; Boca Raton, FL: 1984.
-
- Buturovic L.J. PCP: a program for supervised classification of gene expression profiles. Bioinformatics. 2006;22:245. - PubMed
Publication types
MeSH terms
LinkOut - more resources
Full Text Sources
Research Materials