Using diffusion distances for flexible molecular shape comparison
- PMID: 20868474
- PMCID: PMC2949899
- DOI: 10.1186/1471-2105-11-480
Using diffusion distances for flexible molecular shape comparison
Abstract
Background: Many molecules are flexible and undergo significant shape deformation as part of their function, and yet most existing molecular shape comparison (MSC) methods treat them as rigid bodies, which may lead to incorrect shape recognition.
Results: In this paper, we present a new shape descriptor, named Diffusion Distance Shape Descriptor (DDSD), for comparing 3D shapes of flexible molecules. The diffusion distance in our work is considered as an average length of paths connecting two landmark points on the molecular shape in a sense of inner distances. The diffusion distance is robust to flexible shape deformation, in particular to topological changes, and it reflects well the molecular structure and deformation without explicit decomposition. Our DDSD is stored as a histogram which is a probability distribution of diffusion distances between all sample point pairs on the molecular surface. Finally, the problem of flexible MSC is reduced to comparison of DDSD histograms.
Conclusions: We illustrate that DDSD is insensitive to shape deformation of flexible molecules and more effective at capturing molecular structures than traditional shape descriptors. The presented algorithm is robust and does not require any prior knowledge of the flexible regions.
Figures
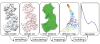
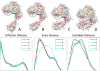
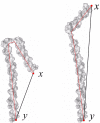
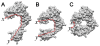
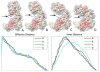
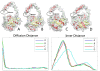
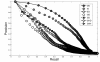
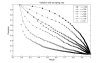
Similar articles
-
3DMolNavi: a web-based retrieval and navigation tool for flexible molecular shape comparison.BMC Bioinformatics. 2012 May 14;13:95. doi: 10.1186/1471-2105-13-95. BMC Bioinformatics. 2012. PMID: 22583488 Free PMC article.
-
IDSS: deformation invariant signatures for molecular shape comparison.BMC Bioinformatics. 2009 May 22;10:157. doi: 10.1186/1471-2105-10-157. BMC Bioinformatics. 2009. PMID: 19463181 Free PMC article.
-
Three dimensional shape comparison of flexible proteins using the local-diameter descriptor.BMC Struct Biol. 2009 May 12;9:29. doi: 10.1186/1472-6807-9-29. BMC Struct Biol. 2009. PMID: 19435524 Free PMC article.
-
Shape classification using the inner-distance.IEEE Trans Pattern Anal Mach Intell. 2007 Feb;29(2):286-99. doi: 10.1109/TPAMI.2007.41. IEEE Trans Pattern Anal Mach Intell. 2007. PMID: 17170481
-
Statistical shape models for 3D medical image segmentation: a review.Med Image Anal. 2009 Aug;13(4):543-63. doi: 10.1016/j.media.2009.05.004. Epub 2009 May 27. Med Image Anal. 2009. PMID: 19525140 Review.
Cited by
-
A local average distance descriptor for flexible protein structure comparison.BMC Bioinformatics. 2014 Apr 2;15:95. doi: 10.1186/1471-2105-15-95. BMC Bioinformatics. 2014. PMID: 24694083 Free PMC article.
-
Three-Dimensional Krawtchouk Descriptors for Protein Local Surface Shape Comparison.Pattern Recognit. 2019 Sep;93:534-545. doi: 10.1016/j.patcog.2019.05.019. Epub 2019 May 8. Pattern Recognit. 2019. PMID: 32042209 Free PMC article.
-
3DMolNavi: a web-based retrieval and navigation tool for flexible molecular shape comparison.BMC Bioinformatics. 2012 May 14;13:95. doi: 10.1186/1471-2105-13-95. BMC Bioinformatics. 2012. PMID: 22583488 Free PMC article.
-
Automated shape-based clustering of 3D immunoglobulin protein structures in chronic lymphocytic leukemia.BMC Bioinformatics. 2018 Nov 20;19(Suppl 14):414. doi: 10.1186/s12859-018-2381-1. BMC Bioinformatics. 2018. PMID: 30453883 Free PMC article.
References
-
- Ballester P, Richards W. Ultrafast shape recognition for similarity search in molecular databases. Proceedings of the Royal Society A. 2007;463(2081):1307–1321. doi: 10.1098/rspa.2007.1823. - DOI
-
- Grant J, Pickup B. A Gaussian description of molecular shape. Journal of Physical Chemistry. 1995;99(11):3503–3510. doi: 10.1021/j100011a016. - DOI
Publication types
MeSH terms
Substances
LinkOut - more resources
Full Text Sources