Inferring the functions of longevity genes with modular subnetwork biomarkers of Caenorhabditis elegans aging
- PMID: 20128910
- PMCID: PMC2872873
- DOI: 10.1186/gb-2010-11-2-r13
Inferring the functions of longevity genes with modular subnetwork biomarkers of Caenorhabditis elegans aging
Abstract
A central goal of biogerontology is to identify robust gene-expression biomarkers of aging. Here we develop a method where the biomarkers are networks of genes selected based on age-dependent activity and a graph-theoretic property called modularity. Tested on Caenorhabditis elegans, our algorithm yields better biomarkers than previous methods - they are more conserved across studies and better predictors of age. We apply these modular biomarkers to assign novel aging-related functions to poorly characterized longevity genes.
Figures
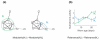
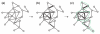
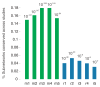
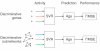
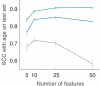
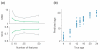
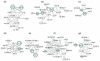
Similar articles
-
Predicting aging/longevity-related genes in the nematode Caenorhabditis elegans.Chem Biodivers. 2007 Nov;4(11):2639-55. doi: 10.1002/cbdv.200790216. Chem Biodivers. 2007. PMID: 18027377
-
RNAi screens to identify components of gene networks that modulate aging in Caenorhabditis elegans.Brief Funct Genomics. 2010 Jan;9(1):53-64. doi: 10.1093/bfgp/elp051. Epub 2010 Jan 6. Brief Funct Genomics. 2010. PMID: 20053814 Free PMC article. Review.
-
Ageing: Microarraying mortality.Nature. 2003 Jul 17;424(6946):259-61. doi: 10.1038/424259a. Nature. 2003. PMID: 12867961 No abstract available.
-
Longevity, genes, and aging.Science. 1996 Jul 5;273(5271):54-9. doi: 10.1126/science.273.5271.54. Science. 1996. PMID: 8658195 Review.
-
The nuclear hormone receptor DAF-12 has opposing effects on Caenorhabditis elegans lifespan and regulates genes repressed in multiple long-lived worms.Aging Cell. 2006 Apr;5(2):127-38. doi: 10.1111/j.1474-9726.2006.00203.x. Aging Cell. 2006. PMID: 16626392
Cited by
-
Integrative approaches for finding modular structure in biological networks.Nat Rev Genet. 2013 Oct;14(10):719-32. doi: 10.1038/nrg3552. Nat Rev Genet. 2013. PMID: 24045689 Free PMC article. Review.
-
An integer linear programming approach for finding deregulated subgraphs in regulatory networks.Nucleic Acids Res. 2012 Mar;40(6):e43. doi: 10.1093/nar/gkr1227. Epub 2011 Dec 30. Nucleic Acids Res. 2012. PMID: 22210863 Free PMC article.
-
Improving Analysis and Annotation of Microarray Data with Protein Interactions.Methods Mol Biol. 2022;2401:51-68. doi: 10.1007/978-1-0716-1839-4_5. Methods Mol Biol. 2022. PMID: 34902122
-
Optimally discriminative subnetwork markers predict response to chemotherapy.Bioinformatics. 2011 Jul 1;27(13):i205-13. doi: 10.1093/bioinformatics/btr245. Bioinformatics. 2011. PMID: 21685072 Free PMC article.
-
Integrative computational biology for cancer research.Hum Genet. 2011 Oct;130(4):465-81. doi: 10.1007/s00439-011-0983-z. Epub 2011 Apr 22. Hum Genet. 2011. PMID: 21691773 Free PMC article. Review.
References
Publication types
MeSH terms
Substances
LinkOut - more resources
Full Text Sources