Cancerous breast lesions on dynamic contrast-enhanced MR images: computerized characterization for image-based prognostic markers
- PMID: 20123903
- PMCID: PMC2826695
- DOI: 10.1148/radiol.09090838
Cancerous breast lesions on dynamic contrast-enhanced MR images: computerized characterization for image-based prognostic markers
Abstract
Purpose: To assess the performance of computer-extracted dynamic contrast material-enhanced (DCE) magnetic resonance (MR) imaging kinetic and morphologic features in the differentiation of invasive versus noninvasive breast lesions and metastatic versus nonmetastatic breast lesions.
Materials and methods: In this institutional review board-approved HIPAA-compliant study, in which the requirement for informed patient consent was waived, breast MR images were retrospectively collected. The images had been obtained with a 1.5-T MR unit by using a gadodiamide-enhanced T1-weighted spoiled gradient-recalled acquisition in the steady state sequence. The breast MR imaging database contained 132 benign, 71 ductal carcinoma in situ (DCIS), and 150 invasive ductal carcinoma (IDC) lesions. Fifty-four IDC lesions were associated with metastasis-positive lymph nodes (LNs), and 64 IDC lesions were associated with negative LNs. Lesion segmentation and extraction of morphologic and kinetic features were automatically performed by a laboratory-developed computer workstation. Features were first selected by using stepwise linear discriminant analysis and then merged by using Bayesian neural networks. Lesion classification performance was assessed with receiver operating characteristic analysis.
Results: Differentiation of DCIS from IDC lesions yielded an area under the receiver operating characteristic curve (AUC) of 0.83 +/- 0.03 (standard error). AUCs were 0.85 +/- 0.02 for differentiation between IDC and benign lesions and 0.79 +/- 0.03 for differentiation between DCIS and benign lesions. Differentiation between IDC lesions associated with positive LNs and IDC lesions associated with negative LNs yielded an AUC of 0.82 +/- 0.04. AUCs were 0.86 +/- 0.03 for differentiation between IDC lesions associated with positive LNs and benign lesions and 0.83 +/- 0.03 for differentiation between IDC lesions associated with negative LNs and benign lesions.
Conclusion: Computer-aided diagnosis of breast DCE MR imaging-depicted lesions was extended from the task of discriminating between malignant and benign lesions to the prognostic tasks of distinguishing between noninvasive and invasive lesions and discriminating between metastatic and nonmetastatic lesions, yielding MR imaging-based prognostic markers.
Supplemental material: http://radiology.rsna.org/lookup/suppl/doi:10.1148/radiol.09090838/-/DC1.
(c) RSNA, 2010
Figures
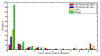
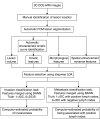
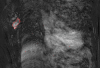
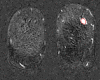
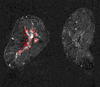
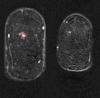
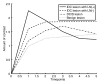
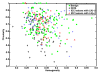
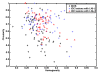
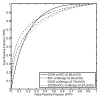
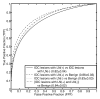
Similar articles
-
Diffusion-weighted imaging improves the diagnostic accuracy of conventional 3.0-T breast MR imaging.Radiology. 2010 Jul;256(1):64-73. doi: 10.1148/radiol.10091367. Radiology. 2010. PMID: 20574085 Free PMC article.
-
A computer-aided diagnosis system for breast DCE-MRI at high spatiotemporal resolution.Med Phys. 2016 Jan;43(1):84. doi: 10.1118/1.4937787. Med Phys. 2016. PMID: 26745902
-
Improving the Accuracy of Computer-aided Diagnosis for Breast MR Imaging by Differentiating between Mass and Nonmass Lesions.Radiology. 2016 Mar;278(3):679-88. doi: 10.1148/radiol.2015150241. Epub 2015 Sep 18. Radiology. 2016. PMID: 26383229
-
Discrimination of benign and malignant breast lesions by using shutter-speed dynamic contrast-enhanced MR imaging.Radiology. 2011 Nov;261(2):394-403. doi: 10.1148/radiol.11102413. Epub 2011 Aug 9. Radiology. 2011. PMID: 21828189 Free PMC article.
-
Using quantitative image analysis to classify axillary lymph nodes on breast MRI: a new application for the Z 0011 Era.Eur J Radiol. 2015 Mar;84(3):392-397. doi: 10.1016/j.ejrad.2014.12.003. Epub 2014 Dec 15. Eur J Radiol. 2015. PMID: 25547328 Free PMC article. Review.
Cited by
-
Radiologist-Level Performance by Using Deep Learning for Segmentation of Breast Cancers on MRI Scans.Radiol Artif Intell. 2021 Dec 15;4(1):e200231. doi: 10.1148/ryai.200231. eCollection 2022 Jan. Radiol Artif Intell. 2021. PMID: 35146431 Free PMC article.
-
Role of texture analysis in breast MRI as a cancer biomarker: A review.J Magn Reson Imaging. 2019 Apr;49(4):927-938. doi: 10.1002/jmri.26556. Epub 2018 Nov 3. J Magn Reson Imaging. 2019. PMID: 30390383 Free PMC article. Review.
-
Automatic Segmentation of Breast Carcinomas from DCE-MRI using a Statistical Learning Algorithm.Proc IEEE Int Symp Biomed Imaging. 2012 May;2012:122-125. doi: 10.1109/ISBI.2012.6235499. Epub 2012 Jul 12. Proc IEEE Int Symp Biomed Imaging. 2012. PMID: 28603582 Free PMC article.
-
DCE-MRI Texture Features for Early Prediction of Breast Cancer Therapy Response.Tomography. 2017 Mar;3(1):23-32. doi: 10.18383/j.tom.2016.00241. Tomography. 2017. PMID: 28691102 Free PMC article.
-
Application of Radiomics and Decision Support Systems for Breast MR Differential Diagnosis.Comput Math Methods Med. 2018 Sep 23;2018:7417126. doi: 10.1155/2018/7417126. eCollection 2018. Comput Math Methods Med. 2018. PMID: 30344618 Free PMC article. Review.
References
-
- Schnall MD. Breast MR imaging. Radiol Clin North Am 2003;41:43–50 - PubMed
-
- Morris EA. Breast cancer imaging with MRI. Radiol Clin North Am 2002;40:443–466 - PubMed
-
- Kuhl CK, Schild HH. Dynamic image interpretation of MRI of the breast. J Magn Reson Imaging 2000;12:965–974 - PubMed
-
- Kuhl CK, Mielcareck P, Klaschik S, et al. Dynamic breast MR imaging: are signal intensity time course data useful for differential diagnosis of enhancing lesions?. Radiology 1999;211:101–110 - PubMed
-
- Bartella L, Smith CS, Dershaw DD, Liberman L. Imaging breast cancer. Radiol Clin North Am 2007;45:45–67 - PubMed
Publication types
MeSH terms
Substances
Grants and funding
LinkOut - more resources
Full Text Sources
Other Literature Sources
Medical