Derivation of an amino acid similarity matrix for peptide: MHC binding and its application as a Bayesian prior
- PMID: 19948066
- PMCID: PMC2790471
- DOI: 10.1186/1471-2105-10-394
Derivation of an amino acid similarity matrix for peptide: MHC binding and its application as a Bayesian prior
Abstract
Background: Experts in peptide:MHC binding studies are often able to estimate the impact of a single residue substitution based on a heuristic understanding of amino acid similarity in an experimental context. Our aim is to quantify this measure of similarity to improve peptide:MHC binding prediction methods. This should help compensate for holes and bias in the sequence space coverage of existing peptide binding datasets.
Results: Here, a novel amino acid similarity matrix (PMBEC) is directly derived from the binding affinity data of combinatorial peptide mixtures. Like BLOSUM62, this matrix captures well-known physicochemical properties of amino acid residues. However, PMBEC differs markedly from existing matrices in cases where residue substitution involves a reversal of electrostatic charge. To demonstrate its usefulness, we have developed a new peptide:MHC class I binding prediction method, using the matrix as a Bayesian prior. We show that the new method can compensate for missing information on specific residues in the training data. We also carried out a large-scale benchmark, and its results indicate that prediction performance of the new method is comparable to that of the best neural network based approaches for peptide:MHC class I binding.
Conclusion: A novel amino acid similarity matrix has been derived for peptide:MHC binding interactions. One prominent feature of the matrix is that it disfavors substitution of residues with opposite charges. Given that the matrix was derived from experimentally determined peptide:MHC binding affinity measurements, this feature is likely shared by all peptide:protein interactions. In addition, we have demonstrated the usefulness of the matrix as a Bayesian prior in an improved scoring-matrix based peptide:MHC class I prediction method. A software implementation of the method is available at: http://www.mhc-pathway.net/smmpmbec.
Figures
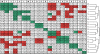
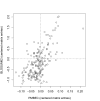
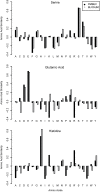
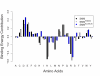
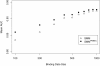
Similar articles
-
NN-align. An artificial neural network-based alignment algorithm for MHC class II peptide binding prediction.BMC Bioinformatics. 2009 Sep 18;10:296. doi: 10.1186/1471-2105-10-296. BMC Bioinformatics. 2009. PMID: 19765293 Free PMC article.
-
The PickPocket method for predicting binding specificities for receptors based on receptor pocket similarities: application to MHC-peptide binding.Bioinformatics. 2009 May 15;25(10):1293-9. doi: 10.1093/bioinformatics/btp137. Epub 2009 Mar 17. Bioinformatics. 2009. PMID: 19297351 Free PMC article.
-
Prediction of MHC class II binding affinity using SMM-align, a novel stabilization matrix alignment method.BMC Bioinformatics. 2007 Jul 4;8:238. doi: 10.1186/1471-2105-8-238. BMC Bioinformatics. 2007. PMID: 17608956 Free PMC article.
-
MHC class I/peptide interactions: binding specificity and kinetics.J Mol Recognit. 1993 Jun;6(2):59-69. doi: 10.1002/jmr.300060204. J Mol Recognit. 1993. PMID: 8305252 Review.
-
Consensus motifs and peptide ligands of MHC class I molecules.Semin Immunol. 1993 Apr;5(2):81-94. doi: 10.1006/smim.1993.1012. Semin Immunol. 1993. PMID: 7684938 Review.
Cited by
-
Predicting MHC class I binder: existing approaches and a novel recurrent neural network solution.Brief Bioinform. 2021 Nov 5;22(6):bbab216. doi: 10.1093/bib/bbab216. Brief Bioinform. 2021. PMID: 34131696 Free PMC article.
-
The Immune Epitope Database and Analysis Resource Program 2003-2018: reflections and outlook.Immunogenetics. 2020 Feb;72(1-2):57-76. doi: 10.1007/s00251-019-01137-6. Epub 2019 Nov 25. Immunogenetics. 2020. PMID: 31761977 Free PMC article. Review.
-
Best practices for bioinformatic characterization of neoantigens for clinical utility.Genome Med. 2019 Aug 28;11(1):56. doi: 10.1186/s13073-019-0666-2. Genome Med. 2019. PMID: 31462330 Free PMC article. Review.
-
neoANT-HILL: an integrated tool for identification of potential neoantigens.BMC Med Genomics. 2020 Feb 22;13(1):30. doi: 10.1186/s12920-020-0694-1. BMC Med Genomics. 2020. PMID: 32087727 Free PMC article.
-
Uncovering the peptide-binding specificities of HLA-C: a general strategy to determine the specificity of any MHC class I molecule.J Immunol. 2014 Nov 15;193(10):4790-802. doi: 10.4049/jimmunol.1401689. Epub 2014 Oct 13. J Immunol. 2014. PMID: 25311805 Free PMC article.
References
-
- Dayhoff MO, Schwartz RM, Orcutt BC. A model of evolutionary change in proteins. Atlas of Protein Sequence and Structure. 1978. pp. 345–352.
-
- Peters B, Bulik S, Tampe R, van Endert PM, Holzhutter H-G. Identifying MHC Class I Epitopes by Predicting the TAP Transport Efficiency of Epitope Precursors. J Immunol. 2003;171(4):1741–1749. - PubMed
Publication types
MeSH terms
Substances
Grants and funding
LinkOut - more resources
Full Text Sources
Other Literature Sources
Research Materials