Supervised risk predictor of breast cancer based on intrinsic subtypes
- PMID: 19204204
- PMCID: PMC2667820
- DOI: 10.1200/JCO.2008.18.1370
Supervised risk predictor of breast cancer based on intrinsic subtypes
Abstract
PURPOSE To improve on current standards for breast cancer prognosis and prediction of chemotherapy benefit by developing a risk model that incorporates the gene expression-based "intrinsic" subtypes luminal A, luminal B, HER2-enriched, and basal-like. METHODS A 50-gene subtype predictor was developed using microarray and quantitative reverse transcriptase polymerase chain reaction data from 189 prototype samples. Test sets from 761 patients (no systemic therapy) were evaluated for prognosis, and 133 patients were evaluated for prediction of pathologic complete response (pCR) to a taxane and anthracycline regimen.
Results: The intrinsic subtypes as discrete entities showed prognostic significance (P = 2.26E-12) and remained significant in multivariable analyses that incorporated standard parameters (estrogen receptor status, histologic grade, tumor size, and node status). A prognostic model for node-negative breast cancer was built using intrinsic subtype and clinical information. The C-index estimate for the combined model (subtype and tumor size) was a significant improvement on either the clinicopathologic model or subtype model alone. The intrinsic subtype model predicted neoadjuvant chemotherapy efficacy with a negative predictive value for pCR of 97%. CONCLUSION Diagnosis by intrinsic subtype adds significant prognostic and predictive information to standard parameters for patients with breast cancer. The prognostic properties of the continuous risk score will be of value for the management of node-negative breast cancers. The subtypes and risk score can also be used to assess the likelihood of efficacy from neoadjuvant chemotherapy.
Conflict of interest statement
Authors' disclosures of potential conflicts of interest and author contributions are found at the end of this article.
Figures
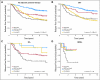
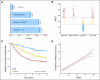
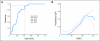
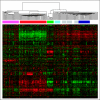
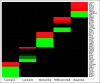
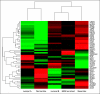
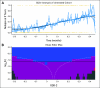
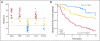
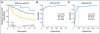
Republished in
-
Supervised Risk Predictor of Breast Cancer Based on Intrinsic Subtypes.J Clin Oncol. 2023 Sep 10;41(26):4192-4199. doi: 10.1200/JCO.22.02511. J Clin Oncol. 2023. PMID: 37672882
Comment in
-
Introducing molecular subtyping of breast cancer into the clinic?J Clin Oncol. 2009 Mar 10;27(8):1153-4. doi: 10.1200/JCO.2008.20.6276. Epub 2009 Feb 9. J Clin Oncol. 2009. PMID: 19204193 No abstract available.
Similar articles
-
Supervised Risk Predictor of Breast Cancer Based on Intrinsic Subtypes.J Clin Oncol. 2023 Sep 10;41(26):4192-4199. doi: 10.1200/JCO.22.02511. J Clin Oncol. 2023. PMID: 37672882
-
The triple negative paradox: primary tumor chemosensitivity of breast cancer subtypes.Clin Cancer Res. 2007 Apr 15;13(8):2329-34. doi: 10.1158/1078-0432.CCR-06-1109. Clin Cancer Res. 2007. PMID: 17438091
-
Clinical implications of the intrinsic molecular subtypes of breast cancer.Breast. 2015 Nov;24 Suppl 2:S26-35. doi: 10.1016/j.breast.2015.07.008. Epub 2015 Aug 5. Breast. 2015. PMID: 26253814 Review.
-
Prognostic value of Ki67 expression in HR-negative breast cancer before and after neoadjuvant chemotherapy.Int J Clin Exp Pathol. 2014 Sep 15;7(10):6862-70. eCollection 2014. Int J Clin Exp Pathol. 2014. PMID: 25400769 Free PMC article.
-
Overview of resistance to systemic therapy in patients with breast cancer.Adv Exp Med Biol. 2007;608:1-22. doi: 10.1007/978-0-387-74039-3_1. Adv Exp Med Biol. 2007. PMID: 17993229 Review.
Cited by
-
Differential chromatin accessibility and transcriptional dynamics define breast cancer subtypes and their lineages.Nat Cancer. 2024 Oct 30. doi: 10.1038/s43018-024-00773-6. Online ahead of print. Nat Cancer. 2024. PMID: 39478117
-
A multi-modal single-cell and spatial expression map of metastatic breast cancer biopsies across clinicopathological features.Nat Med. 2024 Oct 30. doi: 10.1038/s41591-024-03215-z. Online ahead of print. Nat Med. 2024. PMID: 39478111
-
The Influence of Microbiota on Breast Cancer: A Review.Cancers (Basel). 2024 Oct 13;16(20):3468. doi: 10.3390/cancers16203468. Cancers (Basel). 2024. PMID: 39456562 Free PMC article. Review.
-
Development and validation of a gene expression-based Breast Cancer Purity Score.NPJ Precis Oncol. 2024 Oct 24;8(1):242. doi: 10.1038/s41698-024-00730-7. NPJ Precis Oncol. 2024. PMID: 39448787 Free PMC article.
-
Single-cell transcriptional atlas of human breast cancers and model systems.Clin Transl Med. 2024 Oct;14(10):e70044. doi: 10.1002/ctm2.70044. Clin Transl Med. 2024. PMID: 39417215 Free PMC article.
References
-
- van 't Veer LJ, Dai H, van de Vijver MJ, et al. Gene expression profiling predicts clinical outcome of breast cancer. Nature. 2002;415:530–536. - PubMed
-
- van't Veer LJ, Paik S, Hayes DF. Gene expression profiling of breast cancer: A new tumor marker. J Clin Oncol. 2005;23:1631–1635. - PubMed
-
- Paik S, Shak S, Tang G, et al. A multigene assay to predict recurrence of tamoxifen-treated, node-negative breast cancer. N Engl J Med. 2004;351:2817–2826. - PubMed
-
- Paik S, Tang G, Shak S, et al. Gene expression and benefit of chemotherapy in women with node-negative, estrogen receptor-positive breast cancer. J Clin Oncol. 2006;24:3726–3734. - PubMed
Publication types
MeSH terms
Substances
Grants and funding
LinkOut - more resources
Full Text Sources
Other Literature Sources
Medical
Research Materials
Miscellaneous