The evolution of strand preference in simulated RNA replicators with strand displacement: implications for the origin of transcription
- PMID: 18694481
- PMCID: PMC2648946
- DOI: 10.1186/1745-6150-3-33
The evolution of strand preference in simulated RNA replicators with strand displacement: implications for the origin of transcription
Abstract
Background: The simplest conceivable example of evolving systems is RNA molecules that can replicate themselves. Since replication produces a new RNA strand complementary to a template, all templates would eventually become double-stranded and, hence, become unavailable for replication. Thus the problem of how to separate the two strands is considered a major issue for the early evolution of self-replicating RNA. One biologically plausible way to copy a double-stranded RNA is to displace a preexisting strand by a newly synthesized strand. Such copying can in principle be initiated from either the (+) or (-) strand of a double-stranded RNA. Assuming that only one of them, say (+), can act as replicase when single-stranded, strand displacement produces a new replicase if the (-) strand is the template. If, however, the (+) strand is the template, it produces a new template (but no replicase). Modern transcription exhibits extreme strand preference wherein anti-sense strands are always the template. Likewise, replication by strand displacement seems optimal if it also exhibits extreme strand preference wherein (-) strands are always the template, favoring replicase production. Here we investigate whether such strand preference can evolve in a simple RNA replicator system with strand displacement.
Results: We first studied a simple mathematical model of the replicator dynamics. Our results indicated that if the system is well-mixed, there is no selective force acting upon strand preference per se. Next, we studied an individual-based simulation model to investigate the evolution of strand preference under finite diffusion. Interestingly, the results showed that selective forces "emerge" because of finite diffusion. Strikingly, the direction of the strand preference that evolves [i.e. (+) or (-) strand excess] is a complex non-monotonic function of the diffusion intensity. The mechanism underlying this behavior is elucidated. Furthermore, a speciation-like phenomenon is observed under certain conditions: two extreme replication strategies, namely replicase producers and template producers, emerge and coexist among competing replicators.
Conclusion: Finite diffusion enables the evolution of strand preference, the direction of which is a non-monotonic function of the diffusion intensity. By identifying the conditions under which strand preference evolves, this study provides an insight into how a rudimentary transcription-like pattern might have emerged in an RNA-based replicator system.
Reviewers: This article was reviewed by Eugene V Koonin, Rob Kinght and István Scheuring (nominated by David H Ardell). For the full reviews, please go to the Reviewers' comments section.
Figures
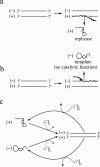
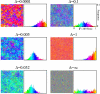
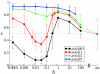
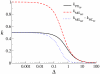
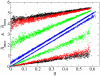
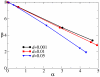
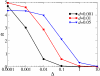
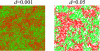
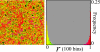
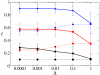
Similar articles
-
Template role of double-stranded RNA in tombusvirus replication.J Virol. 2014 May;88(10):5638-51. doi: 10.1128/JVI.03842-13. Epub 2014 Mar 5. J Virol. 2014. PMID: 24600009 Free PMC article.
-
On the origin of DNA genomes: evolution of the division of labor between template and catalyst in model replicator systems.PLoS Comput Biol. 2011 Mar;7(3):e1002024. doi: 10.1371/journal.pcbi.1002024. Epub 2011 Mar 24. PLoS Comput Biol. 2011. PMID: 21455287 Free PMC article.
-
Replication of partial double-stranded RNAs by Qβ replicase.Biochem Biophys Res Commun. 2015 Nov 13;467(2):293-6. doi: 10.1016/j.bbrc.2015.09.169. Epub 2015 Oct 12. Biochem Biophys Res Commun. 2015. PMID: 26441086
-
Metabolically Coupled Replicator Systems: Overview of an RNA-world model concept of prebiotic evolution on mineral surfaces.J Theor Biol. 2015 Sep 21;381:39-54. doi: 10.1016/j.jtbi.2015.06.002. Epub 2015 Jun 15. J Theor Biol. 2015. PMID: 26087284 Review.
-
Strand transfer events during HIV-1 reverse transcription.Virus Res. 2008 Jun;134(1-2):19-38. doi: 10.1016/j.virusres.2007.12.017. Epub 2008 Feb 14. Virus Res. 2008. PMID: 18279992 Review.
Cited by
-
The phylogenetic forest and the quest for the elusive tree of life.Cold Spring Harb Symp Quant Biol. 2009;74:205-13. doi: 10.1101/sqb.2009.74.006. Epub 2009 Aug 17. Cold Spring Harb Symp Quant Biol. 2009. PMID: 19687142 Free PMC article. Review.
-
Computer simulations of Template-Directed RNA Synthesis driven by temperature cycling in diverse sequence mixtures.PLoS Comput Biol. 2022 Aug 24;18(8):e1010458. doi: 10.1371/journal.pcbi.1010458. eCollection 2022 Aug. PLoS Comput Biol. 2022. PMID: 36001640 Free PMC article.
-
Evolutionary dynamics of RNA-like replicator systems: A bioinformatic approach to the origin of life.Phys Life Rev. 2012 Sep;9(3):219-63. doi: 10.1016/j.plrev.2012.06.001. Epub 2012 Jun 13. Phys Life Rev. 2012. PMID: 22727399 Free PMC article. Review.
-
Computer simulation on the cooperation of functional molecules during the early stages of evolution.PLoS One. 2012;7(4):e35454. doi: 10.1371/journal.pone.0035454. Epub 2012 Apr 13. PLoS One. 2012. PMID: 22514745 Free PMC article.
-
The fundamental units, processes and patterns of evolution, and the tree of life conundrum.Biol Direct. 2009 Sep 29;4:33. doi: 10.1186/1745-6150-4-33. Biol Direct. 2009. PMID: 19788730 Free PMC article.
References
-
- Bartel DP. In: The RNA world. 2. Gesteland RF, Cech TR, Atkins JF, editor. Cold Spring Harbor:Cold Spring Harbor Laboratory Press; 1998. Re-creating an RNA replicase; pp. 143–162.
Publication types
MeSH terms
Substances
LinkOut - more resources
Full Text Sources