Unraveling protein networks with power graph analysis
- PMID: 18617988
- PMCID: PMC2424176
- DOI: 10.1371/journal.pcbi.1000108
Unraveling protein networks with power graph analysis
Abstract
Networks play a crucial role in computational biology, yet their analysis and representation is still an open problem. Power Graph Analysis is a lossless transformation of biological networks into a compact, less redundant representation, exploiting the abundance of cliques and bicliques as elementary topological motifs. We demonstrate with five examples the advantages of Power Graph Analysis. Investigating protein-protein interaction networks, we show how the catalytic subunits of the casein kinase II complex are distinguishable from the regulatory subunits, how interaction profiles and sequence phylogeny of SH3 domains correlate, and how false positive interactions among high-throughput interactions are spotted. Additionally, we demonstrate the generality of Power Graph Analysis by applying it to two other types of networks. We show how power graphs induce a clustering of both transcription factors and target genes in bipartite transcription networks, and how the erosion of a phosphatase domain in type 22 non-receptor tyrosine phosphatases is detected. We apply Power Graph Analysis to high-throughput protein interaction networks and show that up to 85% (56% on average) of the information is redundant. Experimental networks are more compressible than rewired ones of same degree distribution, indicating that experimental networks are rich in cliques and bicliques. Power Graphs are a novel representation of networks, which reduces network complexity by explicitly representing re-occurring network motifs. Power Graphs compress up to 85% of the edges in protein interaction networks and are applicable to all types of networks such as protein interactions, regulatory networks, or homology networks.
Conflict of interest statement
The authors have declared that no competing interests exist.
Figures
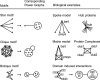
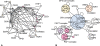
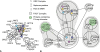
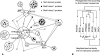
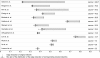
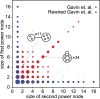
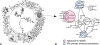
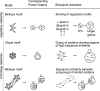
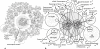
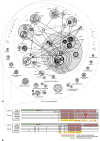
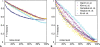
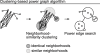
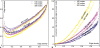
Similar articles
-
Architecture of basic building blocks in protein and domain structural interaction networks.Bioinformatics. 2005 Apr 15;21(8):1479-86. doi: 10.1093/bioinformatics/bti240. Epub 2004 Dec 21. Bioinformatics. 2005. PMID: 15613386
-
Joint evolutionary trees: a large-scale method to predict protein interfaces based on sequence sampling.PLoS Comput Biol. 2009 Jan;5(1):e1000267. doi: 10.1371/journal.pcbi.1000267. Epub 2009 Jan 23. PLoS Comput Biol. 2009. PMID: 19165315 Free PMC article.
-
A lock-and-key model for protein-protein interactions.Bioinformatics. 2006 Aug 15;22(16):2012-9. doi: 10.1093/bioinformatics/btl338. Epub 2006 Jun 20. Bioinformatics. 2006. PMID: 16787977
-
Domain-mediated protein interaction prediction: From genome to network.FEBS Lett. 2012 Aug 14;586(17):2751-63. doi: 10.1016/j.febslet.2012.04.027. Epub 2012 May 3. FEBS Lett. 2012. PMID: 22561014 Review.
-
Discerning molecular interactions: A comprehensive review on biomolecular interaction databases and network analysis tools.Gene. 2018 Feb 5;642:84-94. doi: 10.1016/j.gene.2017.11.028. Epub 2017 Nov 10. Gene. 2018. PMID: 29129810 Review.
Cited by
-
Combing the hairball with BioFabric: a new approach for visualization of large networks.BMC Bioinformatics. 2012 Oct 27;13:275. doi: 10.1186/1471-2105-13-275. BMC Bioinformatics. 2012. PMID: 23102059 Free PMC article.
-
Protein interaction networks--more than mere modules.PLoS Comput Biol. 2010 Jan 29;6(1):e1000659. doi: 10.1371/journal.pcbi.1000659. PLoS Comput Biol. 2010. PMID: 20126533 Free PMC article.
-
Analysis of weighted co-regulatory networks in maize provides insights into new genes and regulatory mechanisms related to inositol phosphate metabolism.BMC Genomics. 2016 Feb 24;17:129. doi: 10.1186/s12864-016-2476-x. BMC Genomics. 2016. PMID: 26911482 Free PMC article.
-
Google goes cancer: improving outcome prediction for cancer patients by network-based ranking of marker genes.PLoS Comput Biol. 2012;8(5):e1002511. doi: 10.1371/journal.pcbi.1002511. Epub 2012 May 17. PLoS Comput Biol. 2012. PMID: 22615549 Free PMC article.
-
FunMod: a Cytoscape plugin for identifying functional modules in undirected protein-protein networks.Genomics Proteomics Bioinformatics. 2014 Aug;12(4):178-86. doi: 10.1016/j.gpb.2014.05.002. Epub 2014 Aug 19. Genomics Proteomics Bioinformatics. 2014. PMID: 25153667 Free PMC article.
References
-
- Fields S, Song O. A novel genetic system to detect protein-protein interactions. Nature. 1989;340:245–246. Available: http://dx.doi.org/10.1038/340245a0. - DOI - PubMed
-
- Rigaut G, Shevchenko A, Rutz B, Wilm M, Mann M, et al. A generic protein purification method for protein complex characterization and proteome exploration. Nat Biotechnol. 1999;17:1030–1032. Available: http://dx.doi.org/10.1038/13732. - DOI - PubMed
-
- Mann M, Hendrickson RC, Pandey A. Analysis of proteins and proteomes by mass spectrometry. Annu Rev Biochem. 2001;70:437–473. Available: http://dx.doi.org/10.1146/annurev.biochem.70.1.437. - DOI - PubMed
-
- Gavin AC, Aloy P, Grandi P, Krause R, Boesche M, et al. Proteome survey reveals modularity of the yeast cell machinery. Nature. 2006;440:631–636. Available: http://dx.doi.org/10.1038/nature04532. - DOI - PubMed
-
- Ito T, Chiba T, Ozawa R, Yoshida M, Hattori M, et al. A comprehensive two-hybrid analysis to explore the yeast protein interactome. Proc Natl Acad Sci U S A. 2001;98:4569–4574. Available: http://dx.doi.org/10.1073/pnas.061034498. - DOI - PMC - PubMed
Publication types
MeSH terms
Substances
LinkOut - more resources
Full Text Sources