Supervised inference of gene-regulatory networks
- PMID: 18177495
- PMCID: PMC2266705
- DOI: 10.1186/1471-2105-9-2
Supervised inference of gene-regulatory networks
Abstract
Background: Inference of protein interaction networks from various sources of data has become an important topic of both systems and computational biology. Here we present a supervised approach to identification of gene expression regulatory networks.
Results: The method is based on a kernel approach accompanied with genetic programming. As a data source, the method utilizes gene expression time series for prediction of interactions among regulatory proteins and their target genes. The performance of the method was verified using Saccharomyces cerevisiae cell cycle and DNA/RNA/protein biosynthesis gene expression data. The results were compared with independent data sources. Finally, a prediction of novel interactions within yeast gene expression circuits has been performed.
Conclusion: Results show that our algorithm gives, in most cases, results identical with the independent experiments, when compared with the YEASTRACT database. In several cases our algorithm gives predictions of novel interactions which have not been reported.
Figures
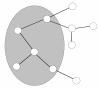
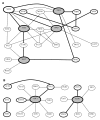
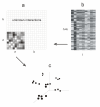
Similar articles
-
Improving protein protein interaction prediction based on phylogenetic information using a least-squares support vector machine.Ann N Y Acad Sci. 2007 Dec;1115:154-67. doi: 10.1196/annals.1407.005. Epub 2007 Oct 9. Ann N Y Acad Sci. 2007. PMID: 17925357
-
Comparative evaluation of reverse engineering gene regulatory networks with relevance networks, graphical gaussian models and bayesian networks.Bioinformatics. 2006 Oct 15;22(20):2523-31. doi: 10.1093/bioinformatics/btl391. Epub 2006 Jul 14. Bioinformatics. 2006. PMID: 16844710
-
Protein network inference from multiple genomic data: a supervised approach.Bioinformatics. 2004 Aug 4;20 Suppl 1:i363-70. doi: 10.1093/bioinformatics/bth910. Bioinformatics. 2004. PMID: 15262821
-
Using product kernels to predict protein interactions.Adv Biochem Eng Biotechnol. 2008;110:215-45. doi: 10.1007/10_2007_084. Adv Biochem Eng Biotechnol. 2008. PMID: 17922100 Review.
-
Gene regulatory network inference: data integration in dynamic models-a review.Biosystems. 2009 Apr;96(1):86-103. doi: 10.1016/j.biosystems.2008.12.004. Epub 2008 Dec 27. Biosystems. 2009. PMID: 19150482 Review.
Cited by
-
Kinetic Modeling and Meta-Analysis of the Bacillus subtilis SigB Regulon during Spore Germination and Outgrowth.Microorganisms. 2021 Jan 5;9(1):112. doi: 10.3390/microorganisms9010112. Microorganisms. 2021. PMID: 33466511 Free PMC article.
-
A semi-supervised learning approach to predict synthetic genetic interactions by combining functional and topological properties of functional gene network.BMC Bioinformatics. 2010 Jun 24;11:343. doi: 10.1186/1471-2105-11-343. BMC Bioinformatics. 2010. PMID: 20573270 Free PMC article.
-
Gene regulatory network inference: evaluation and application to ovarian cancer allows the prioritization of drug targets.Genome Med. 2012 May 1;4(5):41. doi: 10.1186/gm340. Genome Med. 2012. PMID: 22548828 Free PMC article.
References
-
- Scholkopf B, Smola AJ. Learning with kernels. Cambridge, Massachusets , The MIT Press; 2002.
-
- Wang H, Segal E, Ben-Hur A, Koller D, Brutlag DL. Identifying protein-protein interaction sites on a genome-wide scale. In: LK Saul YWLB, editor. Advaneces in Neural Information Processing Systems 17. Cambridge, MA , MIT press; 2005. pp. 1465–1472.
Publication types
MeSH terms
Substances
LinkOut - more resources
Full Text Sources
Molecular Biology Databases
Research Materials