Understanding network concepts in modules
- PMID: 17547772
- PMCID: PMC3238286
- DOI: 10.1186/1752-0509-1-24
Understanding network concepts in modules
Abstract
Background: Network concepts are increasingly used in biology and genetics. For example, the clustering coefficient has been used to understand network architecture; the connectivity (also known as degree) has been used to screen for cancer targets; and the topological overlap matrix has been used to define modules and to annotate genes. Dozens of potentially useful network concepts are known from graph theory.
Results: Here we study network concepts in special types of networks, which we refer to as approximately factorizable networks. In these networks, the pairwise connection strength (adjacency) between 2 network nodes can be factored into node specific contributions, named node 'conformity'. The node conformity turns out to be highly related to the connectivity. To provide a formalism for relating network concepts to each other, we define three types of network concepts: fundamental-, conformity-based-, and approximate conformity-based concepts. Fundamental concepts include the standard definitions of connectivity, density, centralization, heterogeneity, clustering coefficient, and topological overlap. The approximate conformity-based analogs of fundamental network concepts have several theoretical advantages. First, they allow one to derive simple relationships between seemingly disparate networks concepts. For example, we derive simple relationships between the clustering coefficient, the heterogeneity, the density, the centralization, and the topological overlap. The second advantage of approximate conformity-based network concepts is that they allow one to show that fundamental network concepts can be approximated by simple functions of the connectivity in module networks.
Conclusion: Using protein-protein interaction, gene co-expression, and simulated data, we show that a) many networks comprised of module nodes are approximately factorizable and b) in these types of networks, simple relationships exist between seemingly disparate network concepts. Our results are implemented in freely available R software code, which can be downloaded from the following webpage: http://www.genetics.ucla.edu/labs/horvath/ModuleConformity/ModuleNetworks.
Figures
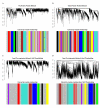
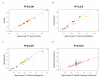
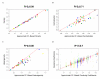
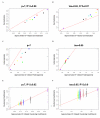
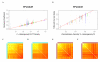
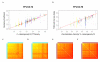
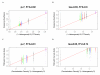
Similar articles
-
Network module detection: Affinity search technique with the multi-node topological overlap measure.BMC Res Notes. 2009 Jul 20;2:142. doi: 10.1186/1756-0500-2-142. BMC Res Notes. 2009. PMID: 19619323 Free PMC article.
-
A general framework for weighted gene co-expression network analysis.Stat Appl Genet Mol Biol. 2005;4:Article17. doi: 10.2202/1544-6115.1128. Epub 2005 Aug 12. Stat Appl Genet Mol Biol. 2005. PMID: 16646834
-
Geometric interpretation of gene coexpression network analysis.PLoS Comput Biol. 2008 Aug 15;4(8):e1000117. doi: 10.1371/journal.pcbi.1000117. PLoS Comput Biol. 2008. PMID: 18704157 Free PMC article.
-
Detecting hierarchical modularity in biological networks.Methods Mol Biol. 2009;541:145-60. doi: 10.1007/978-1-59745-243-4_7. Methods Mol Biol. 2009. PMID: 19381526 Review.
-
Biological Network Inference and analysis using SEBINI and CABIN.Methods Mol Biol. 2009;541:551-76. doi: 10.1007/978-1-59745-243-4_24. Methods Mol Biol. 2009. PMID: 19381531 Review.
Cited by
-
Ketoamide resistance and hepatitis C virus fitness in val55 variants of the NS3 serine protease.Antimicrob Agents Chemother. 2012 Apr;56(4):1907-15. doi: 10.1128/AAC.05184-11. Epub 2012 Jan 17. Antimicrob Agents Chemother. 2012. PMID: 22252823 Free PMC article.
-
Evidence of perturbations of the cytokine network in preterm labor.Am J Obstet Gynecol. 2015 Dec;213(6):836.e1-836.e18. doi: 10.1016/j.ajog.2015.07.037. Epub 2015 Jul 29. Am J Obstet Gynecol. 2015. PMID: 26232508 Free PMC article.
-
Large-scale gene co-expression network as a source of functional annotation for cattle genes.BMC Genomics. 2016 Nov 2;17(1):846. doi: 10.1186/s12864-016-3176-2. BMC Genomics. 2016. PMID: 27806696 Free PMC article.
-
Effects of hierarchical roost removal on northern long-eared bat (Myotis septentrionalis) maternity colonies.PLoS One. 2015 Jan 22;10(1):e0116356. doi: 10.1371/journal.pone.0116356. eCollection 2015. PLoS One. 2015. PMID: 25611060 Free PMC article.
-
Utilizing RNA-Seq data for de novo coexpression network inference.Bioinformatics. 2012 Jun 15;28(12):1592-7. doi: 10.1093/bioinformatics/bts245. Epub 2012 May 3. Bioinformatics. 2012. PMID: 22556371 Free PMC article.
References
Publication types
MeSH terms
Substances
Grants and funding
LinkOut - more resources
Full Text Sources
Molecular Biology Databases