Thousands of samples are needed to generate a robust gene list for predicting outcome in cancer
- PMID: 16585533
- PMCID: PMC1458674
- DOI: 10.1073/pnas.0601231103
Thousands of samples are needed to generate a robust gene list for predicting outcome in cancer
Abstract
Predicting at the time of discovery the prognosis and metastatic potential of cancer is a major challenge in current clinical research. Numerous recent studies searched for gene expression signatures that outperform traditionally used clinical parameters in outcome prediction. Finding such a signature will free many patients of the suffering and toxicity associated with adjuvant chemotherapy given to them under current protocols, even though they do not need such treatment. A reliable set of predictive genes also will contribute to a better understanding of the biological mechanism of metastasis. Several groups have published lists of predictive genes and reported good predictive performance based on them. However, the gene lists obtained for the same clinical types of patients by different groups differed widely and had only very few genes in common. This lack of agreement raised doubts about the reliability and robustness of the reported predictive gene lists, and the main source of the problem was shown to be the small number of samples that were used to generate the gene lists. Here, we introduce a previously undescribed mathematical method, probably approximately correct (PAC) sorting, for evaluating the robustness of such lists. We calculate for several published data sets the number of samples that are needed to achieve any desired level of reproducibility. For example, to achieve a typical overlap of 50% between two predictive lists of genes, breast cancer studies would need the expression profiles of several thousand early discovery patients.
Conflict of interest statement
Conflict of interest statement: No conflicts declared.
Figures
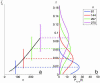
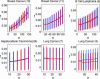
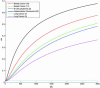
Similar articles
-
Outcome signature genes in breast cancer: is there a unique set?Bioinformatics. 2005 Jan 15;21(2):171-8. doi: 10.1093/bioinformatics/bth469. Epub 2004 Aug 12. Bioinformatics. 2005. PMID: 15308542
-
Identifying the gene signatures from gene-pathway bipartite network guarantees the robust model performance on predicting the cancer prognosis.Biomed Res Int. 2014;2014:424509. doi: 10.1155/2014/424509. Epub 2014 Jul 14. Biomed Res Int. 2014. PMID: 25126556 Free PMC article.
-
Evaluation of public cancer datasets and signatures identifies TP53 mutant signatures with robust prognostic and predictive value.BMC Cancer. 2015 Mar 26;15:179. doi: 10.1186/s12885-015-1102-7. BMC Cancer. 2015. PMID: 25886164 Free PMC article.
-
From description to causality: mechanisms of gene expression signatures in cancer.Cell Cycle. 2006 Jun;5(11):1148-51. doi: 10.4161/cc.5.11.2798. Epub 2006 Jun 1. Cell Cycle. 2006. PMID: 16721055 Review.
-
Gene expression profiling and expanded immunohistochemistry tests to guide the use of adjuvant chemotherapy in breast cancer management: a systematic review and cost-effectiveness analysis.Health Technol Assess. 2013 Oct;17(44):1-302. doi: 10.3310/hta17440. Health Technol Assess. 2013. PMID: 24088296 Free PMC article. Review.
Cited by
-
Reproducibility and concordance of differential DNA methylation and gene expression in cancer.PLoS One. 2012;7(1):e29686. doi: 10.1371/journal.pone.0029686. Epub 2012 Jan 3. PLoS One. 2012. PMID: 22235325 Free PMC article.
-
Algebraic comparison of partial lists in bioinformatics.PLoS One. 2012;7(5):e36540. doi: 10.1371/journal.pone.0036540. Epub 2012 May 17. PLoS One. 2012. PMID: 22615778 Free PMC article.
-
scFed: federated learning for cell type classification with scRNA-seq.Brief Bioinform. 2023 Nov 22;25(1):bbad507. doi: 10.1093/bib/bbad507. Brief Bioinform. 2023. PMID: 38221903 Free PMC article.
-
All (remains) in the family? Using healthy relatives to define Crohn's gut microbiome alterations.Cell Rep Med. 2024 Jul 16;5(7):101651. doi: 10.1016/j.xcrm.2024.101651. Cell Rep Med. 2024. PMID: 39019007 Free PMC article.
-
GPDRP: a multimodal framework for drug response prediction with graph transformer.BMC Bioinformatics. 2023 Dec 17;24(1):484. doi: 10.1186/s12859-023-05618-0. BMC Bioinformatics. 2023. PMID: 38105227 Free PMC article.
References
-
- Early Breast Cancer Trialists’ Collaborative Group. Lancet. 1998;352:930–942. - PubMed
-
- Beer D. G., Kardia S. L., Huang C. C., Giordano T. J., Levin A. M., Misek D. E., Lin L., Chen G., Gharib T. G., Thomas D. G., et al. Nat. Med. 2002;8:816–824. - PubMed
-
- Rosenwald A., Wright G., Chan W. C., Connors J. M., Campo E., Fisher R. I., Gascoyne R. D., Muller-Hermelink H. K., Smeland E. B., Giltnane J. M., et al. N. Engl. J. Med. 2002;346:1937–1947. - PubMed
Publication types
MeSH terms
Substances
LinkOut - more resources
Full Text Sources
Other Literature Sources
Medical