Instance-based concept learning from multiclass DNA microarray data
- PMID: 16483361
- PMCID: PMC1402330
- DOI: 10.1186/1471-2105-7-73
Instance-based concept learning from multiclass DNA microarray data
Abstract
Background: Various statistical and machine learning methods have been successfully applied to the classification of DNA microarray data. Simple instance-based classifiers such as nearest neighbor (NN) approaches perform remarkably well in comparison to more complex models, and are currently experiencing a renaissance in the analysis of data sets from biology and biotechnology. While binary classification of microarray data has been extensively investigated, studies involving multiclass data are rare. The question remains open whether there exists a significant difference in performance between NN approaches and more complex multiclass methods. Comparative studies in this field commonly assess different models based on their classification accuracy only; however, this approach lacks the rigor needed to draw reliable conclusions and is inadequate for testing the null hypothesis of equal performance. Comparing novel classification models to existing approaches requires focusing on the significance of differences in performance.
Results: We investigated the performance of instance-based classifiers, including a NN classifier able to assign a degree of class membership to each sample. This model alleviates a major problem of conventional instance-based learners, namely the lack of confidence values for predictions. The model translates the distances to the nearest neighbors into 'confidence scores'; the higher the confidence score, the closer is the considered instance to a pre-defined class. We applied the models to three real gene expression data sets and compared them with state-of-the-art methods for classifying microarray data of multiple classes, assessing performance using a statistical significance test that took into account the data resampling strategy. Simple NN classifiers performed as well as, or significantly better than, their more intricate competitors.
Conclusion: Given its highly intuitive underlying principles--simplicity, ease-of-use, and robustness--the k-NN classifier complemented by a suitable distance-weighting regime constitutes an excellent alternative to more complex models for multiclass microarray data sets. Instance-based classifiers using weighted distances are not limited to microarray data sets, but are likely to perform competitively in classifications of high-dimensional biological data sets such as those generated by high-throughput mass spectrometry.
Figures
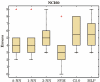
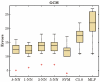
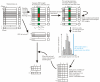
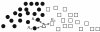
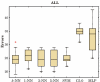
Similar articles
-
Kernel-imbedded Gaussian processes for disease classification using microarray gene expression data.BMC Bioinformatics. 2007 Feb 28;8:67. doi: 10.1186/1471-2105-8-67. BMC Bioinformatics. 2007. PMID: 17328811 Free PMC article.
-
Bias in error estimation when using cross-validation for model selection.BMC Bioinformatics. 2006 Feb 23;7:91. doi: 10.1186/1471-2105-7-91. BMC Bioinformatics. 2006. PMID: 16504092 Free PMC article.
-
Multiclass classification of microarray data samples with a reduced number of genes.BMC Bioinformatics. 2011 Feb 22;12:59. doi: 10.1186/1471-2105-12-59. BMC Bioinformatics. 2011. PMID: 21342522 Free PMC article.
-
Unsupervised pattern recognition: an introduction to the whys and wherefores of clustering microarray data.Brief Bioinform. 2005 Dec;6(4):331-43. doi: 10.1093/bib/6.4.331. Brief Bioinform. 2005. PMID: 16420732 Review.
-
Relative expression analysis for molecular cancer diagnosis and prognosis.Technol Cancer Res Treat. 2010 Apr;9(2):149-59. doi: 10.1177/153303461000900204. Technol Cancer Res Treat. 2010. PMID: 20218737 Free PMC article. Review.
Cited by
-
ANMM4CBR: a case-based reasoning method for gene expression data classification.Algorithms Mol Biol. 2010 Jan 6;5:14. doi: 10.1186/1748-7188-5-14. Algorithms Mol Biol. 2010. PMID: 20051140 Free PMC article.
-
A hybrid BPSO-CGA approach for gene selection and classification of microarray data.J Comput Biol. 2012 Jan;19(1):68-82. doi: 10.1089/cmb.2010.0064. Epub 2011 Jan 6. J Comput Biol. 2012. PMID: 21210743 Free PMC article.
-
Identifying Cancer Biomarkers From Microarray Data Using Feature Selection and Semisupervised Learning.IEEE J Transl Eng Health Med. 2014 Dec 2;2:4300211. doi: 10.1109/JTEHM.2014.2375820. eCollection 2014. IEEE J Transl Eng Health Med. 2014. PMID: 27170887 Free PMC article.
-
TACOA: taxonomic classification of environmental genomic fragments using a kernelized nearest neighbor approach.BMC Bioinformatics. 2009 Feb 11;10:56. doi: 10.1186/1471-2105-10-56. BMC Bioinformatics. 2009. PMID: 19210774 Free PMC article.
-
Use of yeast chemigenomics and COXEN informatics in preclinical evaluation of anticancer agents.Neoplasia. 2011 Jan;13(1):72-80. doi: 10.1593/neo.101214. Neoplasia. 2011. PMID: 21253455 Free PMC article.
References
-
- Ross DT, Scherf U, Eisen MB, Perou CM, Rees C, Spellman P, Iyer V, Jeffrey SS, van de Rijn M, Waltham M, Pergamenschikov A, Lee JC, Lashkari D, Shalon D, Myers TG, Weinstein JN, Botstein D, Brown PO. Systematic variation in gene expression patterns in human cancer cell lines. Nat Gen. 2000;24:227–235. - PubMed
-
- Yeoh EJ, Ross ME, Shurtleff SA, Williams WK, Patel D, Mahfouz R, Behm FG, Raimondi SC, Relling MV, Patel A, Cheng C, Campana D, Wilkins D, Zhou X, Li J, Liu H, Pui CH, Evans WE, Naeve C, Wong L, Downing JR. Classification, subtype discovery, and prediction of outcome in pediatric acute lymphoblastic leukemia by gene expression profiling. Cancer Cell. 2002;1:133–143. - PubMed
-
- Somorjai RL, Dolenko B, Baumgartner R. Class prediction and discovery using gene microarray and proteomics mass spectroscopy data: curses, caveats, cautions. Bioinformatics. 2003;19:1484–1491. - PubMed
-
- Dudoit S, Fridlyand J. Introduction to classification in microarray experiments. In: Berrar D, Dubitzky W, Granzow M, editor. A Practical Approach to Microarray Data Analysis. Boston: Kluwer Academic Publishers; 2002. pp. 131–151.
Publication types
MeSH terms
LinkOut - more resources
Full Text Sources