Investigations into resting-state connectivity using independent component analysis
- PMID: 16087444
- PMCID: PMC1854918
- DOI: 10.1098/rstb.2005.1634
Investigations into resting-state connectivity using independent component analysis
Abstract
Inferring resting-state connectivity patterns from functional magnetic resonance imaging (fMRI) data is a challenging task for any analytical technique. In this paper, we review a probabilistic independent component analysis (PICA) approach, optimized for the analysis of fMRI data, and discuss the role which this exploratory technique can take in scientific investigations into the structure of these effects. We apply PICA to fMRI data acquired at rest, in order to characterize the spatio-temporal structure of such data, and demonstrate that this is an effective and robust tool for the identification of low-frequency resting-state patterns from data acquired at various different spatial and temporal resolutions. We show that these networks exhibit high spatial consistency across subjects and closely resemble discrete cortical functional networks such as visual cortical areas or sensory-motor cortex.
Figures
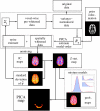
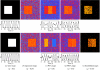
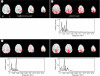
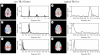
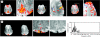
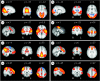
Similar articles
-
Resting state networks in empirical and simulated dynamic functional connectivity.Neuroimage. 2017 Oct 1;159:388-402. doi: 10.1016/j.neuroimage.2017.07.065. Epub 2017 Aug 3. Neuroimage. 2017. PMID: 28782678
-
Evaluation of spatio-temporal decomposition techniques for group analysis of fMRI resting state data sets.Neuroimage. 2014 Feb 15;87:363-82. doi: 10.1016/j.neuroimage.2013.10.062. Epub 2013 Nov 5. Neuroimage. 2014. PMID: 24201012
-
Resting network is composed of more than one neural pattern: an fMRI study.Neuroscience. 2014 Aug 22;274:198-208. doi: 10.1016/j.neuroscience.2014.05.035. Epub 2014 May 29. Neuroscience. 2014. PMID: 24881572
-
Resting state fMRI: A review on methods in resting state connectivity analysis and resting state networks.Neuroradiol J. 2017 Aug;30(4):305-317. doi: 10.1177/1971400917697342. Epub 2017 Mar 29. Neuroradiol J. 2017. PMID: 28353416 Free PMC article. Review.
-
Assessing functional connectivity in the human brain by fMRI.Magn Reson Imaging. 2007 Dec;25(10):1347-57. doi: 10.1016/j.mri.2007.03.007. Epub 2007 May 11. Magn Reson Imaging. 2007. PMID: 17499467 Free PMC article. Review.
Cited by
-
Mapping connectivity in the developing brain.Int J Dev Neurosci. 2013 Nov;31(7):525-42. doi: 10.1016/j.ijdevneu.2013.05.007. Epub 2013 May 27. Int J Dev Neurosci. 2013. PMID: 23722009 Free PMC article.
-
Wavelet-based regularity analysis reveals recurrent spatiotemporal behavior in resting-state fMRI.Hum Brain Mapp. 2015 Sep;36(9):3603-20. doi: 10.1002/hbm.22865. Epub 2015 Jun 12. Hum Brain Mapp. 2015. PMID: 26096080 Free PMC article.
-
Functional brain networks associated with eating behaviors in obesity.Sci Rep. 2016 Mar 31;6:23891. doi: 10.1038/srep23891. Sci Rep. 2016. PMID: 27030024 Free PMC article.
-
Connectivity-based structural and functional parcellation of the human cortex using diffusion imaging and tractography.Front Neuroanat. 2012 Aug 29;6:34. doi: 10.3389/fnana.2012.00034. eCollection 2012. Front Neuroanat. 2012. PMID: 22952459 Free PMC article.
-
Resting-state fMRI functional connectivity: a new perspective to evaluate pain modulation in migraine?Neurol Sci. 2015 May;36 Suppl 1:41-5. doi: 10.1007/s10072-015-2145-x. Neurol Sci. 2015. PMID: 26017510 Review.
References
-
- Alivisatos B, Milner B. Effects of frontal or temporal lobectomy on the use of advance information in a choice reaction task. Neuropsychologia. 1989;27:495–503. - PubMed
-
- Beckmann C, Smith S. Probabilistic independent component analysis for functional magnetic resonance imaging. IEEE Trans. Med. Imaging. 2004;23:137–152. - PubMed
-
- Beckmann C, Noble J, Smith S. Investigating the intrinsic dimensionality of fMRI data for ICA. Seventh International Conference on Functional Mapping of the Human Brain, Brighton, 10–14 June 2001. NeuroImage. 2001;13:S76.
-
- Beckmann C, Woolrich M, Smith S. Gaussian/Gamma mixture modelling of ICA/GLM spatial maps. Ninth International Conference on Functional Mapping of the Human Brain, New York, 18–22 June 2003. NeuroImage. 2003;19:S985.
-
- Behrens T, et al. Non-invasive mapping of connections between human thalamus and cortex using diffusion imaging. Nat. Neurosci. 2003;6:750–757. - PubMed
Publication types
MeSH terms
LinkOut - more resources
Full Text Sources
Other Literature Sources
Medical