-
Notifications
You must be signed in to change notification settings - Fork 1
Commit
This commit does not belong to any branch on this repository, and may belong to a fork outside of the repository.
- Loading branch information
0 parents
commit 8bc0fe1
Showing
95 changed files
with
220,066 additions
and
0 deletions.
There are no files selected for viewing
This file contains bidirectional Unicode text that may be interpreted or compiled differently than what appears below. To review, open the file in an editor that reveals hidden Unicode characters.
Learn more about bidirectional Unicode characters
Original file line number | Diff line number | Diff line change |
---|---|---|
@@ -0,0 +1,133 @@ | ||
# custom | ||
|
||
demo/ | ||
|
||
# Byte-compiled / optimized / DLL files | ||
__pycache__/ | ||
*.py[cod] | ||
*$py.class | ||
|
||
# C extensions | ||
*.so | ||
|
||
# Distribution / packaging | ||
.Python | ||
build/ | ||
develop-eggs/ | ||
dist/ | ||
downloads/ | ||
eggs/ | ||
.eggs/ | ||
lib/ | ||
lib64/ | ||
parts/ | ||
sdist/ | ||
var/ | ||
wheels/ | ||
pip-wheel-metadata/ | ||
share/python-wheels/ | ||
*.egg-info/ | ||
.installed.cfg | ||
*.egg | ||
MANIFEST | ||
|
||
# PyInstaller | ||
# Usually these files are written by a python script from a template | ||
# before PyInstaller builds the exe, so as to inject date/other infos into it. | ||
*.manifest | ||
*.spec | ||
|
||
# Installer logs | ||
pip-log.txt | ||
pip-delete-this-directory.txt | ||
|
||
# Unit test / coverage reports | ||
htmlcov/ | ||
.tox/ | ||
.nox/ | ||
.coverage | ||
.coverage.* | ||
.cache | ||
nosetests.xml | ||
coverage.xml | ||
*.cover | ||
*.py,cover | ||
.hypothesis/ | ||
.pytest_cache/ | ||
|
||
# Translations | ||
*.mo | ||
*.pot | ||
|
||
# Django stuff: | ||
*.log | ||
local_settings.py | ||
db.sqlite3 | ||
db.sqlite3-journal | ||
|
||
# Flask stuff: | ||
instance/ | ||
.webassets-cache | ||
|
||
# Scrapy stuff: | ||
.scrapy | ||
|
||
# Sphinx documentation | ||
docs/_build/ | ||
|
||
# PyBuilder | ||
target/ | ||
|
||
# Jupyter Notebook | ||
.ipynb_checkpoints | ||
|
||
# IPython | ||
profile_default/ | ||
ipython_config.py | ||
|
||
# pyenv | ||
.python-version | ||
|
||
# pipenv | ||
# According to pypa/pipenv#598, it is recommended to include Pipfile.lock in version control. | ||
# However, in case of collaboration, if having platform-specific dependencies or dependencies | ||
# having no cross-platform support, pipenv may install dependencies that don't work, or not | ||
# install all needed dependencies. | ||
#Pipfile.lock | ||
|
||
# PEP 582; used by e.g. github.com/David-OConnor/pyflow | ||
__pypackages__/ | ||
|
||
# Celery stuff | ||
celerybeat-schedule | ||
celerybeat.pid | ||
|
||
# SageMath parsed files | ||
*.sage.py | ||
|
||
# Environments | ||
.env | ||
.venv | ||
env/ | ||
venv/ | ||
ENV/ | ||
env.bak/ | ||
venv.bak/ | ||
|
||
# Spyder project settings | ||
.spyderproject | ||
.spyproject | ||
|
||
# Rope project settings | ||
.ropeproject | ||
|
||
# mkdocs documentation | ||
/site | ||
|
||
# mypy | ||
.mypy_cache/ | ||
.dmypy.json | ||
dmypy.json | ||
|
||
# Pyre type checker | ||
.pyre/ |
This file contains bidirectional Unicode text that may be interpreted or compiled differently than what appears below. To review, open the file in an editor that reveals hidden Unicode characters.
Learn more about bidirectional Unicode characters
Original file line number | Diff line number | Diff line change |
---|---|---|
@@ -0,0 +1,178 @@ | ||
Text To Speech System | ||
===== | ||
|
||
# Introduction | ||
|
||
In this repo, I focused on building a Multi-Speaker Text-to-Speech system :smile: In general, I used [Portaspeech](https://arxiv.org/abs/2109.15166) as an acoustic model and [iSTFTNet](https://arxiv.org/pdf/2203.02395.pdf) as vocoder... | ||
|
||
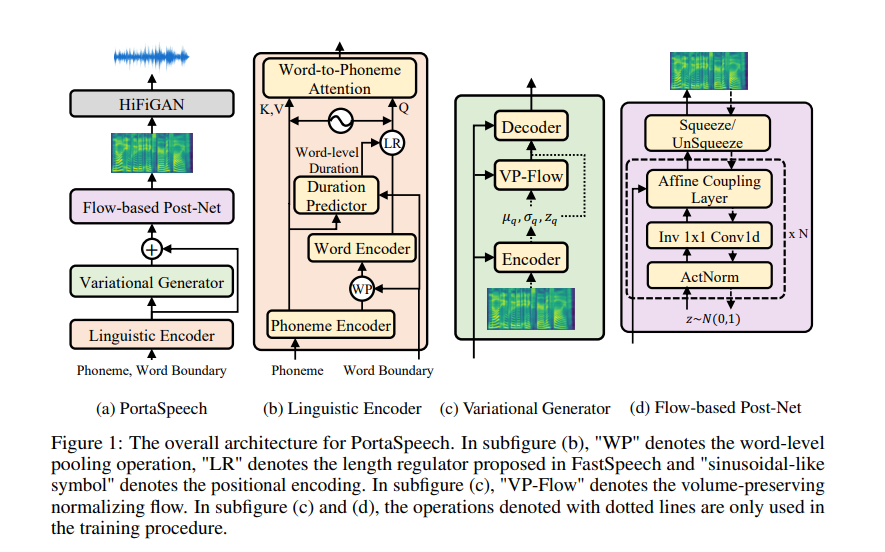 | ||
|
||
Portaspeech is one of the latest acoustic models (published in 2022) and it meets the needs of fast, lightweight, diverse, expressive and high-quality by utilizing some special attributes: Mixture alignment, VAE lightweight with enhanced prior as well as flow-based postnet. | ||
|
||
Mixture alignment is a big point of this paper, which uses both hard alignment (word level duration) and soft alignment (phoneme level duration) to eliminate the sensitivity of the normal alignment approach and also, an additional word-to-phoneme attention mechanism to capture more information about how relationship between a phoneme and a word. This mixture alignment module can be even applied to other TTS models. | ||
|
||
Both Variational Generator and Flow-based Postnet use the linguistic information from the previous block utilize the robustness of normalizing flow and enjoy its benefits during special training and inference strategy. Moreover, the author proposed a sharing parameter mechanism, which furthermore reduces the model parameters, besides the used VAE lightweight-based architecture. | ||
|
||
When it comes to vocoder, HiFIGan is quite a common choice, but I just wanna try one more vocoder named iSTFTNet. This reduces the computational cost from black-box modeling and avoids redundant estimations of high-dimensional spectrograms by substituting the inverse short-time Fourier transform (iSTFT) for some output-side layers of the mel-spectrogram vocoder after sufficiently reducing the frequency dimension using upsampling layers. Therefore, it's faster and more lightweight with a reasonable speech quality compared to HiFiGan. | ||
|
||
 | ||
|
||
# Setup | ||
|
||
## Environment | ||
|
||
You might want to clone the project and install some required packages in a virtual environment: | ||
|
||
```angular2html | ||
cd ViTTS | ||
conda create -n porta python=3.8 | ||
conda activate porta | ||
pip install -r requirements.txt | ||
pip install torch==1.8.0+cu111 torchvision==0.9.0+cu111 torchaudio==0.8.0 -f https://download.pytorch.org/whl/torch_stable.html # for cuda 11.1 | ||
``` | ||
|
||
## Data Preparation | ||
|
||
Assume you have a dataset named `Article`, which contains 2 speakers, each speaker should have wavs folder and a file that contains the scripts (metadata.csv). You need to place your data in folder `raw_data` as follow: | ||
|
||
```angular2html | ||
. | ||
├── configs | ||
│ ├── base | ||
│ │ └── Article | ||
│ └── small | ||
│ └── Article | ||
├── data | ||
│ ├── custom_data | ||
│ ├── lexicon | ||
│ │ ├── lexicon.dict | ||
│ ├── preprocessed_data | ||
│ │ ├── spker_embed | ||
│ │ └── TextGrid | ||
│ │ ├── speaker1 | ||
│ │ ├── speaker2 | ||
│ ├── raw_data | ||
│ │ ├── speaker1 | ||
│ │ │ └── wavs | ||
│ │ │ └── metadata.csv | ||
│ │ ├── speaker2 | ||
│ │ │ └── wavs | ||
│ │ │ └── metadata.csv | ||
├── demo | ||
├── output | ||
│ ├── base | ||
│ │ └── ckpt | ||
│ │ └── Article | ||
│ │ ├──143000.pth.tar | ||
│ └── small | ||
│ └── ckpt | ||
│ └── Article | ||
│ ├──65000.pth.tar | ||
├── src | ||
│ ├── models | ||
│ │ ├── vocoder | ||
│ │ │ ├── hifigan | ||
│ │ │ │ ├── generator_universal.pth.tar | ||
│ │ │ └── iSTFTNet | ||
│ │ │ ├── cp_hifigan | ||
│ │ │ ├── g_00090000 | ||
│ │ ├── portaspeech | ||
│ │ ├── deepspeech | ||
│ ├── evaluation | ||
│ │ └── MOSNet | ||
│ │ └── pre_trained | ||
│ │ ├── blstm.h5 | ||
│ │ ├── cnn.h5 | ||
│ │ ├── cnn_blstm.h5 | ||
└── tools | ||
``` | ||
|
||
## Alignments | ||
|
||
Note that, if you don't have alignments which are duration labels for the training process, you need to create them. Here I used [MFA](https://mfa-models.readthedocs.io/en/latest/) to do that. All file saved with `.TextGrid` tails should be placed like `data/preprocessed_data/TextGrid/*/*.TextGrid`. Then you should try these commands: | ||
|
||
```angular2html | ||
conda install -c conda-forge montreal-forced-aligner | ||
mfa train --config_path path_to_config/mfa_config.yml ./data/custom_data --clean ./data/lexicon/lexicon.dict ./data/preprocessed_data/TextGrid/ | ||
``` | ||
|
||
# Tools | ||
|
||
We should first have `lexicon.dict` in the folder `data/lexicon`. I actually implemented my own G2P tool here but note that is for Vietnamese. In case you use other languages, just use their corresponding g2p tool. To get the lexicon file (grapheme-phoneme dictionary), you should run: | ||
|
||
```angular2html | ||
python3 tools/phoneme_converter.py | ||
``` | ||
|
||
Next, custom data with: | ||
|
||
```angular2html | ||
python3 tools/prepare_alignment.py | ||
``` | ||
|
||
Following that, run following command to create speaker embeddings (using pretrained [DeepSpeaker](https://github.com/philipperemy/deep-speaker) model) and several important folders: | ||
|
||
```angular2html | ||
CUDA_VISIBLE_DEVICES=0 python3 tools/preprocess.py | ||
``` | ||
|
||
Next step, you might wanna train the vocoder, here I implemented both HiFiGan and iSTFTNet. To train iSTFTNet, just simply run: | ||
|
||
```angular2html | ||
CUDA_VISIBLE_DEVICES=0 python src/models/vocoder/iSTFTNet/train.py --config src/models/vocoder/iSTFTNet/config.json | ||
``` | ||
|
||
Now simply training acoustic model with: | ||
|
||
```angular2html | ||
CUDA_VISIBLE_DEVICES=0 python3 train.py --restore_step [] --model_type [small,base] # small or base version of portaspeech | ||
``` | ||
|
||
For inference, run: | ||
|
||
```angular2html | ||
CUDA_VISIBLE_DEVICES=0 python3 synthesize.py --restore_step [] --model_type [small,base] --mode [single,batch] --text [if single mode] --source path_to_txt_file_if_batch_mode | ||
``` | ||
|
||
One special note is that you can find necessary checkpoints of iSTFTNet, HiFiGAN and MOSNet, Deepspeech in their official repos and put them in suitable folders as above structure. Meanwhile, I just cannot provide you with the trained acoustic models hehe! Please train it yourself :) | ||
|
||
# Evaluation | ||
|
||
Here I used [MOSNet](https://github.com/lochenchou/MOSNet) to assess the generated speech. Of course this doesn't 100% reflect the speech quality but anyway it's a useful tool! Otherwise, to really get the most representative MOS scores (P-MOS and Q-MOS) for output speech, you might need a whole system including suitable groups of people following very strict rules, and ... to give feedback on them. Anyway, once you have all necessary trained models, you might wanna run: | ||
|
||
```angular2html | ||
cd src/evaluation/MOSNet/ | ||
python3 ./custom_test.py --rootdir path_to_folder_of_utterances | ||
``` | ||
|
||
# Demo | ||
|
||
You might wanna check another repo of mine [here](https://github.com/manhph2211/ml-deployment), which supports both frontend (React) and backend (torchserve) developments. It should look like this when you run the service: | ||
|
||
 | ||
|
||
# References | ||
|
||
```angular2html | ||
Lee, K. (2022). PortaSpeech (Version 0.2.0) [Computer software]. https://doi.org/10.5281/zenodo.5575261 | ||
``` | ||
|
||
```angular2html | ||
@inproceedings{mosnet, | ||
author={Lo, Chen-Chou and Fu, Szu-Wei and Huang, Wen-Chin and Wang, Xin and Yamagishi, Junichi and Tsao, Yu and Wang, Hsin-Min}, | ||
title={MOSNet: Deep Learning based Objective Assessment for Voice Conversion}, | ||
year=2019, | ||
booktitle={Proc. Interspeech 2019}, | ||
} | ||
``` | ||
|
||
```angular2html | ||
@inproceedings{kaneko2022istftnet, | ||
title={{iSTFTNet}: Fast and Lightweight Mel-Spectrogram Vocoder Incorporating Inverse Short-Time Fourier Transform}, | ||
author={Takuhiro Kaneko and Kou Tanaka and Hirokazu Kameoka and Shogo Seki}, | ||
booktitle={ICASSP}, | ||
year={2022}, | ||
} | ||
``` | ||
|
This file contains bidirectional Unicode text that may be interpreted or compiled differently than what appears below. To review, open the file in an editor that reveals hidden Unicode characters.
Learn more about bidirectional Unicode characters
Original file line number | Diff line number | Diff line change |
---|---|---|
@@ -0,0 +1,46 @@ | ||
external_speaker_dim: 512 | ||
|
||
transformer: | ||
encoder_layer: 4 | ||
encoder_head: 2 | ||
encoder_hidden: 192 | ||
conv_filter_size: 768 | ||
conv_kernel_size: 5 | ||
# encoder_dropout: 0.2 | ||
encoder_window_size: 4 | ||
|
||
variational_generator: | ||
encoder_layer: 8 | ||
decoder_layer: 4 | ||
conv_kernel_size: 5 | ||
conv_stride_size: 4 | ||
encoder_decoder_hidden: 192 | ||
# encoder_decoder_dropout: 0.2 | ||
latent_hidden: 16 | ||
vp_flow_layer: 4 | ||
vp_flow_hidden: 64 | ||
vp_flow_kernel: 3 | ||
|
||
postnet: | ||
wn_layer: 3 | ||
wn_kernel_size: 3 | ||
wn_hidden: 192 | ||
flow_step: 12 | ||
flow_dropout: 0.05 | ||
shared_group: 3 | ||
n_split: 4 | ||
n_sqz: 2 | ||
sigmoid_scale: False | ||
|
||
variance_predictor: | ||
filter_size: 192 | ||
kernel_size: 3 | ||
dropout: 0.5 | ||
|
||
multi_speaker: True | ||
|
||
max_seq_len: 1000 | ||
|
||
vocoder: | ||
model: "HiFi-GAN" # support 'HiFi-GAN', 'MelGAN' | ||
speaker: "universal" # support 'LJSpeech', 'universal' |
This file contains bidirectional Unicode text that may be interpreted or compiled differently than what appears below. To review, open the file in an editor that reveals hidden Unicode characters.
Learn more about bidirectional Unicode characters
Original file line number | Diff line number | Diff line change |
---|---|---|
@@ -0,0 +1,31 @@ | ||
dataset: "Article" | ||
|
||
path: | ||
corpus_path: "data/raw_data/" | ||
lexicon_path: "data/lexicon/lexicon.dict" | ||
raw_path: "data/custom_data" | ||
preprocessed_path: "data/preprocessed_data" | ||
|
||
preprocessing: | ||
speaker_embedder_cuda: True | ||
sort_data: True # sort data by mel frame length | ||
val_size: 512 | ||
text: | ||
text_cleaners: ["basic_cleaners"] | ||
language: "vi" | ||
sub_divide_word: True # subdivide long words into smaller pieces | ||
max_phoneme_num: 7 # threshold for subdivision | ||
audio: | ||
sampling_rate: 22050 | ||
max_wav_value: 32768.0 | ||
stft: | ||
filter_length: 1024 | ||
hop_length: 256 | ||
win_length: 1024 | ||
mel: | ||
n_mel_channels: 80 | ||
mel_fmin: 0 | ||
mel_fmax: 8000 # please set to 8000 for HiFi-GAN vocoder, set to null for MelGAN vocoder | ||
aligner: | ||
beta_binomial_scaling_factor: 1. | ||
speaker_embedder: "DeepSpeaker" |
Oops, something went wrong.