-
Notifications
You must be signed in to change notification settings - Fork 80
New issue
Have a question about this project? Sign up for a free GitHub account to open an issue and contact its maintainers and the community.
By clicking “Sign up for GitHub”, you agree to our terms of service and privacy statement. We’ll occasionally send you account related emails.
Already on GitHub? Sign in to your account
low accuracy at miniImagenet dataset? #1
Comments
Thanks for your review. You did nothing wrong. I am still looking for the reason of this behaviour. I have updated the code to support 5-shot learning with miniImagenet. But still I have low accuracy results with 1 and 5 shot in miniImagenet, with omniglot dataset it works fine. I will look into it as soon as possible. If you find any possible update to the code just let me know. |
You can change n_samplesNShot to 15, and change selected_class_meta_test in def creat_episodes to selected_classes, stay consistent with the superparam in meta learning lstm code, you will get the val and test accuracy about 55% with no fce, 5-ways, 5-shots in miniImagenet. |
Hi @ZUNJI |
Yes, you are right. This procedure indicates that the 'target class' is same as support class. You can read the paper<> and code. Our purpose of few-shot learning is recognising target images in novel classes based on support images in novel classes. The essence is computing the samilarities bewteen target images and support images and choose the most similar class of that support image as the predict target label. So this procedure is no problem. |
Certainly you should insure that the target images is not in support images. So I change n_samplesNShot to 15 then n_samples is 20. Using 5 appending in support set, the rest appending in target set. |
Sorry, it is not param 'n_samples', the param 'number_of_samples' in def create_episodes. |
The code randomly used 1 class of the support set as query label . So when we set the n_samplesNShot to 15, whether the number of classes in 'selected_class_meta_test" should be changed? |
First very thanks for your implement of Matching-Networks with pytorch.
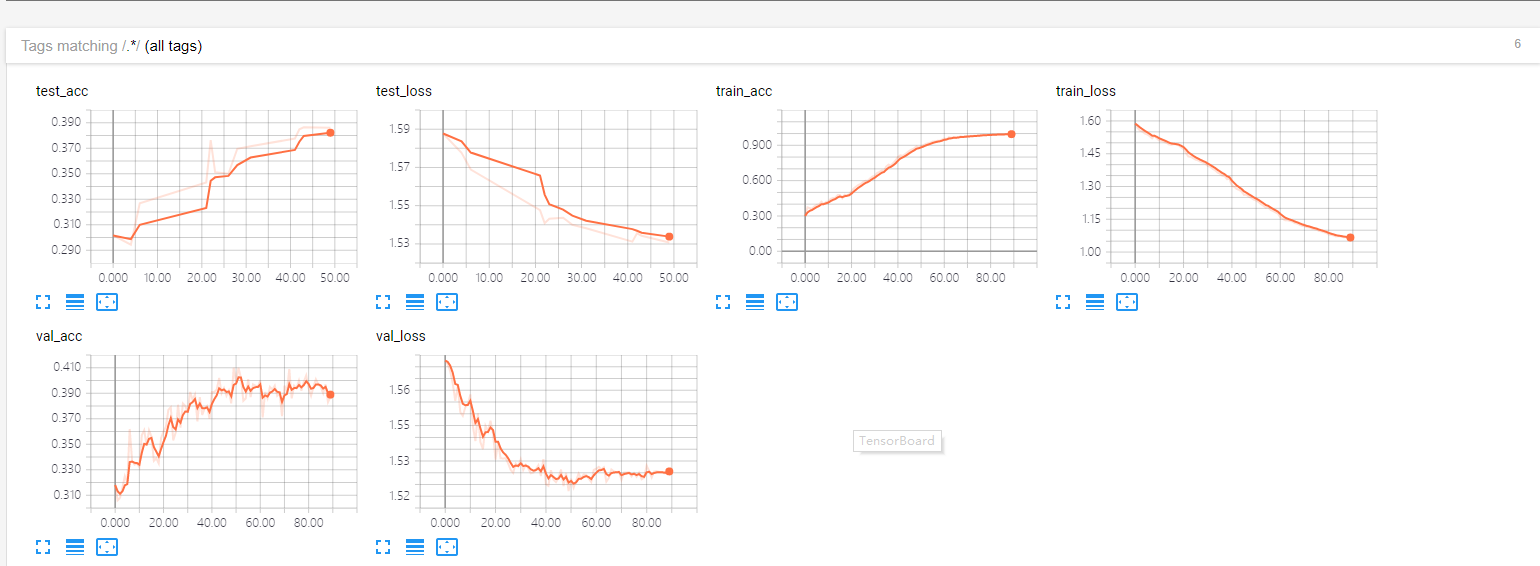
I have follow your setup to run the miniImagenet example,the training accuracy can achieve about 100%,but the val and test accuracy is about 40%.In origin paper it's about 57%.So I wonder if where I'm wrong to run your code or can you tell me your result at miniImagenet?
This is my logs
The text was updated successfully, but these errors were encountered: