Giotto: a toolbox for integrative analysis and visualization of spatial expression data
- PMID: 33685491
- PMCID: PMC7938609
- DOI: 10.1186/s13059-021-02286-2
Giotto: a toolbox for integrative analysis and visualization of spatial expression data
Abstract
Spatial transcriptomic and proteomic technologies have provided new opportunities to investigate cells in their native microenvironment. Here we present Giotto, a comprehensive and open-source toolbox for spatial data analysis and visualization. The analysis module provides end-to-end analysis by implementing a wide range of algorithms for characterizing tissue composition, spatial expression patterns, and cellular interactions. Furthermore, single-cell RNAseq data can be integrated for spatial cell-type enrichment analysis. The visualization module allows users to interactively visualize analysis outputs and imaging features. To demonstrate its general applicability, we apply Giotto to a wide range of datasets encompassing diverse technologies and platforms.
Conflict of interest statement
The authors declare that they have no competing interests.
Figures
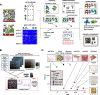
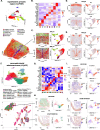
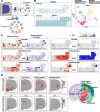
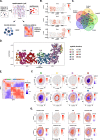
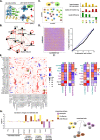
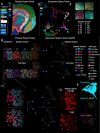
Similar articles
-
Analyzing Spatial Transcriptomics Data Using Giotto.Curr Protoc. 2022 Apr;2(4):e405. doi: 10.1002/cpz1.405. Curr Protoc. 2022. PMID: 35384407 Free PMC article.
-
Giotto Suite: a multi-scale and technology-agnostic spatial multi-omics analysis ecosystem.bioRxiv [Preprint]. 2023 Nov 27:2023.11.26.568752. doi: 10.1101/2023.11.26.568752. bioRxiv. 2023. PMID: 38077085 Free PMC article. Preprint.
-
Squidpy: a scalable framework for spatial omics analysis.Nat Methods. 2022 Feb;19(2):171-178. doi: 10.1038/s41592-021-01358-2. Epub 2022 Jan 31. Nat Methods. 2022. PMID: 35102346 Free PMC article.
-
Exploring tissue architecture using spatial transcriptomics.Nature. 2021 Aug;596(7871):211-220. doi: 10.1038/s41586-021-03634-9. Epub 2021 Aug 11. Nature. 2021. PMID: 34381231 Free PMC article. Review.
-
Mapping Gene Expression in the Spatial Dimension.Small Methods. 2021 Nov;5(11):e2100722. doi: 10.1002/smtd.202100722. Epub 2021 Sep 17. Small Methods. 2021. PMID: 34927963 Review.
Cited by
-
Highly Multiplexed Tissue Imaging in Precision Oncology and Translational Cancer Research.Cancer Discov. 2024 Nov 1;14(11):2071-2088. doi: 10.1158/2159-8290.CD-23-1165. Cancer Discov. 2024. PMID: 39485249 Free PMC article. Review.
-
Seq-Scope: repurposing Illumina sequencing flow cells for high-resolution spatial transcriptomics.Nat Protoc. 2024 Oct 31. doi: 10.1038/s41596-024-01065-0. Online ahead of print. Nat Protoc. 2024. PMID: 39482362 Review.
-
Detecting significant expression patterns in single-cell and spatial transcriptomics with a flexible computational approach.Sci Rep. 2024 Oct 30;14(1):26121. doi: 10.1038/s41598-024-75314-3. Sci Rep. 2024. PMID: 39478009 Free PMC article.
-
StereoSiTE: a framework to spatially and quantitatively profile the cellular neighborhood organized iTME.Gigascience. 2024 Jan 2;13:giae078. doi: 10.1093/gigascience/giae078. Gigascience. 2024. PMID: 39452614 Free PMC article.
-
A robust statistical approach for finding informative spatially associated pathways.Brief Bioinform. 2024 Sep 23;25(6):bbae543. doi: 10.1093/bib/bbae543. Brief Bioinform. 2024. PMID: 39451157 Free PMC article.
References
Publication types
MeSH terms
Associated data
Grants and funding
LinkOut - more resources
Full Text Sources
Other Literature Sources