Breath Metabolomics Provides an Accurate and Noninvasive Approach for Screening Cirrhosis, Primary, and Secondary Liver Tumors
- PMID: 32626836
- PMCID: PMC7327218
- DOI: 10.1002/hep4.1499
Breath Metabolomics Provides an Accurate and Noninvasive Approach for Screening Cirrhosis, Primary, and Secondary Liver Tumors
Abstract
Hepatocellular carcinoma (HCC) and secondary liver tumors, such as colorectal cancer liver metastases are significant contributors to the overall burden of cancer-related morality. Current biomarkers, such as alpha-fetoprotein (AFP) for HCC, result in too many false negatives, necessitating noninvasive approaches with improved sensitivity. Volatile organic compounds (VOCs) detected in the breath of patients can provide valuable insight into disease processes and can differentiate patients by disease status. Here, we investigate whether 22 VOCs from the breath of 296 patients can distinguish those with no liver disease (n = 54), cirrhosis (n = 30), HCC (n = 112), pulmonary hypertension (n = 49), or colorectal cancer liver metastases (n = 51). This work extends previous studies by evaluating the ability for VOC signatures to differentiate multiple diseases in a large cohort of patients. Pairwise disease comparisons demonstrated that most of the VOCs tested are present in significantly different relative abundances (false discovery rate P < 0.1), indicating broad impacts on the breath metabolome across diseases. A predictive model developed using random forest machine learning and cross validation classified patients with 85% classification accuracy and 75% balanced accuracy. Importantly, the model detected HCC with 73% sensitivity compared with 53% for AFP in the same cohort. An added value of this approach is that influential VOCs in the predictive model may provide insight into disease etiology. Acetaldehyde and acetone, both of which have roles in tumor promotion, were considered important VOCs for differentiating disease groups in the predictive model and were increased in patients with cirrhosis and HCC compared to patients with no liver disease (false discovery rate P < 0.1). Conclusion: The use of machine learning and breath VOCs shows promise as an approach to develop improved, noninvasive screening tools for chronic liver disease and primary and secondary liver tumors.
© 2020 The Authors. Hepatology Communications published by Wiley Periodicals, Inc., on behalf of the American Association for the Study of Liver Diseases.
Figures
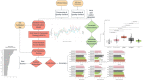
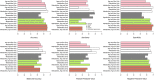
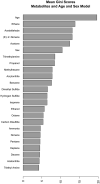
Similar articles
-
Comparison of Two Modern Survival Prediction Tools, SORG-MLA and METSSS, in Patients With Symptomatic Long-bone Metastases Who Underwent Local Treatment With Surgery Followed by Radiotherapy and With Radiotherapy Alone.Clin Orthop Relat Res. 2024 Dec 1;482(12):2193-2208. doi: 10.1097/CORR.0000000000003185. Epub 2024 Jul 23. Clin Orthop Relat Res. 2024. PMID: 39051924
-
Defining the optimum strategy for identifying adults and children with coeliac disease: systematic review and economic modelling.Health Technol Assess. 2022 Oct;26(44):1-310. doi: 10.3310/ZUCE8371. Health Technol Assess. 2022. PMID: 36321689 Free PMC article.
-
Depressing time: Waiting, melancholia, and the psychoanalytic practice of care.In: Kirtsoglou E, Simpson B, editors. The Time of Anthropology: Studies of Contemporary Chronopolitics. Abingdon: Routledge; 2020. Chapter 5. In: Kirtsoglou E, Simpson B, editors. The Time of Anthropology: Studies of Contemporary Chronopolitics. Abingdon: Routledge; 2020. Chapter 5. PMID: 36137063 Free Books & Documents. Review.
-
The effectiveness of school-based family asthma educational programs on the quality of life and number of asthma exacerbations of children aged five to 18 years diagnosed with asthma: a systematic review protocol.JBI Database System Rev Implement Rep. 2015 Oct;13(10):69-81. doi: 10.11124/jbisrir-2015-2335. JBI Database System Rev Implement Rep. 2015. PMID: 26571284
-
Impact of residual disease as a prognostic factor for survival in women with advanced epithelial ovarian cancer after primary surgery.Cochrane Database Syst Rev. 2022 Sep 26;9(9):CD015048. doi: 10.1002/14651858.CD015048.pub2. Cochrane Database Syst Rev. 2022. PMID: 36161421 Free PMC article. Review.
Cited by
-
Liver Impairment-The Potential Application of Volatile Organic Compounds in Hepatology.Metabolites. 2021 Sep 11;11(9):618. doi: 10.3390/metabo11090618. Metabolites. 2021. PMID: 34564434 Free PMC article. Review.
-
Advancing Colorectal Cancer Diagnosis with AI-Powered Breathomics: Navigating Challenges and Future Directions.Diagnostics (Basel). 2023 Dec 15;13(24):3673. doi: 10.3390/diagnostics13243673. Diagnostics (Basel). 2023. PMID: 38132257 Free PMC article. Review.
-
Sample Collection and Processing in Volatile Organic Compound Analysis for Gastrointestinal Cancers.Diagnostics (Basel). 2024 Jul 19;14(14):1563. doi: 10.3390/diagnostics14141563. Diagnostics (Basel). 2024. PMID: 39061700 Free PMC article. Review.
-
Diagnosis of Carcinogenic Pathologies through Breath Biomarkers: Present and Future Trends.Biomedicines. 2023 Nov 11;11(11):3029. doi: 10.3390/biomedicines11113029. Biomedicines. 2023. PMID: 38002028 Free PMC article. Review.
-
Artificial intelligence in the diagnosis and management of colorectal cancer liver metastases.World J Gastroenterol. 2022 Jan 7;28(1):108-122. doi: 10.3748/wjg.v28.i1.108. World J Gastroenterol. 2022. PMID: 35125822 Free PMC article. Review.
References
-
- Siegel RL, Miller KD, Jemal A. Cancer statistics, 2019. CA Cancer J Clin 2019;69:7‐34. - PubMed
-
- Debruyne EN, Delanghe JR. Diagnosing and monitoring hepatocellular carcinoma with alpha‐fetoprotein: new aspects and applications. Clin Chim Acta 2008;395:19‐26. - PubMed
-
- Wang B, Chen D, Chen Y, Hu Z, Cao M, Xie Q, et al. Metabonomic profiles discriminate hepatocellular carcinoma from liver cirrhosis by ultraperformance liquid chromatography‐mass spectrometry. J Proteome Res 2012;11:1217‐1227. - PubMed
Grants and funding
LinkOut - more resources
Full Text Sources
Other Literature Sources