Changes in contact patterns shape the dynamics of the COVID-19 outbreak in China
- PMID: 32350060
- PMCID: PMC7199529
- DOI: 10.1126/science.abb8001
Changes in contact patterns shape the dynamics of the COVID-19 outbreak in China
Abstract
Intense nonpharmaceutical interventions were put in place in China to stop transmission of the novel coronavirus disease 2019 (COVID-19). As transmission intensifies in other countries, the interplay between age, contact patterns, social distancing, susceptibility to infection, and COVID-19 dynamics remains unclear. To answer these questions, we analyze contact survey data for Wuhan and Shanghai before and during the outbreak and contact-tracing information from Hunan province. Daily contacts were reduced seven- to eightfold during the COVID-19 social distancing period, with most interactions restricted to the household. We find that children 0 to 14 years of age are less susceptible to severe acute respiratory syndrome coronavirus 2 (SARS-CoV-2) infection than adults 15 to 64 years of age (odds ratio 0.34, 95% confidence interval 0.24 to 0.49), whereas individuals more than 65 years of age are more susceptible to infection (odds ratio 1.47, 95% confidence interval 1.12 to 1.92). Based on these data, we built a transmission model to study the impact of social distancing and school closure on transmission. We find that social distancing alone, as implemented in China during the outbreak, is sufficient to control COVID-19. Although proactive school closures cannot interrupt transmission on their own, they can reduce peak incidence by 40 to 60% and delay the epidemic.
Copyright © 2020 The Authors, some rights reserved; exclusive licensee American Association for the Advancement of Science. No claim to original U.S. Government Works.
Figures
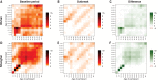
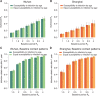
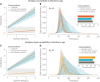
Similar articles
-
Determining the optimal strategy for reopening schools, the impact of test and trace interventions, and the risk of occurrence of a second COVID-19 epidemic wave in the UK: a modelling study.Lancet Child Adolesc Health. 2020 Nov;4(11):817-827. doi: 10.1016/S2352-4642(20)30250-9. Epub 2020 Aug 3. Lancet Child Adolesc Health. 2020. PMID: 32758453 Free PMC article.
-
Epidemiology and transmission of COVID-19 in 391 cases and 1286 of their close contacts in Shenzhen, China: a retrospective cohort study.Lancet Infect Dis. 2020 Aug;20(8):911-919. doi: 10.1016/S1473-3099(20)30287-5. Epub 2020 Apr 27. Lancet Infect Dis. 2020. PMID: 32353347 Free PMC article.
-
Evaluating the Effectiveness of Social Distancing Interventions to Delay or Flatten the Epidemic Curve of Coronavirus Disease.Emerg Infect Dis. 2020 Aug;26(8):1740-1748. doi: 10.3201/eid2608.201093. Epub 2020 Apr 28. Emerg Infect Dis. 2020. PMID: 32343222 Free PMC article.
-
[The outbreak of COVID-19 in China].Internist (Berl). 2020 Aug;61(8):776-781. doi: 10.1007/s00108-020-00833-w. Internist (Berl). 2020. PMID: 32548651 Free PMC article. Review. German.
-
COVID-19 in South Korea.Postgrad Med J. 2020 Jul;96(1137):399-402. doi: 10.1136/postgradmedj-2020-137738. Epub 2020 May 4. Postgrad Med J. 2020. PMID: 32366457 Free PMC article. Review.
Cited by
-
Association of Sleep Duration and Screen Time With Anxiety of Pregnant Women During the COVID-19 Pandemic.Front Psychol. 2021 Apr 20;12:646368. doi: 10.3389/fpsyg.2021.646368. eCollection 2021. Front Psychol. 2021. PMID: 33959075 Free PMC article.
-
Digital proximity tracing on empirical contact networks for pandemic control.Nat Commun. 2021 Mar 12;12(1):1655. doi: 10.1038/s41467-021-21809-w. Nat Commun. 2021. PMID: 33712583 Free PMC article.
-
Transmission roles of symptomatic and asymptomatic COVID-19 cases: a modelling study.Epidemiol Infect. 2022 Sep 27;150:e171. doi: 10.1017/S0950268822001467. Epidemiol Infect. 2022. PMID: 36263615 Free PMC article.
-
Modelling the impact of testing, contact tracing and household quarantine on second waves of COVID-19.Nat Hum Behav. 2020 Sep;4(9):964-971. doi: 10.1038/s41562-020-0931-9. Epub 2020 Aug 5. Nat Hum Behav. 2020. PMID: 32759985 Free PMC article.
-
How the global health security index and environment factor influence the spread of COVID-19: A country level analysis.One Health. 2021 Jun;12:100235. doi: 10.1016/j.onehlt.2021.100235. Epub 2021 Mar 10. One Health. 2021. PMID: 33723518 Free PMC article.
References
-
- Johns Hopkins University, COVID-19 Dashboard (2020); https://coronavirus.jhu.edu/map.html [accessed 16 April 2020].
-
- Chinese Center for Disease Control and Prevention, Update on COVID-19 as of 24:00 on April 16, 2020 (2020); http://2019ncov.chinacdc.cn/2019-nCoV/ [accessed 17 April 2020].
-
- Zhang J., Litvinova M., Wang W., Wang Y., Deng X., Chen X., Li M., Zheng W., Yi L., Chen X., Wu Q., Liang Y., Wang X., Yang J., Sun K., Longini I. M. Jr.., Halloran M. E., Wu P., Cowling B. J., Merler S., Viboud C., Vespignani A., Ajelli M., Yu H., Evolving epidemiology and transmission dynamics of coronavirus disease 2019 outside Hubei province, China: A descriptive and modelling study. Lancet Infect. Dis. S1473-3099(20)30230-9 (2020). 10.1016/S1473-3099(20)30230-9 - DOI - PMC - PubMed
-
- Mossong J., Hens N., Jit M., Beutels P., Auranen K., Mikolajczyk R., Massari M., Salmaso S., Tomba G. S., Wallinga J., Heijne J., Sadkowska-Todys M., Rosinska M., Edmunds W. J., Social contacts and mixing patterns relevant to the spread of infectious diseases. PLOS Med. 5, e74 (2008). 10.1371/journal.pmed.0050074 - DOI - PMC - PubMed
Publication types
MeSH terms
LinkOut - more resources
Full Text Sources
Research Materials
Miscellaneous