Computer-aided diagnosis of lung nodule classification between benign nodule, primary lung cancer, and metastatic lung cancer at different image size using deep convolutional neural network with transfer learning
- PMID: 30052644
- PMCID: PMC6063408
- DOI: 10.1371/journal.pone.0200721
Computer-aided diagnosis of lung nodule classification between benign nodule, primary lung cancer, and metastatic lung cancer at different image size using deep convolutional neural network with transfer learning
Abstract
We developed a computer-aided diagnosis (CADx) method for classification between benign nodule, primary lung cancer, and metastatic lung cancer and evaluated the following: (i) the usefulness of the deep convolutional neural network (DCNN) for CADx of the ternary classification, compared with a conventional method (hand-crafted imaging feature plus machine learning), (ii) the effectiveness of transfer learning, and (iii) the effect of image size as the DCNN input. Among 1240 patients of previously-built database, computed tomography images and clinical information of 1236 patients were included. For the conventional method, CADx was performed by using rotation-invariant uniform-pattern local binary pattern on three orthogonal planes with a support vector machine. For the DCNN method, CADx was evaluated using the VGG-16 convolutional neural network with and without transfer learning, and hyperparameter optimization of the DCNN method was performed by random search. The best averaged validation accuracies of CADx were 55.9%, 68.0%, and 62.4% for the conventional method, the DCNN method with transfer learning, and the DCNN method without transfer learning, respectively. For image size of 56, 112, and 224, the best averaged validation accuracy for the DCNN with transfer learning were 60.7%, 64.7%, and 68.0%, respectively. DCNN was better than the conventional method for CADx, and the accuracy of DCNN improved when using transfer learning. Also, we found that larger image sizes as inputs to DCNN improved the accuracy of lung nodule classification.
Conflict of interest statement
The authors have declared that no competing interests exist.
Figures
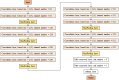
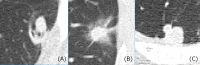
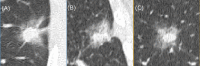
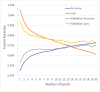
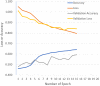
Similar articles
-
Computer-aided diagnosis of lung nodule using gradient tree boosting and Bayesian optimization.PLoS One. 2018 Apr 19;13(4):e0195875. doi: 10.1371/journal.pone.0195875. eCollection 2018. PLoS One. 2018. PMID: 29672639 Free PMC article.
-
Automatic feature learning using multichannel ROI based on deep structured algorithms for computerized lung cancer diagnosis.Comput Biol Med. 2017 Oct 1;89:530-539. doi: 10.1016/j.compbiomed.2017.04.006. Epub 2017 Apr 13. Comput Biol Med. 2017. PMID: 28473055
-
Digital breast tomosynthesis versus digital mammography: integration of image modalities enhances deep learning-based breast mass classification.Eur Radiol. 2020 Feb;30(2):778-788. doi: 10.1007/s00330-019-06457-5. Epub 2019 Nov 5. Eur Radiol. 2020. PMID: 31691121
-
Overview of Computer Aided Detection and Computer Aided Diagnosis Systems for Lung Nodule Detection in Computed Tomography.Curr Med Imaging Rev. 2020;16(1):16-26. doi: 10.2174/1573405615666190206153321. Curr Med Imaging Rev. 2020. PMID: 31989890 Review.
-
Lung nodule diagnosis and cancer histology classification from computed tomography data by convolutional neural networks: A survey.Comput Biol Med. 2022 Jul;146:105691. doi: 10.1016/j.compbiomed.2022.105691. Epub 2022 Jun 6. Comput Biol Med. 2022. PMID: 35691714 Review.
Cited by
-
Convolutional Neural Network ensembles for accurate lung nodule malignancy prediction 2 years in the future.Comput Biol Med. 2020 Jul;122:103882. doi: 10.1016/j.compbiomed.2020.103882. Epub 2020 Jun 24. Comput Biol Med. 2020. PMID: 32658721 Free PMC article.
-
Role of Ensemble Deep Learning for Brain Tumor Classification in Multiple Magnetic Resonance Imaging Sequence Data.Diagnostics (Basel). 2023 Jan 28;13(3):481. doi: 10.3390/diagnostics13030481. Diagnostics (Basel). 2023. PMID: 36766587 Free PMC article.
-
A Survey of Computer-Aided Tumor Diagnosis Based on Convolutional Neural Network.Biology (Basel). 2021 Oct 22;10(11):1084. doi: 10.3390/biology10111084. Biology (Basel). 2021. PMID: 34827077 Free PMC article. Review.
-
Automated Identification of Skull Fractures With Deep Learning: A Comparison Between Object Detection and Segmentation Approach.Front Neurol. 2021 Oct 29;12:687931. doi: 10.3389/fneur.2021.687931. eCollection 2021. Front Neurol. 2021. PMID: 34777193 Free PMC article.
-
Identification of Gastritis Subtypes by Convolutional Neuronal Networks on Histological Images of Antrum and Corpus Biopsies.Int J Mol Sci. 2020 Sep 11;21(18):6652. doi: 10.3390/ijms21186652. Int J Mol Sci. 2020. PMID: 32932860 Free PMC article.
References
-
- Suzuki K. Computer-Aided Detection of Lung Cancer In: Image-Based Computer-Assisted Radiation Therapy. Singapore: Springer; Singapore; 2017:9–40. 10.1007/978-981-10-2945-5_2 - DOI
-
- Kawagishi M, Chen B, Furukawa D, Sekiguchi H, Sakai K, Kubo T, et al. A study of computer-aided diagnosis for pulmonary nodule: comparison between classification accuracies using calculated image features and imaging findings annotated by radiologists. Int J Comput Assist Radiol Surg. 2017;12(5):767–776. 10.1007/s11548-017-1554-0 - DOI - PubMed
-
- de Oseas Carvalho Filho A, Corrêa Silva A, de Cardoso Paiva A, Acatauas Nunes R, Gattass M, Acatauassú Nunes R. Computer-Aided Diagnosis of Lung Nodules in Computed Tomography by Using Phylogenetic Diversity, Genetic Algorithm, and SVM. J Digit Imaging. 2017. 10.1007/s10278-017-9973-6 - DOI - PMC - PubMed
Publication types
MeSH terms
Grants and funding
LinkOut - more resources
Full Text Sources
Other Literature Sources
Medical