Bayesian Nonparametric Ordination for the Analysis of Microbial Communities
- PMID: 29430070
- PMCID: PMC5804367
- DOI: 10.1080/01621459.2017.1288631
Bayesian Nonparametric Ordination for the Analysis of Microbial Communities
Abstract
Human microbiome studies use sequencing technologies to measure the abundance of bacterial species or Operational Taxonomic Units (OTUs) in samples of biological material. Typically the data are organized in contingency tables with OTU counts across heterogeneous biological samples. In the microbial ecology community, ordination methods are frequently used to investigate latent factors or clusters that capture and describe variations of OTU counts across biological samples. It remains important to evaluate how uncertainty in estimates of each biological sample's microbial distribution propagates to ordination analyses, including visualization of clusters and projections of biological samples on low dimensional spaces. We propose a Bayesian analysis for dependent distributions to endow frequently used ordinations with estimates of uncertainty. A Bayesian nonparametric prior for dependent normalized random measures is constructed, which is marginally equivalent to the normalized generalized Gamma process, a well-known prior for nonparametric analyses. In our prior, the dependence and similarity between microbial distributions is represented by latent factors that concentrate in a low dimensional space. We use a shrinkage prior to tune the dimensionality of the latent factors. The resulting posterior samples of model parameters can be used to evaluate uncertainty in analyses routinely applied in microbiome studies. Specifically, by combining them with multivariate data analysis techniques we can visualize credible regions in ecological ordination plots. The characteristics of the proposed model are illustrated through a simulation study and applications in two microbiome datasets.
Keywords: Bayesian factor analysis; Dependent Dirichlet processes; Microbiome data analysis; Uncertainty of ordination.
Figures
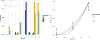
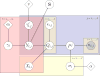
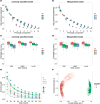
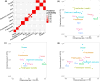
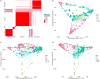
Similar articles
-
Evaluating and presenting uncertainty in model-based unconstrained ordination.Ecol Evol. 2019 Dec 20;10(1):59-69. doi: 10.1002/ece3.5752. eCollection 2020 Jan. Ecol Evol. 2019. PMID: 31988716 Free PMC article.
-
A Bayesian Semiparametric Regression Model for Joint Analysis of Microbiome Data.Front Microbiol. 2018 Mar 26;9:522. doi: 10.3389/fmicb.2018.00522. eCollection 2018. Front Microbiol. 2018. PMID: 29632519 Free PMC article.
-
Comparison of distance-based and model-based ordinations.Ecology. 2020 Jan;101(1):e02908. doi: 10.1002/ecy.2908. Epub 2019 Nov 6. Ecology. 2020. PMID: 31602634
-
A Bayesian nonparametric analysis for zero-inflated multivariate count data with application to microbiome study.J R Stat Soc Ser C Appl Stat. 2021 Aug;70(4):961-979. doi: 10.1111/rssc.12493. Epub 2021 Aug 7. J R Stat Soc Ser C Appl Stat. 2021. PMID: 37440868 Free PMC article.
-
Applications and Comparison of Dimensionality Reduction Methods for Microbiome Data.Front Bioinform. 2022 Feb 24;2:821861. doi: 10.3389/fbinf.2022.821861. eCollection 2022. Front Bioinform. 2022. PMID: 36304280 Free PMC article. Review.
Cited by
-
Successful strategies for human microbiome data generation, storage and analyses.J Biosci. 2019 Oct;44(5):111. J Biosci. 2019. PMID: 31719220 Review.
-
Estimating diversity in networked ecological communities.Biostatistics. 2022 Jan 13;23(1):207-222. doi: 10.1093/biostatistics/kxaa015. Biostatistics. 2022. PMID: 32432696 Free PMC article.
-
A Bioconductor workflow for the Bayesian analysis of spatial proteomics.F1000Res. 2019 Apr 11;8:446. doi: 10.12688/f1000research.18636.1. eCollection 2019. F1000Res. 2019. PMID: 31119032 Free PMC article.
-
A Primer for Microbiome Time-Series Analysis.Front Genet. 2020 Apr 21;11:310. doi: 10.3389/fgene.2020.00310. eCollection 2020. Front Genet. 2020. PMID: 32373155 Free PMC article.
-
Bayesian biclustering for microbial metagenomic sequencing data via multinomial matrix factorization.Biostatistics. 2022 Jul 18;23(3):891-909. doi: 10.1093/biostatistics/kxab002. Biostatistics. 2022. PMID: 33634824 Free PMC article.
References
-
- Abdi H, O’Toole AJ, Valentin D, Edelman B. Computer Vision and Pattern Recognition-Workshops, 2005 CVPR Workshops IEEE Computer Society Conference on. IEEE; 2005. Distatis: The analysis of multiple distance matrices; pp. 42–42.
-
- Anderson MJ, Ellingsen KE, McArdle BH. Multivariate dispersion as a measure of beta diversity. Ecology Letters. 2006;9(6):683–693. - PubMed
-
- Ando T. Bayesian factor analysis with fat-tailed factors and its exact marginal likelihood. Journal of Multivariate Analysis. 2009;100(8):1717–1726.
-
- Brix A. Generalized gamma measures and shot-noise cox processes. Advances in Applied Probability. 1999:929–953.
Publication types
Grants and funding
LinkOut - more resources
Full Text Sources
Other Literature Sources