A Mass Spectrometry-Based Approach for Mapping Protein Subcellular Localization Reveals the Spatial Proteome of Mouse Primary Neurons
- PMID: 28903049
- PMCID: PMC5775508
- DOI: 10.1016/j.celrep.2017.08.063
A Mass Spectrometry-Based Approach for Mapping Protein Subcellular Localization Reveals the Spatial Proteome of Mouse Primary Neurons
Abstract
We previously developed a mass spectrometry-based method, dynamic organellar maps, for the determination of protein subcellular localization and identification of translocation events in comparative experiments. The use of metabolic labeling for quantification (stable isotope labeling by amino acids in cell culture [SILAC]) renders the method best suited to cells grown in culture. Here, we have adapted the workflow to both label-free quantification (LFQ) and chemical labeling/multiplexing strategies (tandem mass tagging [TMT]). Both methods are highly effective for the generation of organellar maps and capture of protein translocations. Furthermore, application of label-free organellar mapping to acutely isolated mouse primary neurons provided subcellular localization and copy-number information for over 8,000 proteins, allowing a detailed analysis of organellar organization. Our study extends the scope of dynamic organellar maps to any cell type or tissue and also to high-throughput screening.
Keywords: EGF signaling; LFQ; TMT; label-free quantification; neurons; organellar proteomics; primary cells; quantitative mass spectrometry; spatial proteomics; tandem mass tagging.
Copyright © 2017 The Author(s). Published by Elsevier Inc. All rights reserved.
Figures
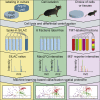
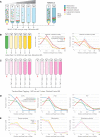
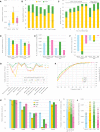
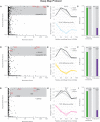
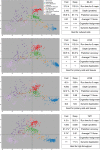
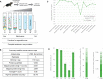
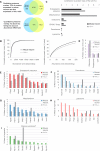
Similar articles
-
Organellar Maps Through Proteomic Profiling - A Conceptual Guide.Mol Cell Proteomics. 2020 Jul;19(7):1076-1087. doi: 10.1074/mcp.R120.001971. Epub 2020 Apr 28. Mol Cell Proteomics. 2020. PMID: 32345598 Free PMC article. Review.
-
Proteomics methods for subcellular proteome analysis.FEBS J. 2013 Nov;280(22):5626-34. doi: 10.1111/febs.12502. Epub 2013 Sep 20. FEBS J. 2013. PMID: 24034475 Review.
-
Dynamic Organellar Maps for Spatial Proteomics.Curr Protoc Cell Biol. 2019 Jun;83(1):e81. doi: 10.1002/cpcb.81. Epub 2018 Nov 29. Curr Protoc Cell Biol. 2019. PMID: 30489039
-
Systematic comparison of label-free, metabolic labeling, and isobaric chemical labeling for quantitative proteomics on LTQ Orbitrap Velos.J Proteome Res. 2012 Mar 2;11(3):1582-90. doi: 10.1021/pr200748h. Epub 2012 Feb 16. J Proteome Res. 2012. PMID: 22188275
-
Global, quantitative and dynamic mapping of protein subcellular localization.Elife. 2016 Jun 9;5:e16950. doi: 10.7554/eLife.16950. Elife. 2016. PMID: 27278775 Free PMC article.
Cited by
-
Spatial proteomics reveals subcellular reorganization in human keratinocytes exposed to UVA light.iScience. 2022 Mar 16;25(4):104093. doi: 10.1016/j.isci.2022.104093. eCollection 2022 Apr 15. iScience. 2022. PMID: 35372811 Free PMC article.
-
Assessing sub-cellular resolution in spatial proteomics experiments.Curr Opin Chem Biol. 2019 Feb;48:123-149. doi: 10.1016/j.cbpa.2018.11.015. Epub 2018 Dec 14. Curr Opin Chem Biol. 2019. PMID: 30711721 Free PMC article. Review.
-
Comparative Analysis of T-Cell Spatial Proteomics and the Influence of HIV Expression.Mol Cell Proteomics. 2022 Mar;21(3):100194. doi: 10.1016/j.mcpro.2022.100194. Epub 2022 Jan 8. Mol Cell Proteomics. 2022. PMID: 35017099 Free PMC article.
-
Cargo Sorting at the trans-Golgi Network for Shunting into Specific Transport Routes: Role of Arf Small G Proteins and Adaptor Complexes.Cells. 2019 Jun 3;8(6):531. doi: 10.3390/cells8060531. Cells. 2019. PMID: 31163688 Free PMC article. Review.
-
SPIDER: constructing cell-type-specific protein-protein interaction networks.Bioinform Adv. 2024 Aug 30;4(1):vbae130. doi: 10.1093/bioadv/vbae130. eCollection 2024. Bioinform Adv. 2024. PMID: 39346952 Free PMC article.
References
-
- Aebersold R., Mann M. Mass-spectrometric exploration of proteome structure and function. Nature. 2016;537:347–355. - PubMed
-
- Cox J., Mann M. MaxQuant enables high peptide identification rates, individualized p.p.b.-range mass accuracies and proteome-wide protein quantification. Nat. Biotechnol. 2008;26:1367–1372. - PubMed
MeSH terms
Substances
Grants and funding
LinkOut - more resources
Full Text Sources
Other Literature Sources