Fundamentals and Recent Developments in Approximate Bayesian Computation
- PMID: 28175922
- PMCID: PMC5837704
- DOI: 10.1093/sysbio/syw077
Fundamentals and Recent Developments in Approximate Bayesian Computation
Abstract
Bayesian inference plays an important role in phylogenetics, evolutionary biology, and in many other branches of science. It provides a principled framework for dealing with uncertainty and quantifying how it changes in the light of new evidence. For many complex models and inference problems, however, only approximate quantitative answers are obtainable. Approximate Bayesian computation (ABC) refers to a family of algorithms for approximate inference that makes a minimal set of assumptions by only requiring that sampling from a model is possible. We explain here the fundamentals of ABC, review the classical algorithms, and highlight recent developments. [ABC; approximate Bayesian computation; Bayesian inference; likelihood-free inference; phylogenetics; simulator-based models; stochastic simulation models; tree-based models.]
Figures
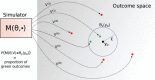
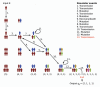
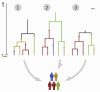
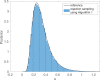
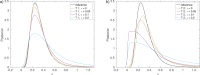
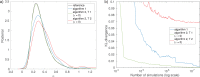
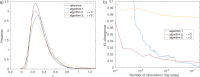
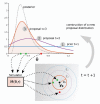
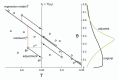
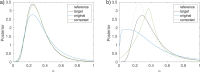
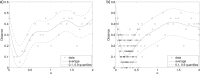
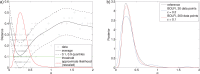
Similar articles
-
A tutorial introduction to Bayesian inference for stochastic epidemic models using Approximate Bayesian Computation.Math Biosci. 2017 May;287:42-53. doi: 10.1016/j.mbs.2016.07.001. Epub 2016 Jul 18. Math Biosci. 2017. PMID: 27444577
-
Approximate Bayesian computation (ABC) gives exact results under the assumption of model error.Stat Appl Genet Mol Biol. 2013 May 6;12(2):129-41. doi: 10.1515/sagmb-2013-0010. Stat Appl Genet Mol Biol. 2013. PMID: 23652634
-
Approximate Bayesian Computation (ABC) in practice.Trends Ecol Evol. 2010 Jul;25(7):410-8. doi: 10.1016/j.tree.2010.04.001. Epub 2010 May 18. Trends Ecol Evol. 2010. PMID: 20488578 Review.
-
AABC: approximate approximate Bayesian computation for inference in population-genetic models.Theor Popul Biol. 2015 Feb;99:31-42. doi: 10.1016/j.tpb.2014.09.002. Epub 2014 Sep 26. Theor Popul Biol. 2015. PMID: 25261426 Free PMC article.
-
On the use of kernel approximate Bayesian computation to infer population history.Genes Genet Syst. 2015;90(3):153-62. doi: 10.1266/ggs.90.153. Genes Genet Syst. 2015. PMID: 26510570 Review.
Cited by
-
Scalable Approximate Bayesian Computation for Growing Network Models via Extrapolated and Sampled Summaries.Bayesian Anal. 2022 Mar;17(1):165-192. doi: 10.1214/20-ba1248. Epub 2020 Dec 8. Bayesian Anal. 2022. PMID: 36213769 Free PMC article.
-
Designing optimal behavioral experiments using machine learning.Elife. 2024 Jan 23;13:e86224. doi: 10.7554/eLife.86224. Elife. 2024. PMID: 38261382 Free PMC article.
-
Progress on network modeling and analysis of gut microecology: a review.Appl Environ Microbiol. 2024 Mar 20;90(3):e0009224. doi: 10.1128/aem.00092-24. Epub 2024 Feb 28. Appl Environ Microbiol. 2024. PMID: 38415584 Free PMC article. Review.
-
Memory Alone Does Not Account for the Way Rats Learn a Simple Spatial Alternation Task.J Neurosci. 2020 Sep 16;40(38):7311-7317. doi: 10.1523/JNEUROSCI.0972-20.2020. Epub 2020 Aug 4. J Neurosci. 2020. PMID: 32753514 Free PMC article.
-
Pneumococcal quorum sensing drives an asymmetric owner-intruder competitive strategy during carriage via the competence regulon.Nat Microbiol. 2019 Jan;4(1):198-208. doi: 10.1038/s41564-018-0314-4. Epub 2018 Dec 10. Nat Microbiol. 2019. PMID: 30546100 Free PMC article.
References
-
- Anderson R.M., May R.M. 1992. Infectious diseases of humans: dynamics and control. Oxford University Press.
-
- Barber S., Voss J., Webster M. 2015.. The rate of convergence for approximate Bayesian computation. Electron. J. Stat. 80–105.
-
- Beaumont M.A. 2010.. Approximate Bayesian computation in evolution and ecology. Annu. Rev. Ecol. Evol. Syst. 41:379–406.
Publication types
MeSH terms
LinkOut - more resources
Full Text Sources
Other Literature Sources