Transcriptomic signatures differentiate survival from fatal outcomes in humans infected with Ebola virus
- PMID: 28100256
- PMCID: PMC5244546
- DOI: 10.1186/s13059-016-1137-3
Transcriptomic signatures differentiate survival from fatal outcomes in humans infected with Ebola virus
Abstract
Background: In 2014, Western Africa experienced an unanticipated explosion of Ebola virus infections. What distinguishes fatal from non-fatal outcomes remains largely unknown, yet is key to optimising personalised treatment strategies. We used transcriptome data for peripheral blood taken from infected and convalescent recovering patients to identify early stage host factors that are associated with acute illness and those that differentiate patient survival from fatality.
Results: The data demonstrate that individuals who succumbed to the disease show stronger upregulation of interferon signalling and acute phase responses compared to survivors during the acute phase of infection. Particularly notable is the strong upregulation of albumin and fibrinogen genes, which suggest significant liver pathology. Cell subtype prediction using messenger RNA expression patterns indicated that NK-cell populations increase in patients who survive infection. By selecting genes whose expression properties discriminated between fatal cases and survivors, we identify a small panel of responding genes that act as strong predictors of patient outcome, independent of viral load.
Conclusions: Transcriptomic analysis of the host response to pathogen infection using blood samples taken during an outbreak situation can provide multiple levels of information on both disease state and mechanisms of pathogenesis. Host biomarkers were identified that provide high predictive value under conditions where other predictors, such as viral load, are poor prognostic indicators. The data suggested that rapid analysis of the host response to infection in an outbreak situation can provide valuable information to guide an understanding of disease outcome and mechanisms of disease.
Figures
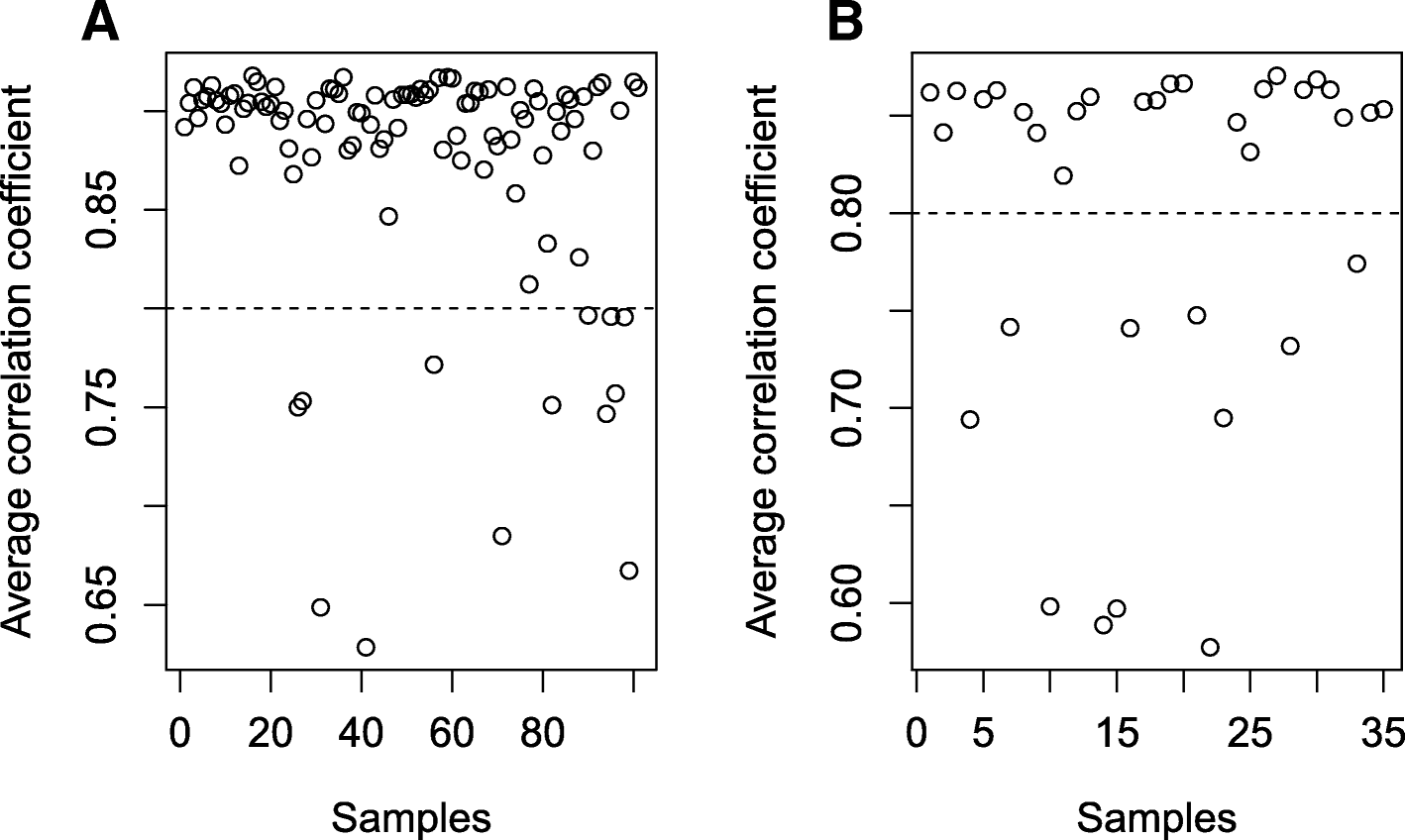
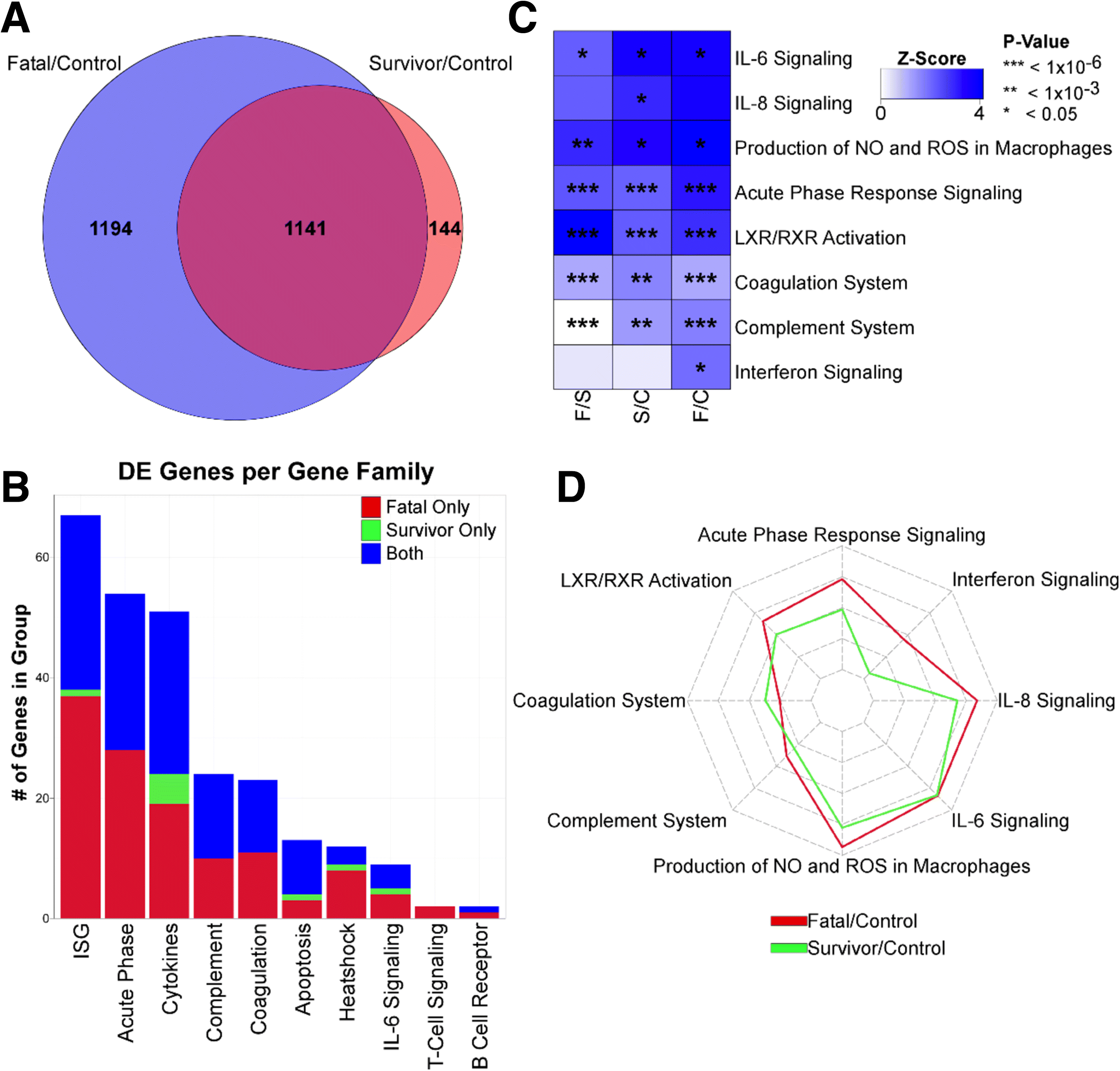
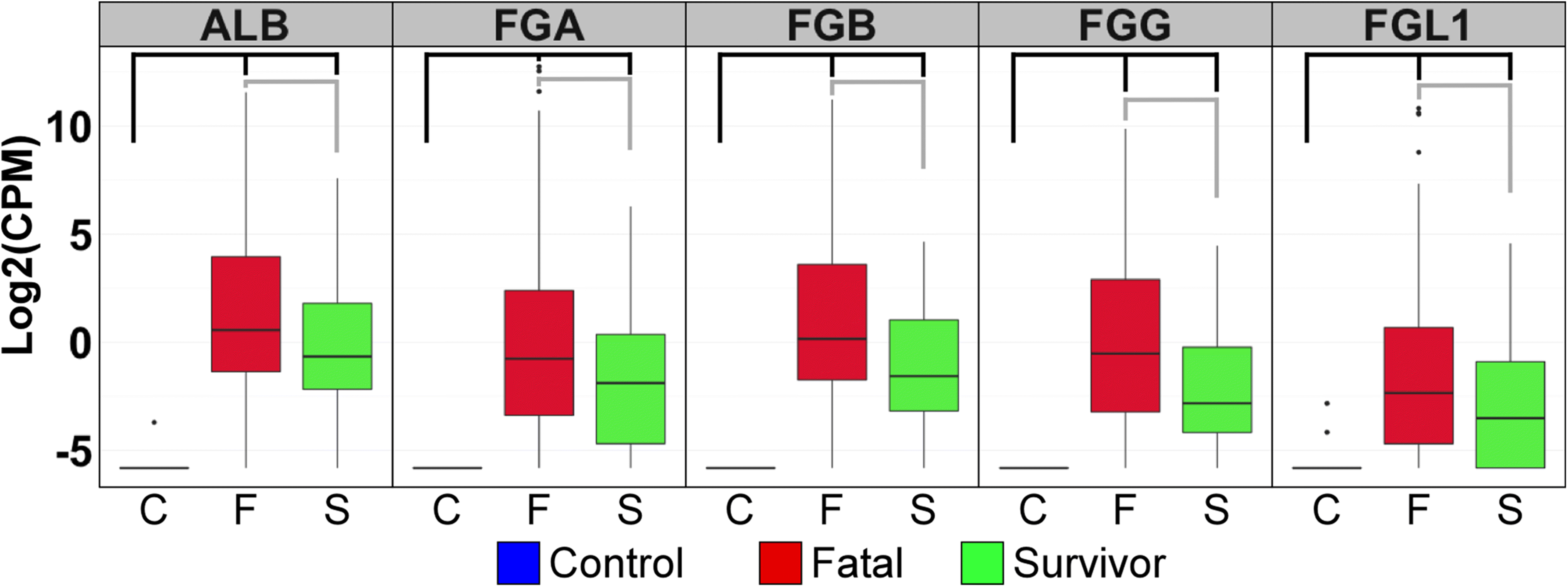
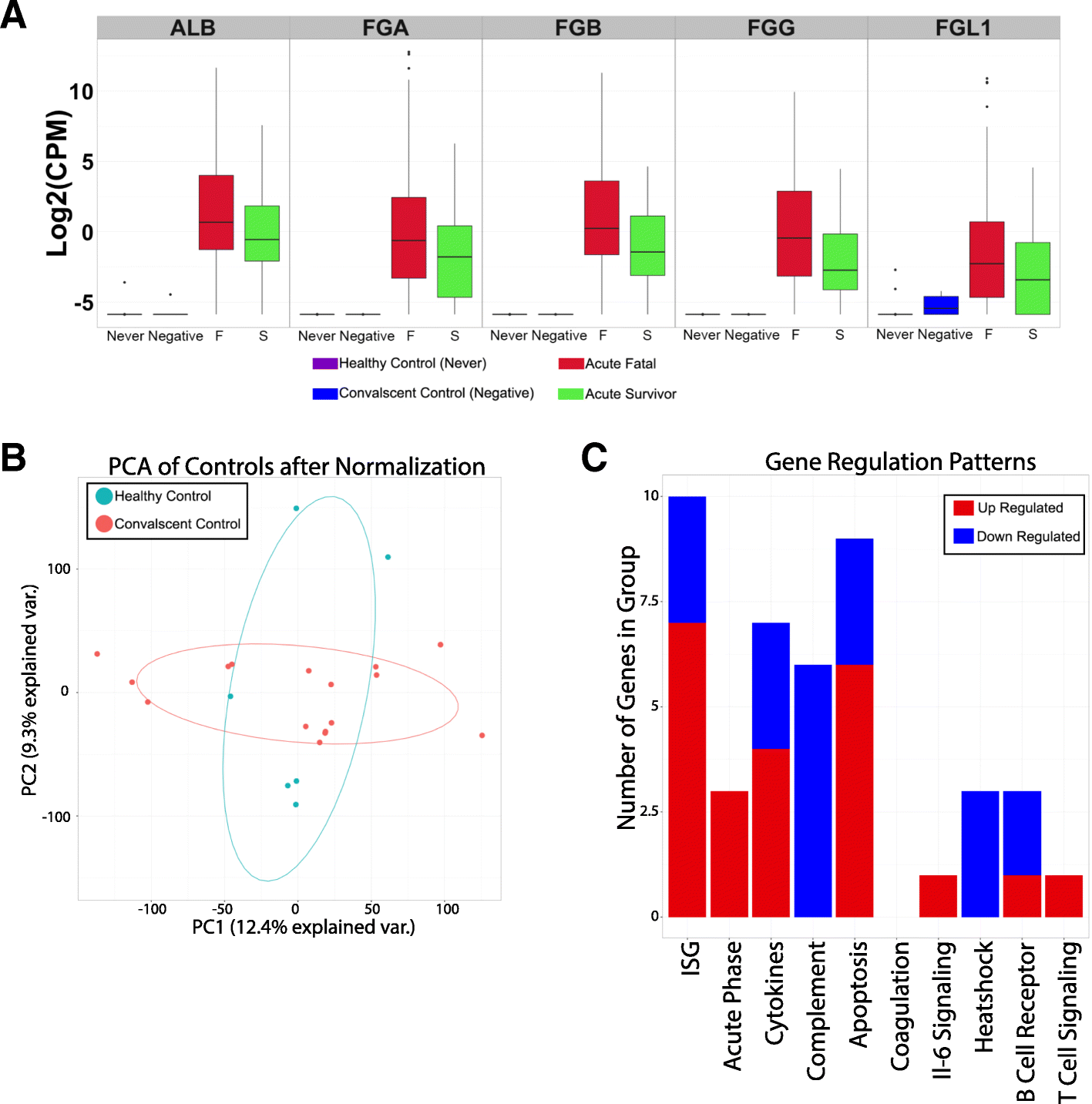
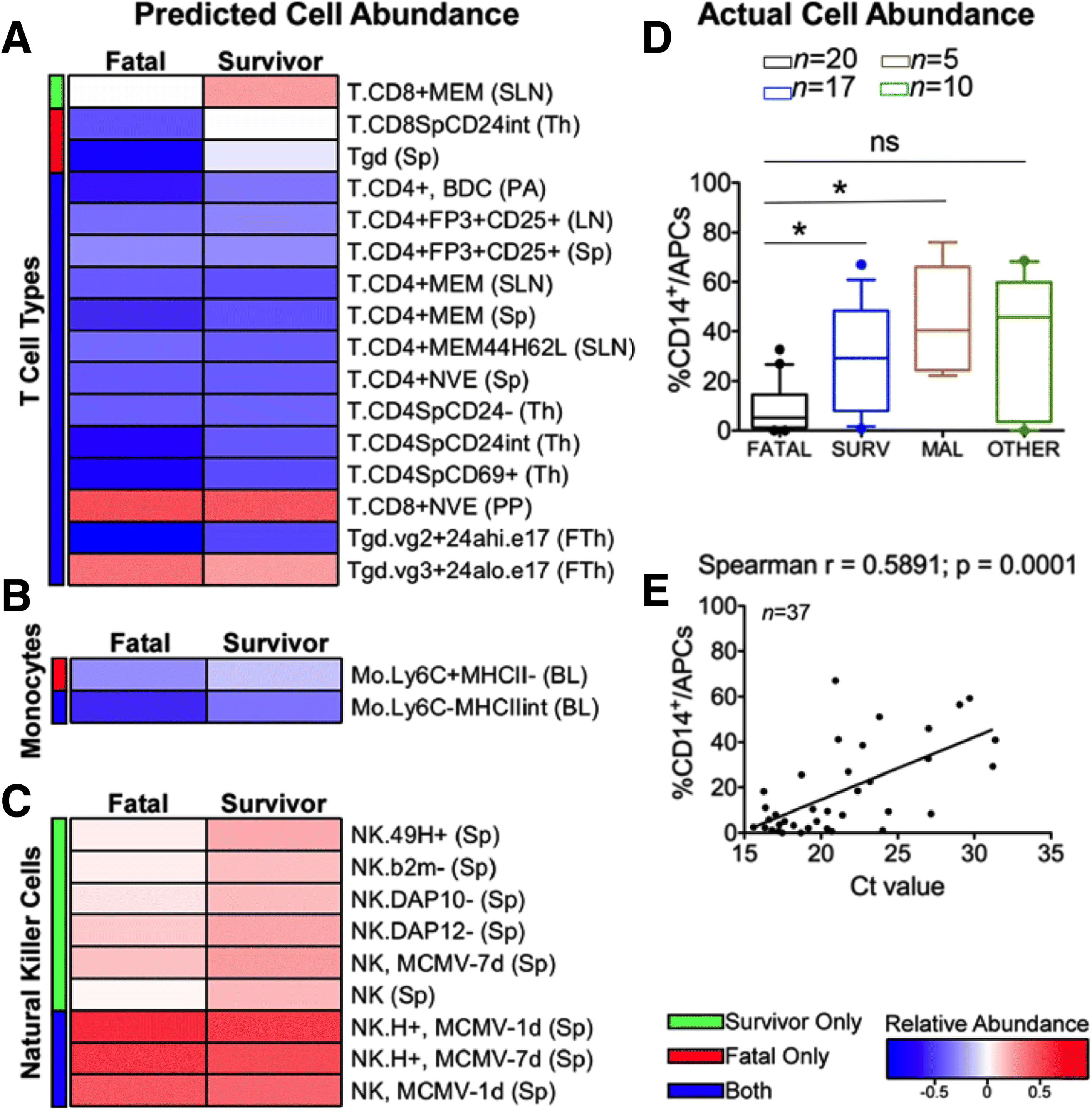
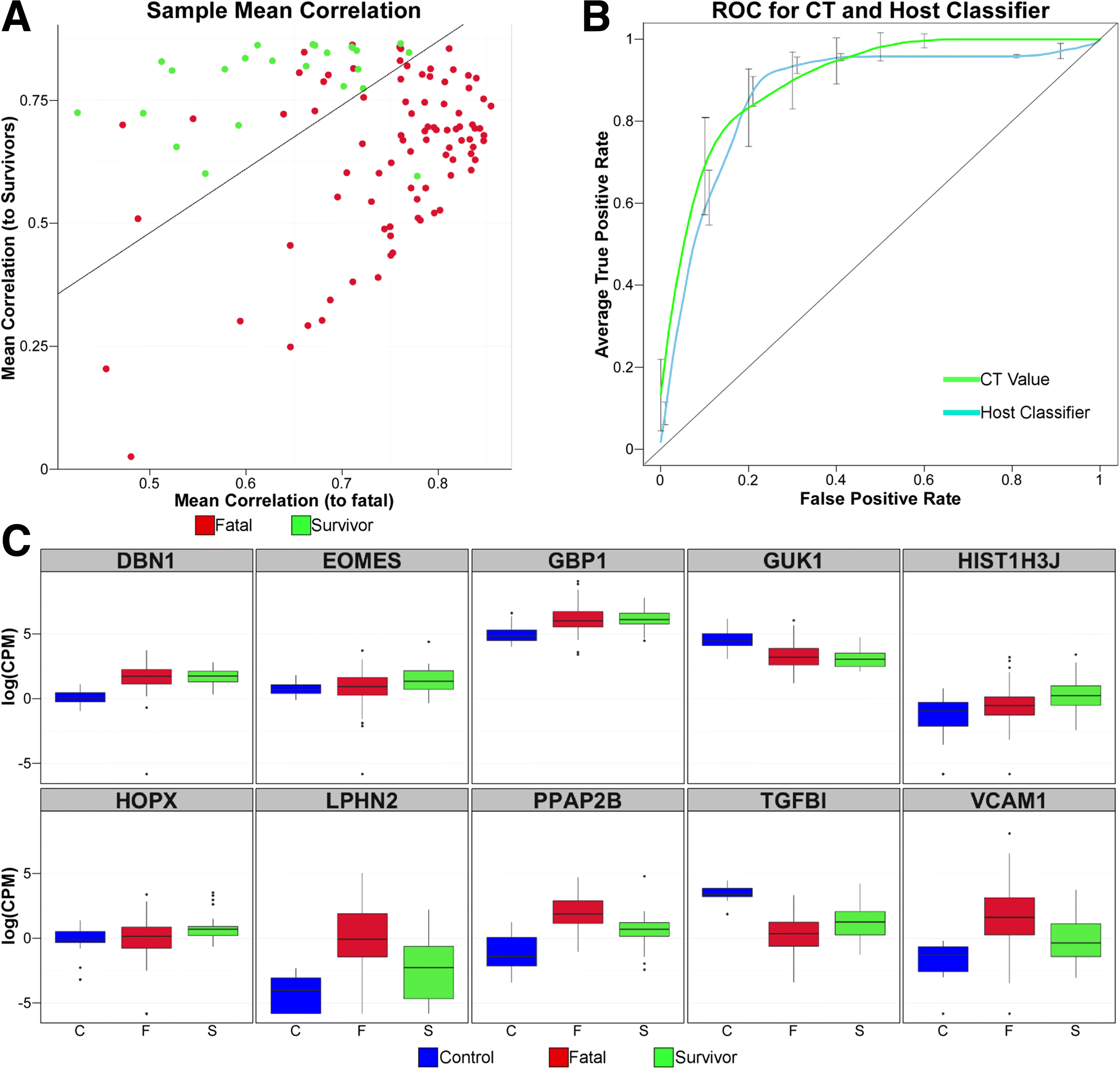
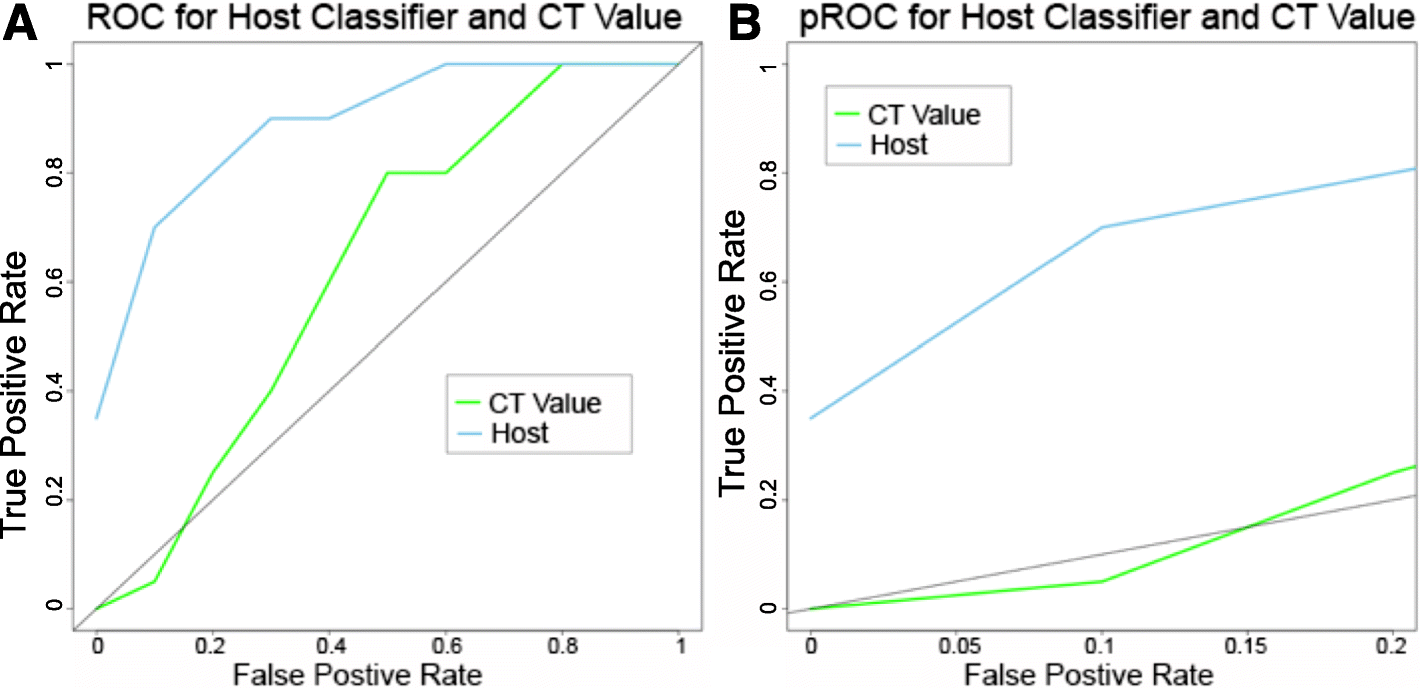
Similar articles
-
Different features of Vδ2 T and NK cells in fatal and non-fatal human Ebola infections.PLoS Negl Trop Dis. 2017 May 30;11(5):e0005645. doi: 10.1371/journal.pntd.0005645. eCollection 2017 May. PLoS Negl Trop Dis. 2017. PMID: 28558022 Free PMC article.
-
Infection with the Makona variant results in a delayed and distinct host immune response compared to previous Ebola virus variants.Sci Rep. 2017 Aug 29;7(1):9730. doi: 10.1038/s41598-017-09963-y. Sci Rep. 2017. PMID: 28852031 Free PMC article.
-
Single-Cell Profiling of Ebola Virus Disease In Vivo Reveals Viral and Host Dynamics.Cell. 2020 Nov 25;183(5):1383-1401.e19. doi: 10.1016/j.cell.2020.10.002. Epub 2020 Nov 6. Cell. 2020. PMID: 33159858 Free PMC article.
-
Host Factors Involved in Ebola Virus Replication.Curr Top Microbiol Immunol. 2018;419:113-150. doi: 10.1007/82_2017_27. Curr Top Microbiol Immunol. 2018. PMID: 28710692 Review.
-
Host Factors in Ebola Infection.Annu Rev Genomics Hum Genet. 2016 Aug 31;17:333-51. doi: 10.1146/annurev-genom-083115-022446. Epub 2016 May 4. Annu Rev Genomics Hum Genet. 2016. PMID: 27147086 Review.
Cited by
-
A blood-based host gene expression assay for early detection of respiratory viral infection: an index-cluster prospective cohort study.Lancet Infect Dis. 2021 Mar;21(3):396-404. doi: 10.1016/S1473-3099(20)30486-2. Epub 2020 Sep 24. Lancet Infect Dis. 2021. PMID: 32979932 Free PMC article.
-
Gene expression analysis identifies hub genes and pathways distinguishing fatal from survivor outcomes of Ebola virus disease.FASEB Bioadv. 2024 Jul 29;6(9):298-310. doi: 10.1096/fba.2024-00055. eCollection 2024 Sep. FASEB Bioadv. 2024. PMID: 39399477 Free PMC article.
-
Multi-cohort analysis of host immune response identifies conserved protective and detrimental modules associated with severity across viruses.Immunity. 2021 Apr 13;54(4):753-768.e5. doi: 10.1016/j.immuni.2021.03.002. Epub 2021 Mar 24. Immunity. 2021. PMID: 33765435 Free PMC article.
-
Distinct negative-sense RNA viruses induce a common set of transcripts encoding proteins forming an extensive network.J Virol. 2024 Oct 22;98(10):e0093524. doi: 10.1128/jvi.00935-24. Epub 2024 Sep 16. J Virol. 2024. PMID: 39283124 Free PMC article.
-
Comparative Transcriptomics in Ebola Makona-Infected Ferrets, Nonhuman Primates, and Humans.J Infect Dis. 2018 Nov 22;218(suppl_5):S486-S495. doi: 10.1093/infdis/jiy455. J Infect Dis. 2018. PMID: 30476250 Free PMC article.
References
-
- Trad MA, Naughton W, Yeung A, Mazlin L, O'sullivan M, Gilroy N, Fisher DA, Stuart RL. Ebola virus disease: an update on current prevention and management strategies. J Clin Virol. 2017;86:5-13. doi:10.1016/j.jcv.2016.11.005. Epub 2016 Nov 11. - PubMed
-
- Shah T, Greig J, van der Plas LM, Achar J, Caleo G, Squire JS, et al. Inpatient signs and symptoms and factors associated with death in children aged 5 years and younger admitted to two Ebola management centres in Sierra Leone, 2014: a retrospective cohort study. Lancet Glob Health. 2016;4:e495–501. doi: 10.1016/S2214-109X(16)30097-3. - DOI - PubMed
Publication types
MeSH terms
Substances
Grants and funding
LinkOut - more resources
Full Text Sources
Other Literature Sources
Medical