cDNA hybrid capture improves transcriptome analysis on low-input and archived samples
- PMID: 24814956
- PMCID: PMC4078367
- DOI: 10.1016/j.jmoldx.2014.03.004
cDNA hybrid capture improves transcriptome analysis on low-input and archived samples
Abstract
The use of massively parallel sequencing for studying RNA expression has greatly enhanced our understanding of the transcriptome through the myriad ways these data can be characterized. In particular, clinical samples provide important insights about RNA expression in health and disease, yet these studies can be complicated by RNA degradation that results from the use of formalin as a clinical preservative and by the limited amounts of RNA often available from these precious samples. In this study we describe the combined use of RNA sequencing with an exome capture selection step to enhance the yield of on-exon sequencing read data when compared with RNA sequencing alone. In particular, the exome capture step preserves the dynamic range of expression, permitting differential comparisons and validation of expressed mutations from limited and FFPE preserved samples, while reducing the data generation requirement. We conclude that cDNA hybrid capture has the potential to significantly improve transcriptome analysis from low-yield FFPE material.
Copyright © 2014 American Society for Investigative Pathology and the Association for Molecular Pathology. Published by Elsevier Inc. All rights reserved.
Figures
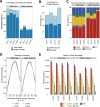
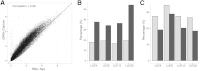
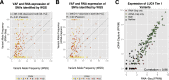
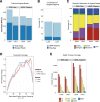

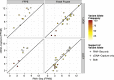
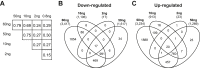
Similar articles
-
The use of exome capture RNA-seq for highly degraded RNA with application to clinical cancer sequencing.Genome Res. 2015 Sep;25(9):1372-81. doi: 10.1101/gr.189621.115. Epub 2015 Aug 7. Genome Res. 2015. PMID: 26253700 Free PMC article.
-
Performance comparison of two commercial human whole-exome capture systems on formalin-fixed paraffin-embedded lung adenocarcinoma samples.BMC Cancer. 2016 Aug 30;16(1):692. doi: 10.1186/s12885-016-2720-4. BMC Cancer. 2016. PMID: 27578032 Free PMC article.
-
Transcriptome sequencing of archived lymphoma specimens is feasible and clinically relevant using exome capture technology.Genes Chromosomes Cancer. 2022 Jan;61(1):27-36. doi: 10.1002/gcc.23002. Epub 2021 Oct 23. Genes Chromosomes Cancer. 2022. PMID: 34647650
-
Single-molecule direct RNA sequencing without cDNA synthesis.Wiley Interdiscip Rev RNA. 2011 Jul-Aug;2(4):565-70. doi: 10.1002/wrna.84. Epub 2011 Mar 14. Wiley Interdiscip Rev RNA. 2011. PMID: 21957044 Free PMC article. Review.
-
Strategies for Converting RNA to Amplifiable cDNA for Single-Cell RNA Sequencing Methods.Adv Exp Med Biol. 2019;1129:1-17. doi: 10.1007/978-981-13-6037-4_1. Adv Exp Med Biol. 2019. PMID: 30968357 Review.
Cited by
-
The use of exome capture RNA-seq for highly degraded RNA with application to clinical cancer sequencing.Genome Res. 2015 Sep;25(9):1372-81. doi: 10.1101/gr.189621.115. Epub 2015 Aug 7. Genome Res. 2015. PMID: 26253700 Free PMC article.
-
Targeted RNA sequencing enhances gene expression profiling of ultra-low input samples.RNA Biol. 2020 Dec;17(12):1741-1753. doi: 10.1080/15476286.2020.1777768. Epub 2020 Jun 28. RNA Biol. 2020. PMID: 32597303 Free PMC article.
-
RNA Sequencing of Tumor-Associated Microglia Reveals Ccl5 as a Stromal Chemokine Critical for Neurofibromatosis-1 Glioma Growth.Neoplasia. 2015 Oct;17(10):776-88. doi: 10.1016/j.neo.2015.10.002. Neoplasia. 2015. PMID: 26585233 Free PMC article.
-
Cancer immunotherapy. A dendritic cell vaccine increases the breadth and diversity of melanoma neoantigen-specific T cells.Science. 2015 May 15;348(6236):803-8. doi: 10.1126/science.aaa3828. Epub 2015 Apr 2. Science. 2015. PMID: 25837513 Free PMC article. Clinical Trial.
-
Targeted RNA Sequencing Assay to Characterize Gene Expression and Genomic Alterations.J Vis Exp. 2016 Aug 4;(114):54090. doi: 10.3791/54090. J Vis Exp. 2016. PMID: 27585245 Free PMC article.
References
-
- Prensner J.R., Iyer M.K., Balbin O.A., Dhanasekaran S.M., Cao Q., Brenner J.C., Laxman B., Asangani I.A., Grasso C.S., Kominsky H.D., Cao X., Jing X., Wang X., Siddiqui J., Wei J.T., Robinson D., Iyer H.K., Palanisamy N., Maher C.A., Chinnaiyan A.M. Transcriptome sequencing across a prostate cancer cohort identifies PCAT-1, an unannotated lincRNA implicated in disease progression. Nat Biotechnol. 2011;29:742–749. - PMC - PubMed
-
- Mortazavi A., Williams B.A., McCue K., Schaeffer L., Wold B. Mapping and quantifying mammalian transcriptomes by RNA-Seq. Nat Methods. 2008;5:621–628. - PubMed
-
- Sultan M., Schulz M.H., Richard H., Magen A., Klingenhoff A., Scherf M., Seifert M., Borodina T., Soldatov A., Parkhomchuk D., Schmidt D., O'Keeffe S., Haas S., Vingron M., Lehrach H., Yaspo M.-L. A global view of gene activity and alternative splicing by deep sequencing of the human transcriptome. Science. 2008;321:956–960. - PubMed
Publication types
MeSH terms
Substances
Grants and funding
LinkOut - more resources
Full Text Sources
Other Literature Sources
Medical