Effectively identifying eQTLs from multiple tissues by combining mixed model and meta-analytic approaches
- PMID: 23785294
- PMCID: PMC3681686
- DOI: 10.1371/journal.pgen.1003491
Effectively identifying eQTLs from multiple tissues by combining mixed model and meta-analytic approaches
Abstract
Gene expression data, in conjunction with information on genetic variants, have enabled studies to identify expression quantitative trait loci (eQTLs) or polymorphic locations in the genome that are associated with expression levels. Moreover, recent technological developments and cost decreases have further enabled studies to collect expression data in multiple tissues. One advantage of multiple tissue datasets is that studies can combine results from different tissues to identify eQTLs more accurately than examining each tissue separately. The idea of aggregating results of multiple tissues is closely related to the idea of meta-analysis which aggregates results of multiple genome-wide association studies to improve the power to detect associations. In principle, meta-analysis methods can be used to combine results from multiple tissues. However, eQTLs may have effects in only a single tissue, in all tissues, or in a subset of tissues with possibly different effect sizes. This heterogeneity in terms of effects across multiple tissues presents a key challenge to detect eQTLs. In this paper, we develop a framework that leverages two popular meta-analysis methods that address effect size heterogeneity to detect eQTLs across multiple tissues. We show by using simulations and multiple tissue data from mouse that our approach detects many eQTLs undetected by traditional eQTL methods. Additionally, our method provides an interpretation framework that accurately predicts whether an eQTL has an effect in a particular tissue.
Conflict of interest statement
The authors have declared that no competing interests exist.
Figures
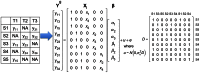




















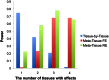
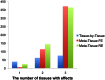
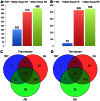


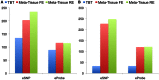


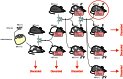
Similar articles
-
A statistical framework for joint eQTL analysis in multiple tissues.PLoS Genet. 2013 May;9(5):e1003486. doi: 10.1371/journal.pgen.1003486. Epub 2013 May 9. PLoS Genet. 2013. PMID: 23671422 Free PMC article.
-
Applying meta-analysis to genotype-tissue expression data from multiple tissues to identify eQTLs and increase the number of eGenes.Bioinformatics. 2017 Jul 15;33(14):i67-i74. doi: 10.1093/bioinformatics/btx227. Bioinformatics. 2017. PMID: 28881962 Free PMC article.
-
Influence of tissue context on gene prioritization for predicted transcriptome-wide association studies.Pac Symp Biocomput. 2019;24:296-307. Pac Symp Biocomput. 2019. PMID: 30864331 Free PMC article. Clinical Trial.
-
Exploiting expression patterns across multiple tissues to map expression quantitative trait loci.BMC Bioinformatics. 2016 Jun 24;17:257. doi: 10.1186/s12859-016-1123-5. BMC Bioinformatics. 2016. PMID: 27341818 Free PMC article.
-
Genome-Wide Expression Quantitative Trait Loci Analysis Using Mixed Models.Front Genet. 2018 Aug 21;9:341. doi: 10.3389/fgene.2018.00341. eCollection 2018. Front Genet. 2018. PMID: 30186313 Free PMC article. Review.
Cited by
-
sn-spMF: matrix factorization informs tissue-specific genetic regulation of gene expression.Genome Biol. 2020 Sep 11;21(1):235. doi: 10.1186/s13059-020-02129-6. Genome Biol. 2020. PMID: 32912314 Free PMC article.
-
Leveraging a surrogate outcome to improve inference on a partially missing target outcome.Biometrics. 2023 Jun;79(2):1472-1484. doi: 10.1111/biom.13629. Epub 2022 Mar 22. Biometrics. 2023. PMID: 35218565 Free PMC article.
-
Tissue specificity-aware TWAS (TSA-TWAS) framework identifies novel associations with metabolic, immunologic, and virologic traits in HIV-positive adults.PLoS Genet. 2021 Apr 26;17(4):e1009464. doi: 10.1371/journal.pgen.1009464. eCollection 2021 Apr. PLoS Genet. 2021. PMID: 33901188 Free PMC article.
-
Identification of expression quantitative trait loci associated with schizophrenia and affective disorders in normal brain tissue.PLoS Genet. 2018 Aug 24;14(8):e1007607. doi: 10.1371/journal.pgen.1007607. eCollection 2018 Aug. PLoS Genet. 2018. PMID: 30142156 Free PMC article.
-
Finding associated variants in genome-wide association studies on multiple traits.Bioinformatics. 2018 Jul 1;34(13):i467-i474. doi: 10.1093/bioinformatics/bty249. Bioinformatics. 2018. PMID: 29949991 Free PMC article.
References
-
- Brem RB, Yvert G, Clinton R, Kruglyak L (2002) Genetic dissection of transcriptional regulation in budding yeast. Science 296: 752–5. - PubMed
-
- Chesler EJ, Lu L, Shou S, Qu Y, Gu J, et al. (2005) Complex trait analysis of gene expression uncovers polygenic and pleiotropic networks that modulate nervous system function. Nat Genet 37: 233–42. - PubMed
-
- Bystrykh L, Weersing E, Dontje B, Sutton S, Pletcher MT, et al. (2005) Uncovering regulatory pathways that affect hematopoietic stem cell function using ‘genetical genomics’. Nat Genet 37: 225–32. - PubMed
Publication types
MeSH terms
Grants and funding
LinkOut - more resources
Full Text Sources
Other Literature Sources