Technical and biological variance structure in mRNA-Seq data: life in the real world
- PMID: 22769017
- PMCID: PMC3505161
- DOI: 10.1186/1471-2164-13-304
Technical and biological variance structure in mRNA-Seq data: life in the real world
Abstract
Background: mRNA expression data from next generation sequencing platforms is obtained in the form of counts per gene or exon. Counts have classically been assumed to follow a Poisson distribution in which the variance is equal to the mean. The Negative Binomial distribution which allows for over-dispersion, i.e., for the variance to be greater than the mean, is commonly used to model count data as well.
Results: In mRNA-Seq data from 25 subjects, we found technical variation to generally follow a Poisson distribution as has been reported previously and biological variability was over-dispersed relative to the Poisson model. The mean-variance relationship across all genes was quadratic, in keeping with a Negative Binomial (NB) distribution. Over-dispersed Poisson and NB distributional assumptions demonstrated marked improvements in goodness-of-fit (GOF) over the standard Poisson model assumptions, but with evidence of over-fitting in some genes. Modeling of experimental effects improved GOF for high variance genes but increased the over-fitting problem.
Conclusions: These conclusions will guide development of analytical strategies for accurate modeling of variance structure in these data and sample size determination which in turn will aid in the identification of true biological signals that inform our understanding of biological systems.
Figures
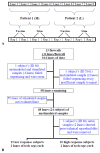
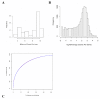
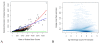
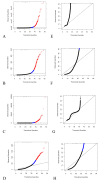
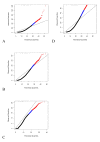
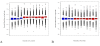
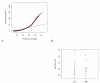
Similar articles
-
NBLDA: negative binomial linear discriminant analysis for RNA-Seq data.BMC Bioinformatics. 2016 Sep 13;17(1):369. doi: 10.1186/s12859-016-1208-1. BMC Bioinformatics. 2016. PMID: 27623864 Free PMC article.
-
Analyzing hospitalization data: potential limitations of Poisson regression.Nephrol Dial Transplant. 2015 Aug;30(8):1244-9. doi: 10.1093/ndt/gfv071. Epub 2015 Mar 25. Nephrol Dial Transplant. 2015. PMID: 25813274
-
Quasi-Poisson vs. negative binomial regression: how should we model overdispersed count data?Ecology. 2007 Nov;88(11):2766-72. doi: 10.1890/07-0043.1. Ecology. 2007. PMID: 18051645
-
On the use of zero-inflated and hurdle models for modeling vaccine adverse event count data.J Biopharm Stat. 2006;16(4):463-81. doi: 10.1080/10543400600719384. J Biopharm Stat. 2006. PMID: 16892908 Clinical Trial.
-
Understanding poisson regression.J Nurs Educ. 2014 Apr;53(4):207-15. doi: 10.3928/01484834-20140325-04. Epub 2014 Mar 25. J Nurs Educ. 2014. PMID: 24654593 Review.
Cited by
-
Transcriptomic profiles of high and low antibody responders to smallpox vaccine.Genes Immun. 2013 Jul-Aug;14(5):277-85. doi: 10.1038/gene.2013.14. Epub 2013 Apr 18. Genes Immun. 2013. PMID: 23594957 Free PMC article.
-
FineSplice, enhanced splice junction detection and quantification: a novel pipeline based on the assessment of diverse RNA-Seq alignment solutions.Nucleic Acids Res. 2014 Apr;42(8):e71. doi: 10.1093/nar/gku166. Epub 2014 Feb 25. Nucleic Acids Res. 2014. PMID: 24574529 Free PMC article.
-
Whole Transcriptome Profiling Identifies CD93 and Other Plasma Cell Survival Factor Genes Associated with Measles-Specific Antibody Response after Vaccination.PLoS One. 2016 Aug 16;11(8):e0160970. doi: 10.1371/journal.pone.0160970. eCollection 2016. PLoS One. 2016. PMID: 27529750 Free PMC article.
-
Toward reliable biomarker signatures in the age of liquid biopsies - how to standardize the small RNA-Seq workflow.Nucleic Acids Res. 2016 Jul 27;44(13):5995-6018. doi: 10.1093/nar/gkw545. Epub 2016 Jun 17. Nucleic Acids Res. 2016. PMID: 27317696 Free PMC article.
-
Calculating sample size estimates for RNA sequencing data.J Comput Biol. 2013 Dec;20(12):970-8. doi: 10.1089/cmb.2012.0283. Epub 2013 Aug 20. J Comput Biol. 2013. PMID: 23961961 Free PMC article.
References
-
- Asmann YW, Klee EW, Thompson EA, Perez EA, Middha S, Oberg AL, Therneau TM, Smith DI, Poland GA, Wieben ED. et al.3′ tag digital gene expression profiling of human brain and universal reference RNA using Illumina Genome Analyzer. BMC Genomics. 2009;10:531. doi: 10.1186/1471-2164-10-531. - DOI - PMC - PubMed
-
- Lee A, Hansen KD, Bullard J, Dudoit S, Sherlock G. Novel low abundance and transient RNAs in yeast revealed by tiling microarrays and ultra high-throughput sequencing are not conserved across closely related yeast species. PLoS genetics. 2008;4(12):e1000299. doi: 10.1371/journal.pgen.1000299. - DOI - PMC - PubMed
Publication types
MeSH terms
Substances
Grants and funding
LinkOut - more resources
Full Text Sources
Molecular Biology Databases