Effects of heterogeneous and clustered contact patterns on infectious disease dynamics
- PMID: 21673864
- PMCID: PMC3107246
- DOI: 10.1371/journal.pcbi.1002042
Effects of heterogeneous and clustered contact patterns on infectious disease dynamics
Erratum in
- PLoS Comput Biol. 2011 Jul;7(7). doi: 10.1371/annotation/85b99614-44b4-4052-9195-a77d52dbdc05
Abstract
The spread of infectious diseases fundamentally depends on the pattern of contacts between individuals. Although studies of contact networks have shown that heterogeneity in the number of contacts and the duration of contacts can have far-reaching epidemiological consequences, models often assume that contacts are chosen at random and thereby ignore the sociological, temporal and/or spatial clustering of contacts. Here we investigate the simultaneous effects of heterogeneous and clustered contact patterns on epidemic dynamics. To model population structure, we generalize the configuration model which has a tunable degree distribution (number of contacts per node) and level of clustering (number of three cliques). To model epidemic dynamics for this class of random graph, we derive a tractable, low-dimensional system of ordinary differential equations that accounts for the effects of network structure on the course of the epidemic. We find that the interaction between clustering and the degree distribution is complex. Clustering always slows an epidemic, but simultaneously increasing clustering and the variance of the degree distribution can increase final epidemic size. We also show that bond percolation-based approximations can be highly biased if one incorrectly assumes that infectious periods are homogeneous, and the magnitude of this bias increases with the amount of clustering in the network. We apply this approach to model the high clustering of contacts within households, using contact parameters estimated from survey data of social interactions, and we identify conditions under which network models that do not account for household structure will be biased.
Conflict of interest statement
The authors have declared that no competing interests exist.
Figures
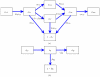







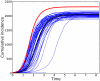




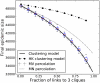


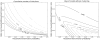
Similar articles
-
Household members do not contact each other at random: implications for infectious disease modelling.Proc Biol Sci. 2018 Dec 19;285(1893):20182201. doi: 10.1098/rspb.2018.2201. Proc Biol Sci. 2018. PMID: 30963910 Free PMC article.
-
Using network properties to predict disease dynamics on human contact networks.Proc Biol Sci. 2011 Dec 7;278(1724):3544-50. doi: 10.1098/rspb.2011.0290. Epub 2011 Apr 27. Proc Biol Sci. 2011. PMID: 21525056 Free PMC article.
-
Models of epidemics: when contact repetition and clustering should be included.Theor Biol Med Model. 2009 Jun 29;6:11. doi: 10.1186/1742-4682-6-11. Theor Biol Med Model. 2009. PMID: 19563624 Free PMC article.
-
Epidemic thresholds in dynamic contact networks.J R Soc Interface. 2009 Mar 6;6(32):233-41. doi: 10.1098/rsif.2008.0218. J R Soc Interface. 2009. PMID: 18664429 Free PMC article.
-
The dynamic nature of contact networks in infectious disease epidemiology.J Biol Dyn. 2010 Sep;4(5):478-89. doi: 10.1080/17513758.2010.503376. J Biol Dyn. 2010. PMID: 22877143 Review.
Cited by
-
Cocirculation of infectious diseases on networks.Phys Rev E Stat Nonlin Soft Matter Phys. 2013 Jun;87(6):060801. doi: 10.1103/PhysRevE.87.060801. Epub 2013 Jun 20. Phys Rev E Stat Nonlin Soft Matter Phys. 2013. PMID: 23848616 Free PMC article.
-
Individual risk perception and empirical social structures shape the dynamics of infectious disease outbreaks.PLoS Comput Biol. 2022 Feb 16;18(2):e1009760. doi: 10.1371/journal.pcbi.1009760. eCollection 2022 Feb. PLoS Comput Biol. 2022. PMID: 35171901 Free PMC article.
-
Multiple peaks patterns of epidemic spreading in multi-layer networks.Chaos Solitons Fractals. 2018 Feb;107:135-142. doi: 10.1016/j.chaos.2017.12.026. Epub 2018 Jan 3. Chaos Solitons Fractals. 2018. PMID: 32288351 Free PMC article.
-
Spatial analyses of wildlife contact networks.J R Soc Interface. 2015 Jan 6;12(102):20141004. doi: 10.1098/rsif.2014.1004. J R Soc Interface. 2015. PMID: 25411407 Free PMC article.
-
Modelling the impact of human behavior using a two-layer Watts-Strogatz network for transmission and control of Mpox.BMC Infect Dis. 2024 Mar 26;24(1):351. doi: 10.1186/s12879-024-09239-7. BMC Infect Dis. 2024. PMID: 38532346 Free PMC article.
References
Publication types
MeSH terms
Grants and funding
LinkOut - more resources
Full Text Sources