How to understand the cell by breaking it: network analysis of gene perturbation screens
- PMID: 20195495
- PMCID: PMC2829042
- DOI: 10.1371/journal.pcbi.1000655
How to understand the cell by breaking it: network analysis of gene perturbation screens
Conflict of interest statement
The author has declared that no competing interests exist.
Figures
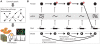
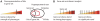
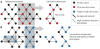
Similar articles
-
Introduction: Cancer Gene Networks.Methods Mol Biol. 2017;1513:1-9. doi: 10.1007/978-1-4939-6539-7_1. Methods Mol Biol. 2017. PMID: 27807826
-
Mining and state-space modeling and verification of sub-networks from large-scale biomolecular networks.BMC Bioinformatics. 2007 Aug 31;8:324. doi: 10.1186/1471-2105-8-324. BMC Bioinformatics. 2007. PMID: 17764552 Free PMC article.
-
KCML: a machine-learning framework for inference of multi-scale gene functions from genetic perturbation screens.Mol Syst Biol. 2020 Mar;16(3):e9083. doi: 10.15252/msb.20199083. Mol Syst Biol. 2020. PMID: 32141232 Free PMC article.
-
Complex networks and simple models in biology.J R Soc Interface. 2005 Dec 22;2(5):419-30. doi: 10.1098/rsif.2005.0067. J R Soc Interface. 2005. PMID: 16849202 Free PMC article. Review.
-
From molecules to cellular networks: past and future outlook.Phys Biol. 2017 Feb 16;14(1):013003. doi: 10.1088/1478-3975/aa5b6a. Phys Biol. 2017. PMID: 28140350 Review.
Cited by
-
From pixels to phenotypes: Integrating image-based profiling with cell health data as BioMorph features improves interpretability.Mol Biol Cell. 2024 Mar 1;35(3):mr2. doi: 10.1091/mbc.E23-08-0298. Epub 2024 Jan 3. Mol Biol Cell. 2024. PMID: 38170589 Free PMC article.
-
Plato's cave algorithm: inferring functional signaling networks from early gene expression shadows.PLoS Comput Biol. 2010 Jun 24;6(6):e1000828. doi: 10.1371/journal.pcbi.1000828. PLoS Comput Biol. 2010. PMID: 20585619 Free PMC article.
-
Incorporating interaction networks into the determination of functionally related hit genes in genomic experiments with Markov random fields.Bioinformatics. 2017 Jul 15;33(14):i170-i179. doi: 10.1093/bioinformatics/btx244. Bioinformatics. 2017. PMID: 28881978 Free PMC article.
-
Shrinkage estimation of dispersion in Negative Binomial models for RNA-seq experiments with small sample size.Bioinformatics. 2013 May 15;29(10):1275-82. doi: 10.1093/bioinformatics/btt143. Epub 2013 Apr 14. Bioinformatics. 2013. PMID: 23589650 Free PMC article.
-
Identification of highly connected and differentially expressed gene subnetworks in metastasizing endometrial cancer.PLoS One. 2018 Nov 1;13(11):e0206665. doi: 10.1371/journal.pone.0206665. eCollection 2018. PLoS One. 2018. PMID: 30383835 Free PMC article.
References
-
- Winzeler EA, Shoemaker DD, Astromoff A, Liang H, Anderson K, et al. Functional characterization of the S. cerevisiae genome by gene deletion and parallel analysis. Science. 1999;285:901–906. - PubMed
-
- Fuchs F, Boutros M. Cellular phenotyping by RNAi. Brief Funct Genomic Proteomic. 2006;5:52–56. - PubMed
-
- Moffat J, Sabatini D. Building mammalian signalling pathways with RNAi screens. Nat Rev Mol Cell Biol. 2006;7:177–187. - PubMed
-
- Boutros M, Ahringer J. The art and design of genetic screens: RNA interference. Nat Rev Genet. 2008;9:554–566. - PubMed
MeSH terms
LinkOut - more resources
Full Text Sources
Other Literature Sources