forked from akanazawa/hmr
-
Notifications
You must be signed in to change notification settings - Fork 52
Commit
This commit does not belong to any branch on this repository, and may belong to a fork outside of the repository.
- Loading branch information
Showing
25 changed files
with
3,420 additions
and
48 deletions.
There are no files selected for viewing
This file contains bidirectional Unicode text that may be interpreted or compiled differently than what appears below. To review, open the file in an editor that reveals hidden Unicode characters.
Learn more about bidirectional Unicode characters
This file contains bidirectional Unicode text that may be interpreted or compiled differently than what appears below. To review, open the file in an editor that reveals hidden Unicode characters.
Learn more about bidirectional Unicode characters
Original file line number | Diff line number | Diff line change |
---|---|---|
@@ -0,0 +1,13 @@ | ||
# TODO: Replace with where you downloaded your resnet_v2_50. | ||
PRETRAINED=/scratch1/projects/tf_datasets/models/resnet_v2_50/resnet_v2_50.ckpt | ||
# TODO: Replace with where you generated tf_record! | ||
DATA_DIR=/scratch1/storage/hmr_release_files/test_tf_datasets/ | ||
|
||
CMD="python -m src.main --d_lr 1e-4 --e_lr 1e-5 --log_img_step 1000 --pretrained_model_path=${PRETRAINED} --data_dir ${DATA_DIR} --e_loss_weight 60. --batch_size=64 --use_3d_label True --e_3d_weight 60. --datasets lsp,lsp_ext,mpii,h36m,coco,mpi_inf_3dhp --epoch 75 --log_dir logs" | ||
|
||
# To pick up training/training from a previous model, set LP | ||
# LP='logs/<WITH_YOUR_TRAINED_MODEL>' | ||
# CMD="python -m src.main --d_lr 1e-4 --e_lr 1e-5 --log_img_step 1000 --load_path=${LP} --e_loss_weight 60. --batch_size=64 --use_3d_label True --e_3d_weight 60. --datasets lsp lsp_ext mpii h36m coco mpi_inf_3dhp --epoch 75" | ||
|
||
echo $CMD | ||
$CMD |
This file contains bidirectional Unicode text that may be interpreted or compiled differently than what appears below. To review, open the file in an editor that reveals hidden Unicode characters.
Learn more about bidirectional Unicode characters
Original file line number | Diff line number | Diff line change |
---|---|---|
@@ -0,0 +1,109 @@ | ||
## Pre-reqs | ||
|
||
### Download required models | ||
|
||
1. Download the mean SMPL parameters (initialization) | ||
``` | ||
wget https://people.eecs.berkeley.edu/~kanazawa/cachedir/hmr/neutral_smpl_mean_params.h5 | ||
``` | ||
|
||
Store this inside `hmr/models/`, along with the neutral SMPL model | ||
(`neutral_smpl_with_cocoplus_reg.pkl`). | ||
|
||
|
||
2. Download the pre-trained resnet-50 from | ||
[Tensorflow](https://github.com/tensorflow/models/tree/master/research/slim#Pretrained) | ||
``` | ||
wget http://download.tensorflow.org/models/resnet_v2_50_2017_04_14.tar.gz && tar -xf resnet_v2_50_2017_04_14.tar.gz | ||
``` | ||
|
||
3. In `src/do_train.sh`, replace the path of `PRETRAINED` to the path of this model (`resnet_v2_50.ckpt`). | ||
|
||
### Download datasets. | ||
Download these datasets somewhere. | ||
|
||
- [LSP](http://sam.johnson.io/research/lsp_dataset.zip) and [LSP extended](http://sam.johnson.io/research/lspet_dataset.zip) | ||
- [COCO](http://cocodataset.org/#download) we used 2014 Train. You also need to | ||
install the [COCO API](https://github.com/cocodataset/cocoapi) for python. | ||
- [MPII](http://human-pose.mpi-inf.mpg.de/#download) | ||
- [MPI-INF-3DHP](http://human-pose.mpi-inf.mpg.de/#download) | ||
|
||
For Human3.6M, download the pre-computed tfrecords [here](https://drive.google.com/file/d/14RlfDlREouBCNsR1QGDP0qpOUIu5LlV5/view?usp=sharing). | ||
Note that this is 9.1GB! I advice you do this in a directly outside of the HMR code base. | ||
``` | ||
wget https://angjookanazawa.com/cachedir/hmr/tf_records_human36m.tar.gz | ||
``` | ||
|
||
If you use these datasets, please consider citing them. | ||
|
||
## Mosh Data. | ||
We provide the MoShed data using the neutral SMPL model. | ||
Please note that usage of this data is for [**non-comercial scientific research only**](http://mosh.is.tue.mpg.de/data_license). | ||
|
||
If you use any of the MoSh data, please cite: | ||
``` | ||
article{Loper:SIGASIA:2014, | ||
title = {{MoSh}: Motion and Shape Capture from Sparse Markers}, | ||
author = {Loper, Matthew M. and Mahmood, Naureen and Black, Michael J.}, | ||
journal = {ACM Transactions on Graphics, (Proc. SIGGRAPH Asia)}, | ||
volume = {33}, | ||
number = {6}, | ||
pages = {220:1--220:13}, | ||
publisher = {ACM}, | ||
address = {New York, NY, USA}, | ||
month = nov, | ||
year = {2014}, | ||
url = {http://doi.acm.org/10.1145/2661229.2661273}, | ||
month_numeric = {11} | ||
} | ||
``` | ||
|
||
[Download link to MoSh](https://drive.google.com/file/d/1b51RMzi_5DIHeYh2KNpgEs8LVaplZSRP/view?usp=sharing) | ||
|
||
## TFRecord Generation | ||
|
||
All the data has to be converted into TFRecords and saved to a `DATA_DIR` of | ||
your choice. | ||
|
||
1. Make `DATA_DIR` where you will save the tf_records. For ex: | ||
``` | ||
mkdir ~/hmr/tf_datasets/ | ||
``` | ||
|
||
2. Edit `prepare_datasets.sh`, with paths to where you downloaded the datasets, | ||
and set `DATA_DIR` to the path to the directory you just made. | ||
|
||
3. From the root HMR directly (where README is), run `prepare_datasets.sh`, which calls the tfrecord conversion scripts: | ||
``` | ||
sh prepare_datasets.sh | ||
``` | ||
|
||
This takes a while! If there is an issue consider running line by line. | ||
|
||
4. Move the downloaded human36m tf_records `tf_records_human36m.tar.gz` into the | ||
`data_dir`: | ||
``` | ||
tar -xf tf_records_human36m.tar.gz | ||
``` | ||
|
||
5. In `do_train.sh` and/or `src/config.py`, set `DATA_DIR` to the path where you saved the | ||
tf_records. | ||
|
||
|
||
## Training | ||
Finally we can start training! | ||
A sample training script (with parameters used in the paper) is in | ||
`do_train.sh`. | ||
|
||
Update the path to in the beginning of this script and run: | ||
``` | ||
sh do_train.sh | ||
``` | ||
|
||
The training write to a log directory that you can specify. | ||
Setup tensorboard to this directory to monitor the training progress like so: | ||
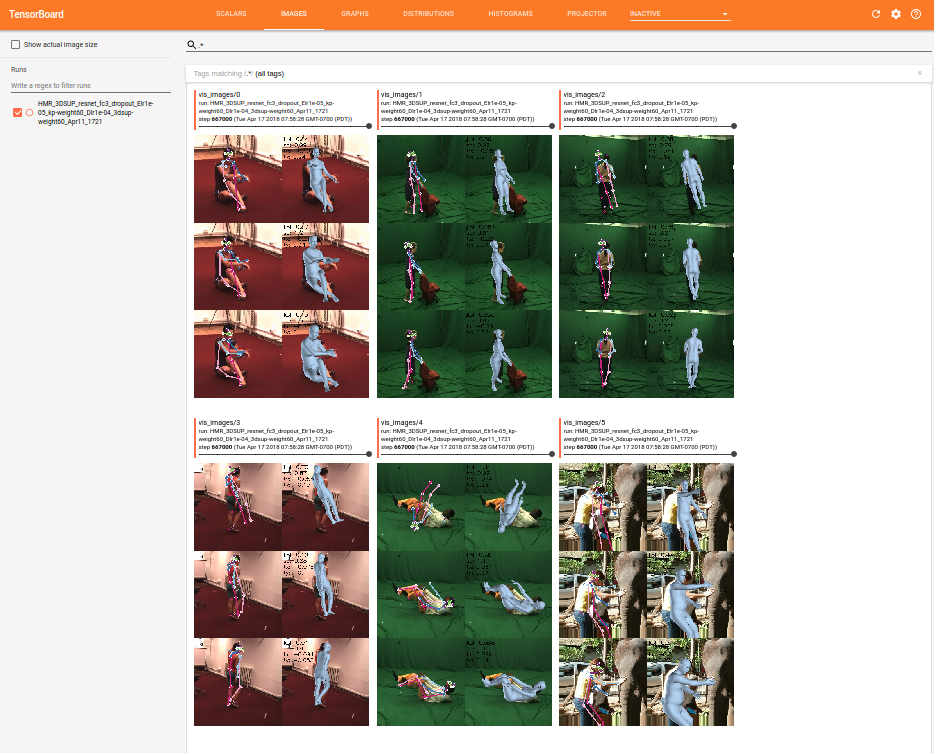 | ||
|
||
It's important to visually monitor the training! Make sure that the images | ||
loaded look right. | ||
|
This file contains bidirectional Unicode text that may be interpreted or compiled differently than what appears below. To review, open the file in an editor that reveals hidden Unicode characters.
Learn more about bidirectional Unicode characters
Original file line number | Diff line number | Diff line change |
---|---|---|
@@ -0,0 +1,55 @@ | ||
# --------------------------- | ||
# ----- SET YOUR PATH!! ----- | ||
# --------------------------- | ||
# This is where you want all of your tf_records to be saved: | ||
DATA_DIR=/scratch1/storage/hmr_release_files/test_tf_datasets | ||
|
||
# This is the directory that contains README.txt | ||
LSP_DIR=/scratch1/storage/human_datasets/lsp_dataset | ||
|
||
# This is the directory that contains README.txt | ||
LSP_EXT_DIR=/scratch1/storage/human_datasets/lsp_extended | ||
|
||
# This is the directory that contains 'images' and 'annotations' | ||
MPII_DIR=/scratch1/storage/human_datasets/mpii | ||
|
||
# This is the directory that contains README.txt | ||
COCO_DIR=/scratch1/storage/coco | ||
|
||
# This is the directory that contains README.txt, S1..S8, etc | ||
MPI_INF_3DHP_DIR=/scratch1/storage/mpi_inf_3dhp | ||
|
||
## Mosh | ||
# This is the path to the directory that contains neutrSMPL_* directories | ||
MOSH_DIR=/scratch1/storage/human_datasets/neutrMosh | ||
# --------------------------- | ||
|
||
|
||
# --------------------------- | ||
# Run each command below from this directory. I advice to run each one independently. | ||
# --------------------------- | ||
|
||
# ----- LSP ----- | ||
python -m src.datasets.lsp_to_tfrecords --img_directory $LSP_DIR --output_directory $DATA_DIR/lsp | ||
|
||
# ----- LSP-extended ----- | ||
python -m src.datasets.lsp_to_tfrecords --img_directory $LSP_EXT_DIR --output_directory $DATA_DIR/lsp_ext | ||
|
||
# ----- MPII ----- | ||
python -m src.datasets.mpii_to_tfrecords --img_directory $MPII_DIR --output_directory $DATA_DIR/mpii | ||
|
||
# ----- COCO ----- | ||
python -m src.datasets.coco_to_tfrecords --data_directory $COCO_DIR --output_directory $DATA_DIR/coco | ||
|
||
# ----- MPI-INF-3DHP ----- | ||
python -m src.datasets.mpi_inf_3dhp_to_tfrecords --data_directory $MPI_INF_3DHP_DIR --output_directory $DATA_DIR/mpi_inf_3dhp | ||
|
||
# ----- Mosh data, for each dataset ----- | ||
# CMU: | ||
python -m src.datasets.smpl_to_tfrecords --data_directory $MOSH_DIR --output_directory $DATA_DIR/mocap_neutrMosh --dataset_name 'neutrSMPL_CMU' | ||
|
||
# H3.6M: | ||
python -m src.datasets.smpl_to_tfrecords --data_directory $MOSH_DIR --output_directory $DATA_DIR/mocap_neutrMosh --dataset_name 'neutrSMPL_H3.6' | ||
|
||
# jointLim: | ||
python -m src.datasets.smpl_to_tfrecords --data_directory $MOSH_DIR --output_directory $DATA_DIR/mocap_neutrMosh --dataset_name 'neutrSMPL_jointLim' |
This file contains bidirectional Unicode text that may be interpreted or compiled differently than what appears below. To review, open the file in an editor that reveals hidden Unicode characters.
Learn more about bidirectional Unicode characters
Original file line number | Diff line number | Diff line change |
---|---|---|
@@ -1,4 +1,4 @@ | ||
# python requiremenst | ||
# python requirements | ||
pip>=9.0 | ||
scipy | ||
numpy | ||
|
This file contains bidirectional Unicode text that may be interpreted or compiled differently than what appears below. To review, open the file in an editor that reveals hidden Unicode characters.
Learn more about bidirectional Unicode characters
Oops, something went wrong.