Automated segmentation of the thyroid gland on thoracic CT scans by multiatlas label fusion and random forest classification
- PMID: 26730397
- PMCID: PMC4695653
- DOI: 10.1117/1.JMI.2.4.044006
Automated segmentation of the thyroid gland on thoracic CT scans by multiatlas label fusion and random forest classification
Abstract
The thyroid is an endocrine gland that regulates metabolism. Thyroid image analysis plays an important role in both diagnostic radiology and radiation oncology treatment planning. Low tissue contrast of the thyroid relative to surrounding anatomic structures makes manual segmentation of this organ challenging. This work proposes a fully automated system for thyroid segmentation on CT imaging. Following initial thyroid segmentation with multiatlas joint label fusion, a random forest (RF) algorithm was applied. Multiatlas label fusion transfers labels from labeled atlases and warps them to target images using deformable registration. A consensus atlas solution was formed based on optimal weighting of atlases and similarity to a given target image. Following the initial segmentation, a trained RF classifier employed voxel scanning to assign class-conditional probabilities to the voxels in the target image. Thyroid voxels were categorized with positive labels and nonthyroid voxels were categorized with negative labels. Our method was evaluated on CT scans from 66 patients, 6 of which served as atlases for multiatlas label fusion. The system with independent multiatlas label fusion method and RF classifier achieved average dice similarity coefficients of [Formula: see text] and [Formula: see text], respectively. The system with sequential multiatlas label fusion followed by RF correction increased the dice similarity coefficient to [Formula: see text] and improved the segmentation accuracy.
Keywords: multiatlas label fusion; random forest; thyroid segmentation.
Figures
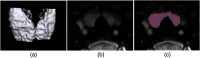
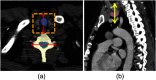
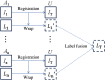
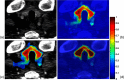
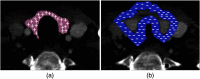
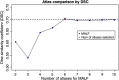
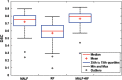
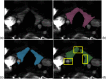
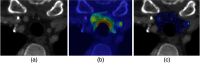
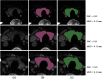
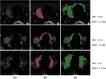
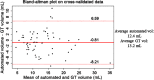
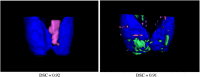
Similar articles
-
Multiatlas whole heart segmentation of CT data using conditional entropy for atlas ranking and selection.Med Phys. 2015 Jul;42(7):3822-33. doi: 10.1118/1.4921366. Med Phys. 2015. PMID: 26133584
-
Dynamic multiatlas selection-based consensus segmentation of head and neck structures from CT images.Med Phys. 2019 Dec;46(12):5612-5622. doi: 10.1002/mp.13854. Epub 2019 Oct 31. Med Phys. 2019. PMID: 31587300 Free PMC article.
-
Enhancing atlas based segmentation with multiclass linear classifiers.Med Phys. 2015 Dec;42(12):7169-81. doi: 10.1118/1.4935946. Med Phys. 2015. PMID: 26632071
-
Multi-atlas label fusion using hybrid of discriminative and generative classifiers for segmentation of cardiac MR images.Annu Int Conf IEEE Eng Med Biol Soc. 2015 Aug;2015:2977-80. doi: 10.1109/EMBC.2015.7319017. Annu Int Conf IEEE Eng Med Biol Soc. 2015. PMID: 26736917
-
Local manifold learning for multiatlas segmentation: application to hippocampal segmentation in healthy population and Alzheimer's disease.CNS Neurosci Ther. 2015 Oct;21(10):826-36. doi: 10.1111/cns.12415. Epub 2015 Jun 30. CNS Neurosci Ther. 2015. PMID: 26122409 Free PMC article.
Cited by
-
Application of Multi-Scale Fusion Attention U-Net to Segment the Thyroid Gland on Localized Computed Tomography Images for Radiotherapy.Front Oncol. 2022 May 26;12:844052. doi: 10.3389/fonc.2022.844052. eCollection 2022. Front Oncol. 2022. PMID: 35720003 Free PMC article.
-
Signal dropout correction-based ultrasound segmentation for diastolic mitral valve modeling.J Med Imaging (Bellingham). 2018 Apr;5(2):021214. doi: 10.1117/1.JMI.5.2.021214. Epub 2018 Feb 9. J Med Imaging (Bellingham). 2018. PMID: 29487886 Free PMC article.
-
Mediastinal lymph node detection and station mapping on chest CT using spatial priors and random forest.Med Phys. 2016 Jul;43(7):4362. doi: 10.1118/1.4954009. Med Phys. 2016. PMID: 27370151 Free PMC article.
References
-
- Sethian J. A., “Level set methods and fast marching methods: evolving interfaces in computational geometry, fluid mechanics, computer vision, and materials science,” in Cambridge Monographs on Applied and Computational Mathematics, 2nd ed., p. 378, Cambridge University Press, Cambridge, UK: (1999).
-
- Boykov Y., Veksler O., Zabih R., “Fast approximate energy minimization via graph cuts,” IEEE Trans. Pattern Anal. Mach. Intell. 23, 1222–1239 (2001).10.1109/34.969114 - DOI
LinkOut - more resources
Full Text Sources